
TED日本語
TED Talks(英語 日本語字幕付き動画)
TED日本語 - ジム・サイモンズ: ウォールストリートを制した天才数学者
TED Talks
ウォールストリートを制した天才数学者
A rare interview with the mathematician who cracked Wall Street
ジム・サイモンズ
Jim Simons
内容
数学者であり暗号の専門家であるジム・サイモンズは、暗号解読に用いる高度な数学を使って、金融市場に潜むパターンを解読できるかもしれないと気付きました。1兆円を超える資産を築いた今、彼は次の世代の数学教師や研究者の育成に携わっています。TEDのクリス・アンダーソンが、サイモンズと膝を交えて数にまつわる彼の驚くべき人生について聞きます。
字幕
SCRIPT
Script
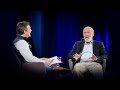
Chris Anderson: You were something of a mathematical phenom. You had already taught at Harvard and MIT at a young age. And then the NSA came calling. What was that about?
Jim Simons: Well the NSA -- that's the National Security Agency -- they didn't exactly come calling. They had an operation at Princeton, where they hired mathematicians to attack secret codes and stuff like that. And I knew that existed. And they had a very good policy, because you could do half your time at your own mathematics, and at least half your time working on their stuff. And they paid a lot. So that was an irresistible pull. So, I went there.
CA: You were a code-cracker.
JS: I was.
CA: Until you got fired.
JS: Well, I did get fired. Yes.
CA: How come?
JS: Well, how come? I got fired because, well, the Vietnam War was on, and the boss of bosses in my organization was a big fan of the war and wrote a New York Times article, a magazine section cover story, about how we would win in Vietnam. And I didn't like that war, I thought it was stupid. And I wrote a letter to the Times, which they published, saying not everyone who works for Maxwell Taylor, if anyone remembers that name, agrees with his views. And I gave my own views ...
CA: Oh, OK. I can see that would --
JS: ... which were different from General Taylor's. But in the end, nobody said anything. But then, I was 29 years old at this time, and some kid came around and said he was a stringer from Newsweek magazine and he wanted to interview me and ask what I was doing about my views. And I told him, "I'm doing mostly mathematics now, and when the war is over, then I'll do mostly their stuff." Then I did the only intelligent thing I'd done that day -- I told my local boss that I gave that interview. And he said, "What'd you say?" And I told him what I said. And then he said, "I've got to call Taylor." He called Taylor; that took 10 minutes. I was fired five minutes after that.
CA: OK.
JS: But it wasn't bad.
CA: It wasn't bad, because you went on to Stony Brook and stepped up your mathematical career. You started working with this man here. Who is this?
JS: Oh, [ Shiing-Shen ] Chern. Chern was one of the great mathematicians of the century. I had known him when I was a graduate student at Berkeley. And I had some ideas, and I brought them to him and he liked them. Together, we did this work which you can easily see up there. There it is.
CA: It led to you publishing a famous paper together. Can you explain at all what that work was?
JS: No.
(Laughter)
JS: I mean, I could explain it to somebody.
(Laughter)
CA: How about explaining this?
JS: But not many. Not many people.
CA: I think you told me it had something to do with spheres, so let's start here.
JS: Well, it did, but I'll say about that work -- it did have something to do with that, but before we get to that -- that work was good mathematics. I was very happy with it; so was Chern. It even started a little sub-field that's now flourishing. But, more interestingly, it happened to apply to physics, something we knew nothing about -- at least I knew nothing about physics, and I don't think Chern knew a heck of a lot. And about 10 years after the paper came out, a guy named Ed Witten in Princeton started applying it to string theory and people in Russia started applying it to what's called "condensed matter." Today, those things in there called Chern-Simons invariants have spread through a lot of physics. And it was amazing. We didn't know any physics. It never occurred to me that it would be applied to physics. But that's the thing about mathematics -- you never know where it's going to go.
CA: This is so incredible. So, we've been talking about how evolution shapes human minds that may or may not perceive the truth. Somehow, you come up with a mathematical theory, not knowing any physics, discover two decades later that it's being applied to profoundly describe the actual physical world. How can that happen?
JS: God knows.
(Laughter)
But there's a famous physicist named [ Eugene ] Wigner, and he wrote an essay on the unreasonable effectiveness of mathematics. Somehow, this mathematics, which is rooted in the real world in some sense -- we learn to count, measure, everyone would do that -- and then it flourishes on its own. But so often it comes back to save the day. General relativity is an example. [ Hermann ] Minkowski had this geometry, and Einstein realized, "Hey! It's the very thing in which I can cast general relativity." So, you never know. It is a mystery. It is a mystery.
CA: So, here's a mathematical piece of ingenuity. Tell us about this.
JS: Well, that's a ball -- it's a sphere, and it has a lattice around it -- you know, those squares. What I'm going to show here was originally observed by [ Leonhard ] Euler, the great mathematician, in the 1700s. And it gradually grew to be a very important field in mathematics: algebraic topology, geometry. That paper up there had its roots in this. So, here's this thing: it has eight vertices,12 edges,six faces. And if you look at the difference -- vertices minus edges plus faces -- you get two. OK, well,two. That's a good number. Here's a different way of doing it -- these are triangles covering -- this has 12 vertices and 30 edges and 20 faces,20 tiles. And vertices minus edges plus faces still equals two. And in fact, you could do this any which way -- cover this thing with all kinds of polygons and triangles and mix them up. And you take vertices minus edges plus faces -- you'll get two. Here's a different shape. This is a torus, or the surface of a doughnut: 16 vertices covered by these rectangles,32 edges,16 faces. Vertices minus edges comes out to be zero. It'll always come out to zero. Every time you cover a torus with squares or triangles or anything like that, you're going to get zero. So, this is called the Euler characteristic. And it's what's called a topological invariant. It's pretty amazing. No matter how you do it, you're always get the same answer. So that was the first sort of thrust, from the mid-1700s, into a subject which is now called algebraic topology.
CA: And your own work took an idea like this and moved it into higher-dimensional theory, higher-dimensional objects, and found new invariances?
JS: Yes. Well, there were already higher-dimensional invariants: Pontryagin classes -- actually, there were Chern classes. There were a bunch of these types of invariants. I was struggling to work on one of them and model it sort of combinatorially, instead of the way it was typically done, and that led to this work and we uncovered some new things. But if it wasn't for Mr. Euler -- who wrote almost 70 volumes of mathematics and had 13 children, who he apparently would dandle on his knee while he was writing -- if it wasn't for Mr. Euler, there wouldn't perhaps be these invariants.
CA: OK, so that's at least given us a flavor of that amazing mind in there. Let's talk about Renaissance. Because you took that amazing mind and having been a code-cracker at the NSA, you started to become a code-cracker in the financial industry. I think you probably didn't buy efficient market theory. Somehow you found a way of creating astonishing returns over two decades. The way it's been explained to me, what's remarkable about what you did wasn't just the size of the returns, it's that you took them with surprisingly low volatility and risk, compared with other hedge funds. So how on earth did you do this, Jim?
JS: I did it by assembling a wonderful group of people. When I started doing trading, I had gotten a little tired of mathematics. I was in my late 30s, I had a little money. I started trading and it went very well. I made quite a lot of money with pure luck. I mean, I think it was pure luck. It certainly wasn't mathematical modeling. But in looking at the data, after a while I realized: it looks like there's some structure here. And I hired a few mathematicians, and we started making some models -- just the kind of thing we did back at IDA [ Institute for Defense Analyses ] . You design an algorithm, you test it out on a computer. Does it work? Doesn't it work? And so on.
CA: Can we take a look at this? Because here's a typical graph of some commodity. I look at that, and I say, "That's just a random, up-and-down walk -- maybe a slight upward trend over that whole period of time." How on earth could you trade looking at that, and see something that wasn't just random?
JS: In the old days -- this is kind of a graph from the old days, commodities or currencies had a tendency to trend. Not necessarily the very light trend you see here, but trending in periods. And if you decided, OK, I'm going to predict today, by the average move in the past 20 days -- maybe that would be a good prediction, and I'd make some money. And in fact, years ago, such a system would work -- not beautifully, but it would work. You'd make money, you'd lose money, you'd make money. But this is a year's worth of days, and you'd make a little money during that period. It's a very vestigial system.
CA: So you would test a bunch of lengths of trends in time and see whether, for example, a 10-day trend or a 15-day trend was predictive of what happened next.
JS: Sure, you would try all those things and see what worked best. Trend-following would have been great in the '60s, and it was sort of OK in the '70s. By the '80s, it wasn't.
CA: Because everyone could see that. So, how did you stay ahead of the pack?
JS: We stayed ahead of the pack by finding other approaches -- shorter-term approaches to some extent. The real thing was to gather a tremendous amount of data -- and we had to get it by hand in the early days. We went down to the Federal Reserve and copied interest rate histories and stuff like that, because it didn't exist on computers. We got a lot of data. And very smart people -- that was the key. I didn't really know how to hire people to do fundamental trading. I had hired a few -- some made money, some didn't make money. I couldn't make a business out of that. But I did know how to hire scientists, because I have some taste in that department. So, that's what we did. And gradually these models got better and better, and better and better.
CA: You're credited with doing something remarkable at Renaissance, which is building this culture, this group of people, who weren't just hired guns who could be lured away by money. Their motivation was doing exciting mathematics and science.
JS: Well, I'd hoped that might be true. But some of it was money.
CA: They made a lot of money.
JS: I can't say that no one came because of the money. I think a lot of them came because of the money. But they also came because it would be fun.
CA: What role did machine learning play in all this?
JS: In a certain sense, what we did was machine learning. You look at a lot of data, and you try to simulate different predictive schemes, until you get better and better at it. It doesn't necessarily feed back on itself the way we did things. But it worked.
CA: So these different predictive schemes can be really quite wild and unexpected. I mean, you looked at everything, right? You looked at the weather, length of dresses, political opinion.
JS: Yes, length of dresses we didn't try.
CA: What sort of things?
JS: Well, everything. Everything is grist for the mill -- except hem lengths. Weather, annual reports, quarterly reports, historic data itself, volumes, you name it. Whatever there is. We take in terabytes of data a day. And store it away and massage it and get it ready for analysis. You're looking for anomalies. You're looking for -- like you said, the efficient market hypothesis is not correct.
CA: But any one anomaly might be just a random thing. So, is the secret here to just look at multiple strange anomalies, and see when they align?
JS: Any one anomaly might be a random thing; however, if you have enough data you can tell that it's not. You can see an anomaly that's persistent for a sufficiently long time -- the probability of it being random is not high. But these things fade after a while; anomalies can get washed out. So you have to keep on top of the business.
CA: A lot of people look at the hedge fund industry now and are sort of ... shocked by it, by how much wealth is created there, and how much talent is going into it. Do you have any worries about that industry, and perhaps the financial industry in general? Kind of being on a runaway train that's -- I don't know -- helping increase inequality? How would you champion what's happening in the hedge fund industry?
JS: I think in the last three or four years, hedge funds have not done especially well. We've done dandy, but the hedge fund industry as a whole has not done so wonderfully. The stock market has been on a roll, going up as everybody knows, and price-earnings ratios have grown. So an awful lot of the wealth that's been created in the last -- let's say,five or six years -- has not been created by hedge funds. People would ask me, "What's a hedge fund?" And I'd say, "One and 20." Which means -- now it's two and 20 -- it's two percent fixed fee and 20 percent of profits. Hedge funds are all different kinds of creatures.
CA: Rumor has it you charge slightly higher fees than that.
JS: We charged the highest fees in the world at one time. Five and 44, that's what we charge.
CA: Five and 44. So five percent flat,44 percent of upside. You still made your investors spectacular amounts of money.
JS: We made good returns, yes. People got very mad: "How can you charge such high fees?" I said, "OK, you can withdraw." But "How can I get more?" was what people were --
(Laughter)
But at a certain point, as I think I told you, we bought out all the investors because there's a capacity to the fund.
CA: But should we worry about the hedge fund industry attracting too much of the world's great mathematical and other talent to work on that, as opposed to the many other problems in the world?
JS: Well, it's not just mathematical. We hire astronomers and physicists and things like that. I don't think we should worry about it too much. It's still a pretty small industry. And in fact, bringing science into the investing world has improved that world. It's reduced volatility. It's increased liquidity. Spreads are narrower because people are trading that kind of stuff. So I'm not too worried about Einstein going off and starting a hedge fund.
CA: You're at a phase in your life now where you're actually investing, though, at the other end of the supply chain -- you're actually boosting mathematics across America. This is your wife, Marilyn. You're working on philanthropic issues together. Tell me about that.
JS: Well, Marilyn started -- there she is up there, my beautiful wife -- she started the foundation about 20 years ago. I think '94. I claim it was '93, she says it was '94, but it was one of those two years.
(Laughter)
We started the foundation, just as a convenient way to give charity. She kept the books, and so on. We did not have a vision at that time, but gradually a vision emerged -- which was to focus on math and science, to focus on basic research. And that's what we've done. Six years ago or so, I left Renaissance and went to work at the foundation. So that's what we do.
CA: And so Math for America is basically investing in math teachers around the country, giving them some extra income, giving them support and coaching. And really trying to make that more effective and make that a calling to which teachers can aspire.
JS: Yeah -- instead of beating up the bad teachers, which has created morale problems all through the educational community, in particular in math and science, we focus on celebrating the good ones and giving them status. Yeah, we give them extra money,15,000 dollars a year. We have 800 math and science teachers in New York City in public schools today, as part of a core. There's a great morale among them. They're staying in the field. Next year, it'll be 1,000 and that'll be 10 percent of the math and science teachers in New York [ City ] public schools.
(Applause)
CA: Jim, here's another project that you've supported philanthropically: Research into origins of life, I guess. What are we looking at here? JS: Well, I'll save that for a second. And then I'll tell you what you're looking at. Origins of life is a fascinating question. How did we get here? Well, there are two questions: One is, what is the route from geology to biology -- how did we get here? And the other question is, what did we start with? What material, if any, did we have to work with on this route? Those are two very, very interesting questions. The first question is a tortuous path from geology up to RNA or something like that -- how did that all work? And the other, what do we have to work with? Well, more than we think. So what's pictured there is a star in formation. Now, every year in our Milky Way, which has 100 billion stars, about two new stars are created. Don't ask me how, but they're created. And it takes them about a million years to settle out. So, in steady state, there are about two million stars in formation at any time. That one is somewhere along this settling-down period. And there's all this crap sort of circling around it, dust and stuff. And it'll form probably a solar system, or whatever it forms. But here's the thing -- in this dust that surrounds a forming star have been found, now, significant organic molecules. Molecules not just like methane, but formaldehyde and cyanide -- things that are the building blocks -- the seeds, if you will -- of life. So, that may be typical. And it may be typical that planets around the universe start off with some of these basic building blocks. Now does that mean there's going to be life all around? Maybe. But it's a question of how tortuous this path is from those frail beginnings, those seeds, all the way to life. And most of those seeds will fall on fallow planets.
CA: So for you, personally, finding an answer to this question of where we came from, of how did this thing happen, that is something you would love to see.
JS: Would love to see. And like to know -- if that path is tortuous enough, and so improbable, that no matter what you start with, we could be a singularity. But on the other hand, given all this organic dust that's floating around, we could have lots of friends out there. It'd be great to know.
CA: Jim, a couple of years ago, I got the chance to speak with Elon Musk, and I asked him the secret of his success, and he said taking physics seriously was it. Listening to you, what I hear you saying is taking math seriously, that has infused your whole life. It's made you an absolute fortune, and now it's allowing you to invest in the futures of thousands and thousands of kids across America and elsewhere. Could it be that science actually works? That math actually works?
JS: Well, math certainly works. Math certainly works. But this has been fun. Working with Marilyn and giving it away has been very enjoyable.
CA: I just find it -- it's an inspirational thought to me, that by taking knowledge seriously, so much more can come from it. So thank you for your amazing life, and for coming here to TED.
Thank you.
Jim Simons!
(Applause)
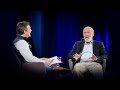
クリス・アンダーソン: あなたはいわゆる天才数学者ですね 若くしてハーバード大やMITで 教鞭と取っておられました するとNSAから 電話がかかってきたとか それはどういう話でしたか?
ジム・サイモンズ:NSA つまり アメリカ国家安全保障局が 実際に電話してきた わけではありません プリンストンに彼らの拠点があり 数学者を雇って暗号解読とかを やっていて その存在については知っていました とても良い雇用条件で 時間の半分を 本来の業務に充てていれば 残りの時間を数学の研究に使うのも 自由だったのです しかも給料が高かったので 抗しがたい魅力でした だから自分から足を運んだのです
クリス:暗号解読の任務に就いたのですね
ジム:その通りです
クリス:解雇されるまでは ですね
ジム:はい 解雇されました
クリス:どうしてそうなったのですか?
ジム:どうしてって? それは 当時ベトナム戦争の 真っ最中でしたが そこの上司の上司というのが 大の戦争好きで ニューヨーク・タイムズ日曜版に ベトナム戦争で勝利する方法について 特集記事を書いたのです 私は戦争が嫌いですから 愚かなことだと思いました それでタイムズ紙に投稿して それが掲載されました マクスウェル・テイラーの ― みんな彼を覚えているかわかりませんが 彼の元で働く人間が全部 彼に賛同しているわけではないと言って 私自身の考えを書いたのですが ―
クリス:なるほど クビになるわけだ
ジム:テイラー将軍とは 意見が異なったということです しかし その時は 何も言われませんでした 当時29歳でしたが 私のところに ニューズウィーク誌の記者だという 若者がやってきてインタビューしたいと言い 私が自分の主張に関して 何をしているのかと聞きました 私はこう答えました 「今はもっぱら数学をしています 戦争が終わったら NSAの仕事に戻ります」 そして私がその日にした 唯一聡明なことをしました インタビューを受けたことを 上司に伝えたのです 「何をしゃべったんだ」と質問され 言ったがままに報告しました 「テイラー将軍に電話せねばならない」 と彼は言い 10分ほど電話しに行き 戻って5分後には 解雇されていました
クリス:なるほど
ジム:でも 悪いことではありませんでした
クリス:それでニューヨーク大 ストーニー・ブルック校で職を得て 数学者としてのキャリアを 歩み始めたのですね そしてこの人物と一緒に 研究することになりました どういう方ですか?
ジム:シンシェン・チャーンです チャーンは20世紀を代表する 数学者の一人です 彼とはバークレー校の大学院生の時からの 知り合いでした 私にはちょっとしたアイデアがあって それを彼に話すと彼は興味を示し 共同でやることになりました 画面で見てもらうと 簡単に分かると思いますが (笑) これです
クリス: それが とても有名になる 共著論文へと繋がりました その内容について 少し紹介いただけませんか?
ジム:無理だよ
(笑)
ジム:理解できる人ならいいですが ―
(笑)
クリス: こちらの説明ならいかがですか?
ジム:そういう人は あまり多くはありませんから
クリス:その理論はこの球と 関係しているということでしたね ここから始めて下さい
ジム:たしかに関係しています あの研究のことは この後お話しします この球と関係はあるのですが その前に言っておきたいことがあります あの論文はもっぱら 数学に関するものでした 私もチャーンもそのことに 不満はありません 今では大きく発展している 数学の分野を切り開きさえしました しかし興味深いことに それが物理学にも応用されるようになったのです 私は物理なんて知らないし チャーンだって 大して知らないでしょう 論文が出て10年くらいして プリンストン大のエド・ウィッテンという人が ひも理論に応用し始めました またロシア人が凝縮系物理学という 理論にも応用し始めました 今では そのチャーン・サイモンズ不変量と 呼ばれるものが 物理学の様々な分野で使われています 驚くばかりです 私たちは物理の知識などなく 物理学に応用されるなんて 予想もしていませんでした でも それが数学の特徴なのです 何に応用されるか分かりません
クリス:素晴らしいことですね 真理を理解するか 分からないものながら 進化は人の精神をどう形作っていくのか という話をしましたが あなたは物理学のことを知らずに 数学の理論を作ったというのに それが20年後に 現実の物理の世界の 根本的なところを記述するために 応用されるのですから どうしてそんなことが 可能なのでしょう?
ジム: 神のみぞ知る です
(笑)
ユージン・ウィグナーという 有名な物理学者が 「(自然科学における)数学の不条理なまでの有効性」という論文を書いています 数学というものは ある意味で現実の世界に根付いていて 人は数えたり測ったりするようになり みんなすることですが その後 独自の発展をしていきます しかしそれが巡り巡って 現実の問題を解決するのです 一般相対性理論がその一例です ヘルマン・ミンコフスキーが その名を冠する幾何学を考え出し アインシュタインが「これこそ一般相対性理論の 記述に求めていたものだ!」と見出す 数学理論がどう使われるかなんて 分かりません 実に不思議なものです
クリス:ここに素晴らしい 数学の成果の一例があります これについて解説して下さい
ジム:これはボール ― 球で表面に格子が組まれています 正方形の形をしていますね ここで説明することは レオンハルト・オイラーによって見出されたことです 1700年代の偉大な数学者です その発見は数学の とても重要な分野である 代数的位相数幾何学へと 発展しました 私たちの論文も ここにルーツがあります では説明しましょう ここには8つの頂点、12の辺と 6つの面があります 頂点の数から辺の数を引き 面の数を足すと 2となります 2です まあそんなもんでしょう 別のケースを見てみましょう 三角形で覆ってみます 今度は12の頂点 30の辺、20の面があり 20枚のタイルで覆われていますが 頂点-辺+面は またもや2になります 実際のところ 覆うものが たとえ 三角形や他の多角形 それが混合していようとも 結果は同じで 頂点-辺+面は 2になるのです 今度は別の形です トーラスで ドーナツ状の形をしています これを長方形で覆います 頂点は16、辺は32、面の数は16です 頂点-辺+面は 0になります いつだって0です トーラスは 正方形、三角形や 他のどんなもので覆っても 0になるのです このような数を オイラーの標数といいます 位相不変量と呼ばれるものの一種です とても興味深いことです どの様にやっても いつも同じ結果が得られます この分野は1700年代中頃に芽生え 今では代数的位相幾何学と 呼ばれるものになりました
クリス:あなた方が作り上げた理論は ここにヒントを得て より高い次元の理論へと 高次元の物体へと拡げ 新たな不変量を見出したということですね
ジム:そうです しかし高次元の不変量自体は以前からありました ポントリャーギン類 それにチャーンの名が付いたのもありました こういった不変量は たくさんあって 私はそのうちの一つについて 研究していたのですが 通常行われていたのとは異なる ある種組み合せ論的な定式化をし その結果 新たな発見があったのです しかしオイラー先生がいなかったら ― 彼は70巻もの数学書を 書き上げましたが 13人も子供がいたので きっと膝の上に子供を乗せながら 本を書いていたことでしょう オイラー先生がいなかったら このような 不変量は発見されていなかったかもしれません
クリス:なるほど 素晴らしき知性の一端を見せて頂きました ではルネサンス社の話を聞かせて下さい 頭脳明晰なあなたは NSAで暗号解読の仕事に携わりましたが その後ファイナンスの暗号に 取り組むようになりました 効率的市場仮説というのを 信じなかったのだと思いますが 20年間に驚くほどのリターンを 生み出したある方法を見出しました 聞いたところによると それがすごいのは リターンが大きいだけでなく 他のヘッジファンドに比べ 驚くほど安定度が高く リスクが低いのだと どうやったら こんな事が出来たのですか?
ジム:まずは優秀な人材を集めました 私がトレーディングを始めた時 少し数学に飽きていました 30代後半で ちょっとしたお金を持っていました トレーディングを始めて これが首尾良くいきました かなり稼ぎましたが 単なる幸運でした 偶然だったと思います それは決して数学モデルとは 関係していませんでした しかし しばらくデータを見ているうちに 気が付きました そこにはある種の構造が 存在するように見えたのです そこで数学者を何人か雇い モデルをいくつか構築し始めました それはIDA(防衛分析研究所)で やっていた類の事です アルゴリズムを設計し コンピューターにかけて 上手くいくかどうか 試してみるわけです
クリス:これをご覧いただけますか? これは典型的な相場のグラフで これを見て私ならこう思います 「ランダムに上下しているな 全体を見ると少しだけ 上向きの傾向があるかも」 あなたはどうやって ランダムでない部分を見てとり 上手く取引をすることが 出来たのですか?
ジム: これは昔のものですね 古き時代のグラフです 商品や通貨の相場に トレンドがありました ここで見られるような穏やかなトレンドだけでなく 周期的なトレンドがあります それが分かったら 過去20日間の平均的な変動から 今日の値を予測します 上手く予想できれば 儲けることができます 以前には そういう方法が通用しました 完璧ではありませんが 上手くいったのです 儲けたり 損したり 儲けたり となりますが 長く続けていれば 期間全体としては ちょっとしたお金を稼げます 今や通用しないやり方です
クリス:あなたは様々なトレンドの 周期を試したのですね 例えば 10日周期、15日周期について その先が予測可能かどうか 試そうとしたのですね
ジム:様々なものを試し どれが最善かを探すわけです トレンドによる予測は60年代には 上手くいきました 70年代も そこそこ上手くいきました でも80年代は 違いました
クリス: 皆が同じことを やったからですね あなたは どうやって彼らに 先んじようとしましたか?
ジム:別の方法を考えることで 先行しました 短期の予測といったものですが ― 大きいのは大量のデータを 集めたということです 当初は手作業でした 連邦準備銀行に行って過去の利率の データを複写するといったことです コンピューターにはデータが 保存されていませんでしたからね データを沢山取得しました そして とても頭の良い人達を雇う これが鍵です ファンダメンタル投資する人を どう採用したらいいかなんて分かりませんでした 何人か雇いましたが 儲けたり 損したりで それでは十分な利益が 出せませんでした しかし科学者の採用であれば うまくできました それに関しては 目が利いたからです これが種明かしです そうやって予測モデルは 徐々に改善され さらに改善していきました
クリス:ルネサンス社の特徴的なことも あなたの業績とされていますね 高給に釣られるだけの人は 雇わないという 社風を作り上げました 数学と科学で面白いことがやれるというのが モチベーションになっているという
ジム:そうだったと願いたいですが お金も重要な要素でした
クリス:随分稼いでいますものね
ジム:金目当てで来る人が いないとは言えません 多くの人がお金に引かれて 来たと思いますが しかし同時に 面白そうだという理由もありました
クリス:機械学習は どのような役割を果たしたのですか?
ジム:ある意味で 我々がやったことは機械学習です 多くのデータを分析し 様々な予測手法を試し 徐々により良い手法を 見出していきます 必ずしもフィードバックにより 改善していくわけではありませんが 上手くいきました
クリス:予測方法の中には 意外で型破りなものもあったそうですね 天気、ドレスの長さ 政治的意見といったものまで あらゆるものを試してみたのですね?
ジム: はい でもドレスの長さは試していません
クリス: どんなものを試されましたか?
ジム:何もかもです 使えるものは何でも ― 裾の長さを別にすれば 天気、年次報告 四半期報告、歴史的データ、売上高 あるものは何でもです 毎日 数テラバイトのデータを取り込んで 保存、加工し 分析に使えるようにします そして異常値を探し出します あなたが言われたように 効率的市場仮説というのは 正しくありません
クリス:異常値1つとれば ランダムでしかありませんが 複数の奇妙な異常値の間に 関連性を見つけ出すのが 鍵だとか
ジム:単一の異常値は ランダムなものかもしれませんが しかし 十分なデータを集めれば そうでないと判断できます 十分に長い時間持続するような 異常が見つかります そのようなことが偶然に起こる確率は 高くありません しかしそのような異常も いずれ消えてしまいます ですから 常に 先を行く必要があります
クリス: ヘッジファンド業界を見て 多くの人は ある意味 ショックを受けています 多大な富がそこで産み出され そこには多くの才能ある者 関わっているからです この業界 あるいは 金融業界一般に対して 懸念はありませんか? 暴走列車のようで ― 何というか 格差の拡大を助長しているとか ヘッジファンド業界で起きていることを どう擁護しますか?
ジム:この3、4年ほどは ヘッジファンドはさほど 上手くいっていません 我々の商売は首尾よくいきましたが ヘッジファンド業界全体としては 捗々しくありません 一方 株式市場はご存じのとおり 上昇しています 株価収益率は上がりました この5~6年でもたらされた 富の大部分は ヘッジファンドによるものではありません 「ヘッジファンドって何?」 と聞かれたら 「1と20」と答えます 今では「2と20」になっていますが ― 2%の固定手数料と 利益の20%をいただくという意味です ヘッジファンドというのは 別種の生き物なんです
クリス:あなた方はもう少し高い手数料を 取っているという噂ですが
ジム:ある時点で我々は 業界で最も高い手数料を取っていました 「5 と 44」です
クリス:「5 と 44」というと 5%の固定手数料と 利益の44%ということですね それでも投資家は 大変な利益を得られた
ジム:実際 高いリターンを得ました 人々は怒りだしました 何でそんな高い手数料を取るのだと 「止めてもいいですよ」 と私は言いました どうやればもっと儲けられるかというのが みんなの考えることです
(笑)
しかし これはあなたに お話ししたと思いますが ある時点で外部からの投資を 受け入れなくなりました
クリス:しかし優秀な数学者が ヘッジファンド業界に集中し 世界のその他の問題解決のための 人材が不足することを憂慮すべきでは ありませんか?
ジム:数学者だけでなく 天文学者や物理学者なども雇っています しかし気にする程のことではありません 今でも業界としては 小さなものです 事実 投資の分野に 科学を持ち込んだことで 世界は良くなったと思います 不安定性が抑えられ 資金の流動性が高まりました 取引が増えることで 商品間のスプレッド(価格差)が縮小しました 私はアインシュタインのような天才が科学を捨て ヘッジファンドを始めることを憂慮はしていません
クリス:あなたは今の時点になって 反対の供給側に 投資するようになりましたね アメリカ中で 数学の後押しをしています この方は奥さんのマリリンさんですね お2人で慈善活動をなさっています このことについてお話し下さい
ジム:そこに写っている 美しき我が妻マリリンは 財団を約20年前に設立しました 1994年だったと思います 私が93年だと主張しても 彼女は94年と言います 何れにしろ どちらかの年です
(笑)
我々は財団を設立しました 寄付するのには都合の良い方法でしたから 彼女が帳簿の管理などをしていました 当時 はっきりしたビジョンはありませんでしたが 徐々に芽生えてきました 基礎研究を重視し 数学や科学に焦点を当てるということです そして これを実行に移しました 私は6年ほど前に ルネッサンス社を辞めて 財団で働くようになりました 今でも働いています
クリス:「Math for America」では 米国内の数学教師に資金を与え 追加報酬を与えたり 支援や指導を行っています 教育の効率を高め 教師たちが目指せる 使命を提示していますね
ジム:問題のある教師を 叱責するよりその方がいいです 特に数学や科学の分野では 叱責しても 教育界全体で やる気の低下を招くだけです そこで 優秀な者を表彰し 地位を与えることに注力しました 年間2百万円弱の報奨金を与えます 現在 ニューヨーク市の公立校にいる 数学や科学の教師 800人を支援しており 彼らはその中心的役割を担っています 彼らにはやる気があり 教育現場に留まっています 来年にはニューヨーク市の公立校の 数学と科学の教師の10%にあたる 1千人へと拡大します
(拍手)
クリス:あなたは別のプロジェクトに 対しても慈善的支援を行っていますね 生命の起源に関するものだとか どのような研究ですか? ジム:それにお答えする前に あなたが質問された 生命の起源の謎とは とても興味深いものだと言いたいのです どのようにして誕生したのでしょうか? 2つの謎があります 地質学的なものから 生物学的なものへの遷移は どのように起きたのか というのが一つ もう一つの謎は 何から始まったのかということ その遷移において どの物質が起源となったのか? これら2つは とても興味深い謎です 最初の謎は 地質からRNAのようなものに至るまでの 途方もない進化の道筋についてで その仕組みは どのようなものだったのかということ もう一方の謎 生命体をなす物質を得る過程は 従来の説を超えたものなのかもしれません この写真は星の形成過程を示しています 我が銀河系には約千億個の星がありますが 毎年2つほどの新しい星が誕生しています その仕組みについては知りませんが とにかく誕生しています 星ができるまでには 百万年ほどの時間がかかります そのため 定常的に 形成過程の星が 2百万個あります 写真はこの形成過程にある星です その周りを取り巻いて 塵のようなものがあります そして太陽系みたいなものが形成されます ここに注目すべきことがあります 形成過程の星の 周辺を取り巻く塵には 重要な有機分子が含まれていることが 分かってきました メタンといった分子だけでなく ホルムアルデヒドやシアン化物といった 生命の種ともいえる基本物質が あるのです これは当たり前に 起きていることなのかもしれません そういう生命の基本物質から 惑星ができるというのは 典型的なことなのかもしれません ならば 生命は至る所に居るのでは? そうなのかもしれません しかし 種となる物質が存在するというだけの はかない原始状態から 生命誕生に至る過程が どれ程に 大変なことなのかという疑問が残されます 種となる物質は 休眠している惑星に 降り注ぐことになります
クリス:つまり あなたは 生命の起源と誕生に関する謎を 解き明かしたいとお考えなのですね
ジム:解明されればと願っています 生命の誕生が 開始条件に関わらず ほぼ不可能なほど起こりにくいなら 地球に生命があるのは 特異的なことということになります 逆にさほど難しくないなら 宇宙に漂う有機物の塵から そこらじゅうに 生命は存在するのかもしれません ぜひ知りたいところです
クリス:2年前 イーロン・マスクに 話を聞く機会があったのですが 彼に成功の秘密について尋ねると 物理に真剣に取り組むことだと 彼は答えました あなたの話によると あなたは数学に真剣に取り組み それがあなたの人生全体に 力を与えています そして巨万の富を得て それをアメリカや世界の大勢の子供たちの 将来のために投資することを 可能にしています これは科学が役に立つ ということでしょうか? 数学は役に立つのか?
ジム:数学は間違いなく 役に立ちますよ それに楽しくもあります マリリンと一緒に働き 人々に貢献する これはとても楽しいことです
クリス:知に真剣に取り組むことで かくも多くのものが得られうるということに とても感銘を受けました TEDにお越し頂いて あなたの 素晴らしい人生について聞かせて頂き
ありがとうございました
ジム・サイモンズでした!
(拍手)
品詞分類
- 主語
- 動詞
- 助動詞
- 準動詞
- 関係詞等
TED 日本語
TED Talks
関連動画
人類の未来を変革する10年 あるいは地球が不安定化する10年ヨハン・ロックストローム
2020.10.15ビッグデータと小規模農場と2つのトマトの物語エリン・バウムガートナー
2020.09.11屋内垂直農場が農業の未来なのか?スチュアート・オダ
2020.02.07新しい政治の物語はすべてを変えられるジョージ・モンビオ
2019.09.05一過性の都市に見る驚異の建築術ラフール・メロトラ
2019.08.29政治家をあるものに置き換える大胆な構想セザー・ヒダルゴ
2019.04.03気候のための行動への心開かれる訴え | TED Talkグレタ・サンバーグ
おすすめ 12019.02.13気候変動に対してあなたが出来るいちばん大事なことキャサリン・ヘイホー
2019.01.11何をやるときにも自分に問う3つの質問ステイシー・エイブラムス
2019.01.08子供たちに学ぶ都市計画マーラ・ミンツァー
2018.11.28テクノロジー企業があなたを欺いてデータとプライバシーを放棄させる手口フィン・リュツォー=ホルム・ミスタッド
2018.11.21街の東側に貧困地域が多いのはなぜかスティーブン・デベリー
2018.09.12無人操縦エアタクシーが移動方法をどう変えるかロディン・リアソフ
2018.07.20悪夢のような子供向けYouTube動画 ― 今のインターネットのどこが間違っているのかジェームズ・ブライドル
2018.07.13もしも無作為に選ばれた人たちが政治家になったら - TED Talkブレット・ヘニッヒ
2018.06.26オンラインの嫌がらせコメントから、オフラインの建設的な対話を生む方法ディラン・マロン
2018.05.18
洋楽 おすすめ
RECOMMENDS
洋楽歌詞
ダイナマイトビーティーエス
洋楽最新ヒット2020.08.20ディス・イズ・ミーグレイテスト・ショーマン・キャスト
洋楽人気動画2018.01.11グッド・ライフGイージー、ケラーニ
洋楽人気動画2017.01.27ホワット・ドゥ・ユー・ミーン?ジャスティン・ビーバー
洋楽人気動画2015.08.28ファイト・ソングレイチェル・プラッテン
洋楽人気動画2015.05.19ラヴ・ミー・ライク・ユー・ドゥエリー・ゴールディング
洋楽人気動画2015.01.22アップタウン・ファンクブルーノ・マーズ、マーク・ロンソン
洋楽人気動画2014.11.20ブレイク・フリーアリアナ・グランデ
洋楽人気動画2014.08.12ハッピーファレル・ウィリアムス
ポップス2014.01.08カウンティング・スターズワンリパブリック
ロック2013.05.31ア・サウザンド・イヤーズクリスティーナ・ペリー
洋楽人気動画2011.10.26ユー・レイズ・ミー・アップケルティック・ウーマン
洋楽人気動画2008.05.30ルーズ・ユアセルフエミネム
洋楽人気動画2008.02.21ドント・ノー・ホワイノラ・ジョーンズ
洋楽人気動画2008.02.15オンリー・タイムエンヤ
洋楽人気動画2007.10.03ミス・ア・シングエアロスミス
ロック2007.08.18タイム・トゥ・セイ・グッバイサラ・ブライトマン
洋楽人気動画2007.06.08シェイプ・オブ・マイ・ハートスティング
洋楽人気動画2007.03.18ウィ・アー・ザ・ワールド(U.S.A. フォー・アフリカ)マイケル・ジャクソン
洋楽人気動画2006.05.14ホテル・カリフォルニアイーグルス
ロック2005.07.06