
TED日本語
TED Talks(英語 日本語字幕付き動画)
TED日本語 - セバスチャン・ワーニック: データから人気テレビ番組を作るには
TED Talks
データから人気テレビ番組を作るには
How to use data to make a hit TV show
セバスチャン・ワーニック
Sebastian Wernicke
内容
たくさんデータを集めると、よりよい意思決定ができるようになるでしょうか?AmazonやGoogle、Netflixといった競争力が高くデータに強い企業は、データ分析だけでは常に最適な結果は出せないことに気づいています。データサイエンティストのセバスチャン・ワーニックが、純粋にデータだけに基づいて決断する場合、どこがうまくいかないのか分析し、賢いデータの使い方を提案します。
字幕
SCRIPT
Script
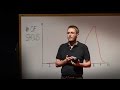
Roy Price is a man that most of you have probably never heard about, even though he may have been responsible for 22 somewhat mediocre minutes of your life on April 19,2013. He may have also been responsible for 22 very entertaining minutes, but not very many of you. And all of that goes back to a decision that Roy had to make about three years ago.
So you see, Roy Price is a senior executive with Amazon Studios. That's the TV production company of Amazon. He's 47 years old, slim, spiky hair, describes himself on Twitter as "movies, TV, technology, tacos." And Roy Price has a very responsible job, because it's his responsibility to pick the shows, the original content that Amazon is going to make. And of course that's a highly competitive space. I mean, there are so many TV shows already out there, that Roy can't just choose any show. He has to find shows that are really, really great. So in other words, he has to find shows that are on the very right end of this curve here.
So this curve here is the rating distribution of about 2,500 TV shows on the website IMDB, and the rating goes from one to 10, and the height here shows you how many shows get that rating. So if your show gets a rating of nine points or higher, that's a winner. Then you have a top two percent show. That's shows like "Breaking Bad," "Game of Thrones," "The Wire," so all of these shows that are addictive, whereafter you've watched a season, your brain is basically like, "Where can I get more of these episodes?" That kind of show. On the left side, just for clarity, here on that end, you have a show called "Toddlers and Tiaras" --
(Laughter)
-- which should tell you enough about what's going on on that end of the curve.
Now, Roy Price is not worried about getting on the left end of the curve, because I think you would have to have some serious brainpower to undercut "Toddlers and Tiaras." So what he's worried about is this middle bulge here, the bulge of average TV, you know, those shows that aren't really good or really bad, they don't really get you excited. So he needs to make sure that he's really on the right end of this.
So the pressure is on, and of course it's also the first time that Amazon is even doing something like this, so Roy Price does not want to take any chances. He wants to engineer success. He needs a guaranteed success, and so what he does is, he holds a competition.
So he takes a bunch of ideas for TV shows, and from those ideas, through an evaluation, they select eight candidates for TV shows, and then he just makes the first episode of each one of these shows and puts them online for free for everyone to watch. And so when Amazon is giving out free stuff, you're going to take it, right? So millions of viewers are watching those episodes.
What they don't realize is that, while they're watching their shows, actually, they are being watched. They are being watched by Roy Price and his team, who record everything. They record when somebody presses play, when somebody presses pause, what parts they skip, what parts they watch again. So they collect millions of data points, because they want to have those data points to then decide which show they should make. And sure enough, so they collect all the data, they do all the data crunching, and an answer emerges, and the answer is, "Amazon should do a sitcom about four Republican US Senators." They did that show.
So does anyone know the name of the show? (Audience: "Alpha House.") Yes, "Alpha House," but it seems like not too many of you here remember that show, actually, because it didn't turn out that great. It's actually just an average show, actually -- literally, in fact, because the average of this curve here is at 7.4, and "Alpha House" lands at 7.5, so a slightly above average show, but certainly not what Roy Price and his team were aiming for. Meanwhile, however, at about the same time, at another company, another executive did manage to land a top show using data analysis, and his name is Ted, Ted Sarandos, who is the Chief Content Officer of Netflix, and just like Roy, he's on a constant mission to find that great TV show, and he uses data as well to do that, except he does it a little bit differently. So instead of holding a competition, what he did -- and his team of course -- was they looked at all the data they already had about Netflix viewers, you know, the ratings they give their shows, the viewing histories, what shows people like, and so on. And then they use that data to discover all of these little bits and pieces about the audience: what kinds of shows they like, what kind of producers, what kind of actors. And once they had all of these pieces together, they took a leap of faith, and they decided to license not a sitcom about four Senators but a drama series about a single Senator. You guys know the show?
(Laughter)
Yes, "House of Cards," and Netflix of course, nailed it with that show, at least for the first two seasons.
"House of Cards" gets a 9.1 rating on this curve, so it's exactly where they wanted it to be.
Now, the question of course is, what happened here? So you have two very competitive, data-savvy companies. They connect all of these millions of data points, and then it works beautifully for one of them, and it doesn't work for the other one. So why? Because logic kind of tells you that this should be working all the time. I mean, if you're collecting millions of data points on a decision you're going to make, then you should be able to make a pretty good decision. You have 200 years of statistics to rely on. You're amplifying it with very powerful computers. The least you could expect is good TV, right?
And if data analysis does not work that way, then it actually gets a little scary, because we live in a time where we're turning to data more and more to make very serious decisions that go far beyond TV. Does anyone here know the company Multi-Health Systems? No one. OK, that's good actually. OK, so Multi-Health Systems is a software company, and I hope that nobody here in this room ever comes into contact with that software, because if you do, it means you're in prison.
(Laughter)
If someone here in the US is in prison, and they apply for parole, then it's very likely that data analysis software from that company will be used in determining whether to grant that parole. So it's the same principle as Amazon and Netflix, but now instead of deciding whether a TV show is going to be good or bad, you're deciding whether a person is going to be good or bad. And mediocre TV,22 minutes, that can be pretty bad, but more years in prison, I guess, even worse.
And unfortunately, there is actually some evidence that this data analysis, despite having lots of data, does not always produce optimum results. And that's not because a company like Multi-Health Systems doesn't know what to do with data. Even the most data-savvy companies get it wrong. Yes, even Google gets it wrong sometimes.
In 2009, Google announced that they were able, with data analysis, to predict outbreaks of influenza, the nasty kind of flu, by doing data analysis on their Google searches. And it worked beautifully, and it made a big splash in the news, including the pinnacle of scientific success: a publication in the journal "Nature." It worked beautifully for year after year after year, until one year it failed. And nobody could even tell exactly why. It just didn't work that year, and of course that again made big news, including now a retraction of a publication from the journal "Nature." So even the most data-savvy companies, Amazon and Google, they sometimes get it wrong. And despite all those failures, data is moving rapidly into real-life decision-making -- into the workplace, law enforcement, medicine. So we should better make sure that data is helping.
Now, personally I've seen a lot of this struggle with data myself, because I work in computational genetics, which is also a field where lots of very smart people are using unimaginable amounts of data to make pretty serious decisions like deciding on a cancer therapy or developing a drug. And over the years, I've noticed a sort of pattern or kind of rule, if you will, about the difference between successful decision-making with data and unsuccessful decision-making, and I find this a pattern worth sharing, and it goes something like this.
So whenever you're solving a complex problem, you're doing essentially two things. The first one is, you take that problem apart into its bits and pieces so that you can deeply analyze those bits and pieces, and then of course you do the second part. You put all of these bits and pieces back together again to come to your conclusion. And sometimes you have to do it over again, but it's always those two things: taking apart and putting back together again.
And now the crucial thing is that data and data analysis is only good for the first part. Data and data analysis, no matter how powerful, can only help you taking a problem apart and understanding its pieces. It's not suited to put those pieces back together again and then to come to a conclusion. There's another tool that can do that, and we all have it, and that tool is the brain. If there's one thing a brain is good at, it's taking bits and pieces back together again, even when you have incomplete information, and coming to a good conclusion, especially if it's the brain of an expert.
And that's why I believe that Netflix was so successful, because they used data and brains where they belong in the process. They use data to first understand lots of pieces about their audience that they otherwise wouldn't have been able to understand at that depth, but then the decision to take all these bits and pieces and put them back together again and make a show like "House of Cards," that was nowhere in the data. Ted Sarandos and his team made that decision to license that show, which also meant, by the way, that they were taking a pretty big personal risk with that decision. And Amazon, on the other hand, they did it the wrong way around. They used data all the way to drive their decision-making, first when they held their competition of TV ideas, then when they selected "Alpha House" to make as a show. Which of course was a very safe decision for them, because they could always point at the data, saying, "This is what the data tells us." But it didn't lead to the exceptional results that they were hoping for.
So data is of course a massively useful tool to make better decisions, but I believe that things go wrong when data is starting to drive those decisions. No matter how powerful, data is just a tool, and to keep that in mind, I find this device here quite useful. Many of you will ...
(Laughter)
Before there was data, this was the decision-making device to use.
(Laughter)
Many of you will know this. This toy here is called the Magic 8 Ball, and it's really amazing, because if you have a decision to make, a yes or no question, all you have to do is you shake the ball, and then you get an answer -- "Most Likely" -- right here in this window in real time. I'll have it out later for tech demos.
(Laughter)
Now, the thing is, of course -- so I've made some decisions in my life where, in hindsight, I should have just listened to the ball. But, you know, of course, if you have the data available, you want to replace this with something much more sophisticated, like data analysis to come to a better decision. But that does not change the basic setup. So the ball may get smarter and smarter and smarter, but I believe it's still on us to make the decisions if we want to achieve something extraordinary, on the right end of the curve. And I find that a very encouraging message, in fact, that even in the face of huge amounts of data, it still pays off to make decisions, to be an expert in what you're doing and take risks. Because in the end, it's not data, it's risks that will land you on the right end of the curve.
Thank you.
(Applause)
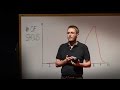
ロイ・プライスを知っている人は ほとんどいないでしょう でも彼こそ 2013年4月19日に 22分間 みなさんを 退屈させたであろう張本人です その時間が「楽しかった」と言う人も いるかもしれませんが それほど多くはないでしょう すべては その3年前に ロイが下した ある決定にさかのぼります
ロイ・プライスが役員を務めるのは アマゾン・スタジオ すなわち Amazonのテレビ制作会社です 彼は すらっとして 髪を逆立てた 47歳 Twitterの自己紹介は「映画 テレビ テクノロジーとタコス 好き」です Amazonが制作するオリジナル番組を 選ぶのが彼の仕事ですから 責任は重大です 競争も激しい業界です すでに大量のテレビ番組があって 何を選んでもいいわけではありません 本当にすごい番組を 見出さなければならないんです つまり このグラフの右端に来るような 番組を見つける必要があります
このグラフは IMDbというサイトに 掲載されている 約2,500番組の評価の分布です 評価は 1から10まで 縦軸は その評価を得た番組の数です もし 選んだ番組が9点以上の 評価を得れば 成功と言えます 上位2%に入りますから 『ブレイキング・バッド』や 『ゲーム・オブ・スローンズ』『ザ・ワイヤー』が それに当たる番組で どれもハマりやすく 1シーズン見たら 「どこで もっと見られる?」と 脳が欲してしまうような 番組です 一応 説明すると 左端には 美少女コンテスト・リアリティー番組 『Toddlers & Tiaras』が来ます
(笑)
これで グラフの左端が 何を表しているか よくわかるはずです
ただロイ・プライスは 左端のことは 心配していません 『Toddlers & Tiaras』を下回るには かなりの知恵が必要ですから だから 彼が心配するのは グラフのピーク付近です これは平均的な番組の数で 可もなく不可もなく 特に見たいとも思わない 番組なんです だから 何としてもグラフの右端に 行かなくてはなりません
プレッシャーは大きい上に Amazonが こういう事業を 手がけるのは初めてですから ロイ・プライスは 賭けに出る気はありません 絶対 成功する方法を考えます 確実に成功するために コンテストを開きます
番組の企画をたくさん集めて それぞれ評価し その中から 8つの番組を候補として選びます それから それぞれ1話を オンラインで公開し 誰でも見られるようにします Amazonが無料で配信すれば 誰だって見ますよね その結果 数百万人が 番組を見ることになります
ただ視聴者が気付いていないのは 番組を見ている間 実は自分が見られていることです ロイのチームは すべてを記録して 視聴者を観察します いつ再生し いつ一時停止したか どこを飛ばし どこをもう一度見たか 記録するんです こうして数百万の データポイントを集めます このデータポイントを使って どの番組を制作するか 決定します すべてのデータを集めて データを分析すると 答えが見えてきました その答えとは 「制作すべき番組は 4人の共和党上院議員が主役のホームコメディである」 そして制作しました
どの番組かわかりますか? (観客)『アルファ・ハウス』 そう 『アルファ・ハウス』です でも思い出せない方が多かったのは 大した番組ではなかったからです 文字通り平均点の番組です このグラフの平均は7.4ですが 『アルファ・ハウス』は7.5でしたから まさに普通というか 少しマシな程度の番組です 当然 ロイ・プライスたちの 狙いとはかけ離れています 話かわって 同じ頃 別の会社で もう一人の重役がデータ分析で ヒット番組を作ろうとしていました 彼の名前は テッド・サランドス Netflix社のコンテンツ部門代表です ロイと同じように 最高の番組を 見つけるのが仕事です 彼もデータを活用しますが 方法は少し違います 彼のチームは コンテストを開くのではなく Netflixの視聴者に関する 全データを分析しました 番組の評価や視聴履歴 どんな番組が好まれるか といったデータです そして ここから視聴者に関する こまごまとした情報を 探っていくのです 視聴者が好む番組や プロデューサー 俳優についてです そして情報をすべて組み合わせ 腹をくくって ライセンス契約を決めたのは 4人の上院議員のコメディではなく 1人の上院議員が登場する ドラマシリーズでした わかりますよね
(笑)
そう『ハウス・オブ・カード 野望の階段』で Netflixは 少なくとも2シーズンは成功しました
(笑)(拍手)
『ハウス・オブ・カード』は 9.1の評価を得ていて まさに思惑通りです
ここで当然 疑問が湧いてきます 競争力が高くデータに強い 2つの会社があり どちらも数百万のデータポイントを 組み合わせていますが 片方は とてもうまくいき もう片方は うまくいかない なぜでしょう? 論理的には常にうまくいくはずです つまり ある決定を下そうとする時に データポイントが数百万あれば かなりうまくいくはずなんです 200年の歴史を持つ統計学と 高性能のコンピュータが 力を貸してくれます 平凡な番組に終わるはずなど ないでしょう
ただ もしデータ分析が 思い通りにならなかったら 恐ろしいことです というのも テレビ以外の 様々な重要な決断を下す時 ますますデータに頼る時代に 私たちは生きているんですから Multi-Health Systems という会社を 知っている方はいますか? いませんね よかった Multi-Health Systems は ソフトウェア会社ですが ここに お世話になる人が いないといいですね もし お世話になるとすれば その人は 受刑者だからです
(笑)
アメリカで刑務所に入っている人が 仮釈放を申請すると 許可するかどうかを決めるために この会社のデータ分析ソフトが 使われる場合が多いんです AmazonやNetflixと同じ原理ですが テレビ番組の良し悪しを 決めるのではなく 1人の人間の善悪を決めるんです 22分間 退屈な番組を見るのは 苦痛かもしれませんが さらに数年 刑務所で過ごすのは ずっときついでしょう
ただ残念なことに データ分析では 大量のデータがあったとしても 常に最適な結果を出せるとは 限らないという証拠があります これはMulti-Health Systemsなどの企業が データの扱い方を知らないからではなく 極めてデータに強い企業でも誤ります そう Googleさえ 時に間違うんです
2009年 Googleは ある発表をしました 検索データを分析することで 感染力の強いインフルエンザの 流行を予測できたというのです 予測は かなりうまくいき 大きなニュースになりました 科学界 最大の栄誉である ネイチャー誌への掲載も果たしました 予測は翌年も次の年も うまくいっていましたが ある年 失敗しました 確かな理由は誰にもわかりませんでした いきなり失敗したんです もちろん これも大きなニュースになり ネイチャー誌の論文も 撤回されました AmazonやGoogleといった 極めてデータに強い企業でさえ 時に誤るんです 一方 このような失敗にも関わらず データは すごいスピードで 日常の意思決定にも 仕事の場にも 法執行機関にも 医療の現場にも 入り込んでいます だからデータが本当に 役立っているか 確認すべきです
私自身もデータとの格闘を 目の当たりにしてきました 私は計算遺伝学を研究していますが この分野でも頭の切れる人たちが 想像もつかない量のデータを使って がんの治療や 新薬の開発といった 重大な決断を下しています ここ数年 私は データを使った意思決定が 成功する場合と失敗する場合の間に ある種のパターンというか 規則性のようなものが あることに気づきました このパターンは 伝える価値があると思います
複雑な問題を解決する場合 主に2つのことをします はじめに 要素を深く分析できるように 問題を細かく分割し それから 次に進みます 要素を全部 もう一度組み合わせ 結論を引き出すんです 同じことを 繰り返す場合もありますが やることは常に この2つ 分割し 組み立て直すんです
ここで重要なのは データと その分析が有効なのは 最初の部分だけだという点です データと分析が いかに強力だろうと 役に立つのは 問題を分割して 要素を理解するところまでです 要素を組み立て直して 結論に至るには 適していないのです 私たちには 結論を引き出す 別のツールがあります それは 脳です 脳には得意なことがあります 不完全な情報しかない場合でも 要素を組み立てて 適切な結論を出すことです 特に専門家の脳は そうです
Netflixが成功した理由は データと脳を それぞれ適した場面で 利用したからでしょう まずデータを使って 視聴者に関する情報を理解しました そうしなければ そこまで 深く理解できなかったでしょう 一方で 要素を全部集めて組み立て直し 『ハウス・オブ・カード』のような データからは出てこない 番組を制作しました ゴーサインを出すと決断したのは テッド・サランドスのチームです つまり彼らは この決断によって 個人的に大きなリスクを負ったのです それに対して Amazonは方法を誤りました 意思決定の全過程でデータを使ったのです 最初に企画コンテストを開いた時も 『アルファ・ハウス』を選んで 制作した時もそうでした もちろん これは安全な決断でした だって「データから明らかだ」と 言えば済むんですから でも それでは彼らが望む 並外れた成果は上げられませんでした
確かに よりよい意思決定には データはとても役立つツールです ただ データが意思決定を 強いるようになると 問題が起きてくると思います どれほどパワフルだろうと データは単なる道具です それを意識するには この装置が役立つことに気づきました 納得する人も多いでしょう
(笑)
データが出現する前は 意思決定の手段といえば これのことでした
(笑)
知っている方も多いでしょう これは「マジック8ボール」 本当にすごい装置です もしイエスかノーの形で 何か決定しなければならない時 このボールを振るだけで 答えが出ます 「可能性は高い」 こんな風に リアルタイムで出ます 後でデモ会場に展示しましょう
(笑)
さて 肝心な点ですが これまでの私の決断には 後で考えると ボールに尋ねた方が よかったものもあります でもデータが使えるなら こんな おもちゃではなく データ分析など より洗練された手段を使って よりよく意思決定したいと 思うはずです ただ それでも 基本的な仕組みは変わりません ボールは どんどん 賢くなっていくかもしれませんが もし私たちが グラフの右端にある 何か ものすごいことを 成し遂げたいなら 今でも自分自身の決断が重要です 大量のデータを目の前にして それでもなお 自分で決定すること そして その道の専門家として リスクを負うことが 成功につながるというのは とても励みになる 教訓だと思います 結局 グラフの右端に 達するために必要なのは データではなく リスクなのです
ありがとう
(拍手)
品詞分類
- 主語
- 動詞
- 助動詞
- 準動詞
- 関係詞等
TED 日本語
TED Talks
関連動画
実証実験が示す週4日勤務制の恩恵ジュリエット・ショアー
おすすめ 12022.05.27経済的価値とは何か、そして誰がそれを生み出すのか?マリアナ・マッツカート
2020.01.10教師のこころの健康をどうすればサポートできるかシドニー・ジェンセン
2019.12.13資本主義の不都合な秘密と進むべき道ニック・ハノーアー
2019.10.18新しい言語を学ぶ秘訣 - TED Talkリディア・マホヴァ
2019.01.24人口が100億人に達する地球でどう生き延びるか?チャールズ・C・マン
2018.11.16漫画は教室にふさわしい | TED Talkジーン・ヤン
2018.06.15健全な経済は成長ではなく繁栄を目指しデザインされるべき | TED Talkケイト・ラワース
2018.06.04データで見ると、世界は良くなっているのか、悪くなっているのか?スティーブン・ピンカー
おすすめ 12018.05.21言語はいかに我々の考えを形作るのかレラ・ボロディツキー
2018.05.02公的資金による学術研究の成果を自由に見られないのはなぜか?エリカ・ストーン
2018.04.19子ども達が生涯の読書家になるためにアルヴィン・アービー
2018.04.04人間の感情の歴史ティファニー・ワット・スミス
2018.01.31世帯収入ごとの世界の暮らしを覗いてみようアンナ・ロスリング・ロンランド
おすすめ 12018.01.18職が無くなる未来社会でのお金の稼ぎ方マーティン・フォード
おすすめ 12017.11.16多様な考え方が持つ革命的な力エリフ・シャファク
2017.10.27
洋楽 おすすめ
RECOMMENDS
洋楽歌詞
ステイザ・キッド・ラロイ、ジャスティン・ビーバー
洋楽最新ヒット2021.08.20スピーチレス~心の声ナオミ・スコット
洋楽最新ヒット2019.05.23シェイプ・オブ・ユーエド・シーラン
洋楽人気動画2017.01.30フェイデッドアラン・ウォーカー
洋楽人気動画2015.12.03ウェイティング・フォー・ラヴアヴィーチー
洋楽人気動画2015.06.26シー・ユー・アゲインウィズ・カリファ
洋楽人気動画2015.04.06シュガーマルーン5
洋楽人気動画2015.01.14シェイク・イット・オフテイラー・スウィフト
ポップス2014.08.18オール・アバウト・ザット・ベースメーガン・トレイナー
ポップス2014.06.11ストーリー・オブ・マイ・ライフワン・ダイレクション
洋楽人気動画2013.11.03コール・ミー・メイビーカーリー・レイ・ジェプセン
洋楽人気動画2012.03.01美しき生命コールドプレイ
洋楽人気動画2008.08.04バッド・デイ~ついてない日の応援歌ダニエル・パウター
洋楽人気動画2008.05.14サウザンド・マイルズヴァネッサ・カールトン
洋楽人気動画2008.02.19イッツ・マイ・ライフボン・ジョヴィ
ロック2007.10.11アイ・ウォント・イット・ザット・ウェイバックストリート・ボーイズ
洋楽人気動画2007.09.14マイ・ハート・ウィル・ゴー・オンセリーヌ・ディオン
洋楽人気動画2007.07.12ヒーローマライア・キャリー
洋楽人気動画2007.03.21オールウェイズ・ラヴ・ユーホイットニー・ヒューストン
洋楽人気動画2007.02.19オネスティビリー・ジョエル
洋楽人気動画2005.09.16