
TED日本語
TED Talks(英語 日本語字幕付き動画)
TED日本語 - アン・ミルグラム: 犯罪抑止の鍵はハイテク統計
TED Talks
犯罪抑止の鍵はハイテク統計
Why smart statistics are the key to fighting crime
アン・ミルグラム
Anne Milgram
内容
2007年にニュージャージー州の州司法長官になったアン・ミルグラムは驚くべき事実に気づきました。自分達がどんな人間を刑務所に送っているのか把握していない上に、判決によって社会が本当に安全になったかを知る術が無かったのです。ここから彼女のインスピレーションに満ちた探求、すなわち、アメリカの刑事司法制度にデータ分析と統計分析を取り入れる試みが始まったのです。
字幕
SCRIPT
Script
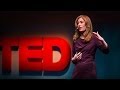
In 2007, I became the attorney general of the state of New Jersey. Before that, I'd been a criminal prosecutor, first in the Manhattan district attorney's office, and then at the United States Department of Justice.
But when I became the attorney general,two things happened that changed the way I see criminal justice. The first is that I asked what I thought were really basic questions. I wanted to understand who we were arresting, who we were charging, and who we were putting in our nation's jails and prisons. I also wanted to understand if we were making decisions in a way that made us safer. And I couldn't get this information out. It turned out that most big criminal justice agencies like my own didn't track the things that matter. So after about a month of being incredibly frustrated, I walked down into a conference room that was filled with detectives and stacks and stacks of case files, and the detectives were sitting there with yellow legal pads taking notes. They were trying to get the information I was looking for by going through case by case for the past five years. And as you can imagine, when we finally got the results, they weren't good. It turned out that we were doing a lot of low-level drug cases on the streets just around the corner from our office in Trenton.
The second thing that happened is that I spent the day in the Camden, New Jersey police department. Now, at that time, Camden, New Jersey, was the most dangerous city in America. I ran the Camden Police Department because of that. I spent the day in the police department, and I was taken into a room with senior police officials, all of whom were working hard and trying very hard to reduce crime in Camden. And what I saw in that room, as we talked about how to reduce crime, were a series of officers with a lot of little yellow sticky notes. And they would take a yellow sticky and they would write something on it and they would put it up on a board. And one of them said, "We had a robbery two weeks ago. We have no suspects." And another said, "We had a shooting in this neighborhood last week. We have no suspects." We weren't using data-driven policing. We were essentially trying to fight crime with yellow Post-it notes.
Now, both of these things made me realize fundamentally that we were failing. We didn't even know who was in our criminal justice system, we didn't have any data about the things that mattered, and we didn't share data or use analytics or tools to help us make better decisions and to reduce crime. And for the first time, I started to think about how we made decisions. When I was an assistant D.A., and when I was a federal prosecutor, I looked at the cases in front of me, and I generally made decisions based on my instinct and my experience. When I became attorney general, I could look at the system as a whole, and what surprised me is that I found that that was exactly how we were doing it across the entire system -- in police departments, in prosecutors's offices, in courts and in jails. And what I learned very quickly is that we weren't doing a good job. So I wanted to do things differently. I wanted to introduce data and analytics and rigorous statistical analysis into our work. In short, I wanted to moneyball criminal justice.
Now, moneyball, as many of you know, is what the Oakland A's did, where they used smart data and statistics to figure out how to pick players that would help them win games, and they went from a system that was based on baseball scouts who used to go out and watch players and use their instinct and experience, the scouts' instincts and experience, to pick players, from one to use smart data and rigorous statistical analysis to figure out how to pick players that would help them win games.
It worked for the Oakland A's, and it worked in the state of New Jersey. We took Camden off the top of the list as the most dangerous city in America. We reduced murders there by 41 percent, which actually means 37 lives were saved. And we reduced all crime in the city by 26 percent. We also changed the way we did criminal prosecutions. So we went from doing low-level drug crimes that were outside our building to doing cases of statewide importance, on things like reducing violence with the most violent offenders, prosecuting street gangs, gun and drug trafficking, and political corruption.
And all of this matters greatly, because public safety to me is the most important function of government. If we're not safe, we can't be educated, we can't be healthy, we can't do any of the other things we want to do in our lives. And we live in a country today where we face serious criminal justice problems. We have 12 million arrests every single year. The vast majority of those arrests are for low-level crimes, like misdemeanors,70 to 80 percent. Less than five percent of all arrests are for violent crime. Yet we spend 75 billion, that's b for billion, dollars a year on state and local corrections costs. Right now, today, we have 2.3 million people in our jails and prisons. And we face unbelievable public safety challenges because we have a situation in which two thirds of the people in our jails are there waiting for trial. They haven't yet been convicted of a crime. They're just waiting for their day in court. And 67 percent of people come back. Our recidivism rate is amongst the highest in the world. Almost seven in 10 people who are released from prison will be rearrested in a constant cycle of crime and incarceration.
So when I started my job at the Arnold Foundation, I came back to looking at a lot of these questions, and I came back to thinking about how we had used data and analytics to transform the way we did criminal justice in New Jersey. And when I look at the criminal justice system in the United States today, I feel the exact same way that I did about the state of New Jersey when I started there, which is that we absolutely have to do better, and I know that we can do better.
So I decided to focus on using data and analytics to help make the most critical decision in public safety, and that decision is the determination of whether, when someone has been arrested, whether they pose a risk to public safety and should be detained, or whether they don't pose a risk to public safety and should be released. Everything that happens in criminal cases comes out of this one decision. It impacts everything. It impacts sentencing. It impacts whether someone gets drug treatment. It impacts crime and violence. And when I talk to judges around the United States, which I do all the time now, they all say the same thing, which is that we put dangerous people in jail, and we let non-dangerous, nonviolent people out. They mean it and they believe it. But when you start to look at the data, which, by the way, the judges don't have, when we start to look at the data, what we find time and time again, is that this isn't the case. We find low-risk offenders, which makes up 50 percent of our entire criminal justice population, we find that they're in jail. Take Leslie Chew, who was a Texas man who stole four blankets on a cold winter night. He was arrested, and he was kept in jail on 3,500 dollars bail, an amount that he could not afford to pay. And he stayed in jail for eight months until his case came up for trial, at a cost to taxpayers of more than 9,000 dollars. And at the other end of the spectrum, we're doing an equally terrible job. The people who we find are the highest-risk offenders, the people who we think have the highest likelihood of committing a new crime if they're released, we see nationally that 50 percent of those people are being released.
The reason for this is the way we make decisions. Judges have the best intentions when they make these decisions about risk, but they're making them subjectively. They're like the baseball scouts 20 years ago who were using their instinct and their experience to try to decide what risk someone poses. They're being subjective, and we know what happens with subjective decision making, which is that we are often wrong. What we need in this space are strong data and analytics.
What I decided to look for was a strong data and analytic risk assessment tool, something that would let judges actually understand with a scientific and objective way what the risk was that was posed by someone in front of them. I looked all over the country, and I found that between five and 10 percent of all U.S. jurisdictions actually use any type of risk assessment tool, and when I looked at these tools, I quickly realized why. They were unbelievably expensive to administer, they were time-consuming, they were limited to the local jurisdiction in which they'd been created. So basically, they couldn't be scaled or transferred to other places.
So I went out and built a phenomenal team of data scientists and researchers and statisticians to build a universal risk assessment tool, so that every single judge in the United States of America can have an objective, scientific measure of risk. In the tool that we've built, what we did was we collected 1.5 million cases from all around the United States, from cities, from counties, from every single state in the country, the federal districts. And with those 1.5 million cases, which is the largest data set on pretrial in the United States today, we were able to basically find that there were 900-plus risk factors that we could look at to try to figure out what mattered most. And we found that there were nine specific things that mattered all across the country and that were the most highly predictive of risk. And so we built a universal risk assessment tool. And it looks like this. As you'll see, we put some information in, but most of it is incredibly simple, it's easy to use, it focuses on things like the defendant's prior convictions, whether they've been sentenced to incarceration, whether they've engaged in violence before, whether they've even failed to come back to court. And with this tool, we can predict three things. First, whether or not someone will commit a new crime if they're released. Second, for the first time, and I think this is incredibly important, we can predict whether someone will commit an act of violence if they're released. And that's the single most important thing that judges say when you talk to them. And third, we can predict whether someone will come back to court. And every single judge in the United States of America can use it, because it's been created on a universal data set.
What judges see if they run the risk assessment tool is this -- it's a dashboard. At the top, you see the New Criminal Activity Score,six of course being the highest, and then in the middle you see, "Elevated risk of violence." What that says is that this person is someone who has an elevated risk of violence that the judge should look twice at. And then, towards the bottom, you see the Failure to Appear Score, which again is the likelihood that someone will come back to court.
Now I want to say something really important. It's not that I think we should be eliminating the judge's instinct and experience from this process. I don't. I actually believe the problem that we see and the reason that we have these incredible system errors, where we're incarcerating low-level, nonviolent people and we're releasing high-risk, dangerous people, is that we don't have an objective measure of risk. But what I believe should happen is that we should take that data-driven risk assessment and combine that with the judge's instinct and experience to lead us to better decision making. The tool went statewide in Kentucky on July 1, and we're about to go up in a number of other U.S. jurisdictions. Our goal, quite simply, is that every single judge in the United States will use a data-driven risk tool within the next five years. We're now working on risk tools for prosecutors and for police officers as well, to try to take a system that runs today in America the same way it did 50 years ago, based on instinct and experience, and make it into one that runs on data and analytics.
Now, the great news about all this, and we have a ton of work left to do, and we have a lot of culture to change, but the great news about all of it is that we know it works. It's why Google is Google, and it's why all these baseball teams use moneyball to win games. The great news for us as well is that it's the way that we can transform the American criminal justice system. It's how we can make our streets safer, we can reduce our prison costs, and we can make our system much fairer and more just. Some people call it data science. I call it moneyballing criminal justice.
Thank you.
(Applause)
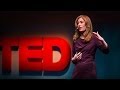
2007年に 私はニュージャージー州の 州司法長官になりました それまではマンハッタン地区の 地区検察局や 司法省で検察官をしていました
州司法長官になって 刑事裁判の見方が変わる 2つの出来事がありました 1つ目は根本的な疑問を 抱くようになったことです 自分達が どんな人間を 逮捕し 告訴して 刑務所や拘置所に 送っているのだろうか? また 社会が もっと安全になるような 判決を下しているのだろうか? でも そういう情報は 手に入りませんでした ニュージャージー州を含む 大規模な刑事司法機関では 肝心な点を追跡調査して いなかったのです 不満を募らせながら 1か月待ち 刑事が居並ぶ会議室に 乗り込みました 事件ファイルが 山積みになっていて 皆 黄色のレポート用紙に メモを取っていました 必要な情報を 手に入れるために 過去5年間の事件を 全てチェックさせたのです すると 皆さんの想像通り ― ひどい状況が明らかになりました 調査によると扱った事件の多くが トレントンにある自分達の職場の 周辺で発生した ― 比較的軽い 麻薬犯罪だったのです
2つ目の出来事とは ニュージャージー州 カムデン警察署での経験です 当時カムデンは アメリカで最も危険な街でした だから私がカムデン署を 指揮することになったのです 私はその日 署を訪れ カムデンの犯罪を減らそうと 全力を尽くしている ― 警察幹部の部屋に通されました 私達が犯罪撲滅について 話し合っている時 目にしたのは 黄色い付箋を 大量に持った警官達でした 皆 付箋にメモを書いて ボードに貼り 次々と報告していきます 「2週間前 強盗事件発生 ― 容疑者情報なし」 「先週この付近で銃撃事件発生 容疑者情報なし」・・・ 捜査にデータを 活用していなかったのです 黄色の付箋だけを頼りに 犯罪に闘いを 挑むようなものです
この2つの出来事がきっかけで 根本的な失敗に 気づいたのです 私達は どんな人間が 裁判を受けているか知らず 必要なデータがない上に 共有もしていない・・・ 適切な判断を下したり 犯罪を減らすための データ分析手法やツールさえ 使っていませんでした 私は 初めて判決に至る過程を 検討し始めました 地方検事補 時代も 連邦検事だった時も 私は目の前の事件に集中し 勘と経験だけを頼りに 判決を下してきました 州司法長官になって 制度全体が見えるようになると 驚くべき発見がありました 司法制度における どの部門でも 勘と経験だけで 判断していたのです 警察署でも検察局でも 裁判所でも刑務所でも同じでした 上手くいっていないのは 明白だったので 別の方法が 必要だと感じました データや分析法や 厳密な統計解析の導入が 必要だと思ったのです 要は刑事司法制度界の 「マネーボール」です
ご存じの通り マネーボールとは オークランド・A's が 勝利に貢献できる選手を 獲得するために データと統計を 駆使した手法のことです かつてはスカウトが 実際に選手を見て 勘と経験を頼りに 選手を獲得していましたが 今ではデータと 厳密な統計分析によって 勝利に貢献できる選手を 選びだしています
A's で成功した この手法は ニュージャージーでも成功しました カムデンは全米で最も危険な街という 汚名を返上しました 殺人事件は41%減少しました つまり37人の命が 救われたのです 犯罪の総数は26%減少しました また刑事訴追の方法も 改めました 私達の身の回りで起こる ― 比較的軽い麻薬犯罪よりも 州全体に関わる事件 例えば 重大な暴力犯罪の抑止や ストリートギャングの摘発 ― 銃と麻薬の取引や 政治汚職の摘発に力を入れました
どれも非常に重要です なぜなら 治安こそが 政府の最も重要な 仕事だと考えるからです 安全でなければ 教育も健康も保障できません 自分のやりたいことが 不可能になるのです 現在この国は 刑事司法制度上の 深刻な問題を抱えています 毎年1,200万人が逮捕されますが そのほとんどは 重要度の低い軽犯罪で 70~80%を占めます 凶悪犯罪は 逮捕総数の わずか5%未満です それでも州や地方で 犯罪者の更正にかかるコストは 750億ドルにのぼります 現在 230万人が拘置所や刑務所に 収監されています これは治安上 極めて深刻な状況です というのも拘置所に 収容されている人間の 3分の2は 裁判の開始を 待っているだけなのです 彼らは有罪判決を 受けたわけではなく ただ出廷の時を待っています さらに67%が再犯を重ねます 州の再犯率は 世界的に見ても最悪です 刑務所から10人釈放されても 7人程度が再逮捕され 犯罪と監獄生活を 繰り返しています
私がアーノルド財団で 働きはじめた時 ― これまでの問題を振り返りました データと分析法を どう活用して ニュージャージー州の刑事司法制度を 改革したか振り返ったのです 現在のアメリカの 刑事司法制度には ニュージャージー州と 同じ課題があると思います つまり まだまだ 改善の余地はあるし 改善できるはずです
そこで 私が集中して 取り組むことにしたのは 治安上 最も重要な判断で データ分析を使うことです そのような判断の一つは 誰かを逮捕した時に 治安上のリスクが高いと 考えて勾留するか リスクは低いと考えて 釈放するかを 決めるような場合です この判断こそが 刑事裁判の出発点で 全てに影響を及ぼします 量刑の判断にも 薬物治療の必要性にも 暴力や犯罪にも影響を及ぼします 最近 全国の判事から 話を聞く機会が多いのですが 全員が こう言います 「自分達は 危険な人間を 刑務所に送り そうではない人間は 釈放している」 全員がそう確信しています でも判事達は データを持っていません 実際にデータを検討すると それに当てはまらないケースが しばしば見つかるのです 刑事裁判を受けた者の 50%を占める 危険度の低い犯罪者が 刑務所に入っています 例えばテキサス出身の レズリー・チューは 寒い冬の夜に 毛布を4枚盗んで 逮捕されましたが 3,500ドルの保釈金を 払う事ができず 拘置所に入りました それから裁判が始まるまで 8か月も勾留されたのです 納税者の負担額は 9,000ドル以上になります 逆の場合でも 状況は深刻です 極めて危険性が 高いと判断され 釈放された場合 再犯の可能性が 非常に高い犯罪者の内 ― 実に50%が 釈放されています
こうなった原因は 判断の下し方にあります 判事はリスクの見極めに 最善を尽くしていますが 判断が主観的なのです 20年前 野球のスカウト達が 勘と経験だけを頼りに リスクを評価したのと 同じことをしているのです 判事達は主観で判断しています ただ主観的な判断は しばしば誤りにつながります この分野に必要なのは 確かなデータと分析法なのです
私が求めていたものは 確固たるデータと 分析的なリスク評価ツール ― すなわち 判事の前にいる人間が どんな危険性を持つかを 科学的 客観的に 捉えるためのツールでした 全国的に見ると 何らかのリスク評価ツールを 利用しているのは 全体の わずか5~10%でした 実際のツールを見ていくと 原因はすぐにわかりました どれも管理コストが恐ろしく高く 時間もかかり 地元でしか使えない ― ツールばかりだったのです そのため 基本的に 対象エリアを広げたり 転用はできませんでした
だから私はデータ科学者や 研究者や統計学者からなる 優秀なチームを編成し どこでも使える リスク評価ツールを製作しました 目指したのは全米の判事全員が 客観的かつ科学的な リスク評価ができることです このツールを使って 私達は150万件の 事例を集めました アメリカ全土 つまり 市や郡の裁判所 ― すべての州裁判所と 連邦地裁からです そして 公判前の 事例データとしては 全米で最大規模となる ― この150万例から 900以上のリスク要因を見つけ どの要素が 最も重要なのかを 突き止めようとしました その結果 全国的に共通し 最もリスクを予測しやすい 要因が9つあると わかりました こうして ユニバーサルな リスク評価ツールが出来たのです 画面をご覧ください 多少の入力は必要ですが 全体的に とてもシンプルで 使うのは簡単です このツールが扱うのは 被告の前科や 禁固刑を受けた経験 暴力事件への関与や 裁判所に出頭しなかった前歴です このツールで 3つの予測が可能になります まず 釈放後に別の犯罪を 犯す可能性の予測です 2つ目は 初の試みですが とても重要と思われることで 釈放後に暴力事件を 起こす可能性の予測です これは どの判事も 重要な要素だと考えています 最後に 裁判所に出頭する ― 可能性の予測です アメリカの判事なら 誰でも このツールを利用できます どこにでも当てはまる データに基づいているからです
リスク評価ツールを起動すると ダッシュボードが現れます 一番上は 「新規犯罪スコア」で 最高値は「6」です その下は「暴力リスクの増加度」です この値は 対象となる人物が 暴力的な傾向が強いかどうかを 判事が検討するために使います さらに その下 ― 「未出頭スコア」は 裁判所に再び出頭する 可能性を示しています
さて ここで強調したい点があります 私はリスク評価において 判事の勘と経験を すべて排除すべきだとは 考えていません そうすべきではありません 私達が直面している問題 つまり 非暴力的な者を刑務所に入れ リスクが高い危険な者を 釈放するという 制度上のひどい誤りが 起きる原因は 客観的にリスクを評価する 手段がないことです しかし これからは データに基づくリスク評価と 判事の勘や経験を 組み合わせることで よりよい判断を 目指すべきだと考えます このツールは7月1日に ケンタッキー州全域で稼動し 他の管轄区域にも 広がりつつあります 私達の目標は ただひとつ ― 5年以内に 全米の判事が このリスク評価ツールを 使うようになることです 私達は現在 ― 検察官や警官用の ツールにも取り掛かっています 現在のやり方は 50年前と同じで 勘と経験に頼っていますが これをデータと分析による システムに替えたいのです
確かに まだ課題は 山ほど残っています 考え方も変える必要があります ただ この変革の素晴らしい点は 効果が証明されていることです Googleはデータ分析により成功し 野球チームは勝つために 「マネーボール」を採用するのです この手法が素晴らしいのは アメリカの刑事司法制度を 改善できるからです 私達の街はもっと安全になり 刑務所のコストは減り 制度は よりフェアで 公正なものになるのです これを「データ科学」と呼ぶ人もいますが 私にとっては刑事司法界の マネーボールなのです
ありがとうございました
(拍手)
品詞分類
- 主語
- 動詞
- 助動詞
- 準動詞
- 関係詞等
TED 日本語
TED Talks
関連動画
人類の未来を変革する10年 あるいは地球が不安定化する10年ヨハン・ロックストローム
2020.10.15ビッグデータと小規模農場と2つのトマトの物語エリン・バウムガートナー
2020.09.11屋内垂直農場が農業の未来なのか?スチュアート・オダ
2020.02.07新しい政治の物語はすべてを変えられるジョージ・モンビオ
2019.09.05一過性の都市に見る驚異の建築術ラフール・メロトラ
2019.08.29政治家をあるものに置き換える大胆な構想セザー・ヒダルゴ
2019.04.03気候のための行動への心開かれる訴え | TED Talkグレタ・サンバーグ
おすすめ 12019.02.13気候変動に対してあなたが出来るいちばん大事なことキャサリン・ヘイホー
2019.01.11何をやるときにも自分に問う3つの質問ステイシー・エイブラムス
2019.01.08子供たちに学ぶ都市計画マーラ・ミンツァー
2018.11.28テクノロジー企業があなたを欺いてデータとプライバシーを放棄させる手口フィン・リュツォー=ホルム・ミスタッド
2018.11.21街の東側に貧困地域が多いのはなぜかスティーブン・デベリー
2018.09.12無人操縦エアタクシーが移動方法をどう変えるかロディン・リアソフ
2018.07.20悪夢のような子供向けYouTube動画 ― 今のインターネットのどこが間違っているのかジェームズ・ブライドル
2018.07.13もしも無作為に選ばれた人たちが政治家になったら - TED Talkブレット・ヘニッヒ
2018.06.26オンラインの嫌がらせコメントから、オフラインの建設的な対話を生む方法ディラン・マロン
2018.05.18
洋楽 おすすめ
RECOMMENDS
洋楽歌詞
ダイナマイトビーティーエス
洋楽最新ヒット2020.08.20ディス・イズ・ミーグレイテスト・ショーマン・キャスト
洋楽人気動画2018.01.11グッド・ライフGイージー、ケラーニ
洋楽人気動画2017.01.27ホワット・ドゥ・ユー・ミーン?ジャスティン・ビーバー
洋楽人気動画2015.08.28ファイト・ソングレイチェル・プラッテン
洋楽人気動画2015.05.19ラヴ・ミー・ライク・ユー・ドゥエリー・ゴールディング
洋楽人気動画2015.01.22アップタウン・ファンクブルーノ・マーズ、マーク・ロンソン
洋楽人気動画2014.11.20ブレイク・フリーアリアナ・グランデ
洋楽人気動画2014.08.12ハッピーファレル・ウィリアムス
ポップス2014.01.08カウンティング・スターズワンリパブリック
ロック2013.05.31ア・サウザンド・イヤーズクリスティーナ・ペリー
洋楽人気動画2011.10.26ユー・レイズ・ミー・アップケルティック・ウーマン
洋楽人気動画2008.05.30ルーズ・ユアセルフエミネム
洋楽人気動画2008.02.21ドント・ノー・ホワイノラ・ジョーンズ
洋楽人気動画2008.02.15オンリー・タイムエンヤ
洋楽人気動画2007.10.03ミス・ア・シングエアロスミス
ロック2007.08.18タイム・トゥ・セイ・グッバイサラ・ブライトマン
洋楽人気動画2007.06.08シェイプ・オブ・マイ・ハートスティング
洋楽人気動画2007.03.18ウィ・アー・ザ・ワールド(U.S.A. フォー・アフリカ)マイケル・ジャクソン
洋楽人気動画2006.05.14ホテル・カリフォルニアイーグルス
ロック2005.07.06