
TED日本語
TED Talks(英語 日本語字幕付き動画)
TED日本語 - ジャレド・フィックリン: 新しい音楽の見かた(色で見る! 炎で見る!)
TED Talks
新しい音楽の見かた(色で見る! 炎で見る!)
New ways to see music (with color! and fire!)
ジャレド・フィックリン
Jared Ficklin
内容
デザイナーのジャレド・フィックリンが、音楽を鮮烈に視覚化します。色による表現、さらにはTEDステージに初めて火を持ち込んで、音が私達にどのような感覚を与えるのかを明らかにします。さらにそこからスケートボード場の音の分析に進み、音が私達の創造性をどう高めるかを分析します。 ジャレド・フィックリンはフロッグ・デザインでユーザーインターフェースを設計しています。趣味として、光や形や火による音楽の表現を追求しています。
字幕
SCRIPT
Script
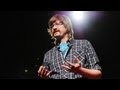
My passions are music, technology and making things. And it's the combination of these things that has led me to the hobby of sound visualization, and, on occasion, has led me to play with fire.
This is a Rubens' tube. It's one of many I've made over the years, and I have one here tonight. It's about an 8-foot-long tube of metal, it's got a hundred or so holes on top, on that side is the speaker, and here is some lab tubing, and it's connected to this tank of propane. So, let's fire it up and see what it does. So let's play a 550-herz frequency and watch what happens.
Thank you. (Applause) It's okay to applaud the laws of physics, but essentially what's happening here -- (Laughter) -- is the energy from the sound via the air and gas molecules is influencing the combustion properties of propane, creating a visible waveform, and we can see the alternating regions of compression and rarefaction that we call frequency, and the height is showing us amplitude. So let's change the frequency of the sound, and watch what happens to the fire.
So every time we hit a resonant frequency we get a standing wave and that emergent sine curve of fire. So let's turn that off. We're indoors. Thank you. (Applause)
I also have with me a flame table. It's very similar to a Rubens' tube, and it's also used for visualizing the physical properties of sound, such as eigenmodes, so let's fire it up and see what it does.
Ooh. (Laughter) Okay. Now, while the table comes up to pressure, let me note here that the sound is not traveling in perfect lines. It's actually traveling in all directions, and the Rubens' tube's a little like bisecting those waves with a line, and the flame table's a little like bisecting those waves with a plane, and it can show a little more subtle complexity, which is why I like to use it to watch Geoff Farina play guitar.
(Music)
All right, so it's a delicate dance. If you watch closely - (Applause) If you watch closely, you may have seen some of the eigenmodes, but also you may have seen that jazz music is better with fire. Actually, a lot of things are better with fire in my world, but the fire's just a foundation. It shows very well that eyes can hear, and this is interesting to me because technology allows us to present sound to the eyes in ways that accentuate the strength of the eyes for seeing sound, such as the removal of time.
So here, I'm using a rendering algorithm to paint the frequencies of the song "Smells Like Teen Spirit" in a way that the eyes can take them in as a single visual impression, and the technique will also show the strengths of the visual cortex for pattern recognition. So if I show you another song off this album, and another, your eyes will easily pick out the use of repetition by the band Nirvana, and in the frequency distribution, the colors, you can see the clean-dirty-clean sound that they are famous for, and here is the entire album as a single visual impression, and I think this impression is pretty powerful.
At least, it's powerful enough that if I show you these four songs, and I remind you that this is "Smells Like Teen Spirit," you can probably correctly guess, without listening to any music at all, that the song a die hard Nirvana fan would enjoy is this song, "I'll Stick Around" by the Foo Fighters, whose lead singer is Dave Grohl, who was the drummer in Nirvana. The songs are a little similar, but mostly I'm just interested in the idea that someday maybe we'll buy a song because we like the way it looks.
All right, now for some more sound data. This is data from a skate park, and this is Mabel Davis skate park in Austin, Texas. (Skateboard sounds) And the sounds you're hearing came from eight microphones attached to obstacles around the park, and it sounds like chaos, but actually all the tricks start with a very distinct slap, but successful tricks end with a pop, whereas unsuccessful tricks more of a scratch and a tumble, and tricks on the rail will ring out like a gong, and voices occupy very unique frequencies in the skate park.
So if we were to render these sounds visually, we might end up with something like this. This is all 40 minutes of the recording, and right away the algorithm tells us a lot more tricks are missed than are made, and also a trick on the rails is a lot more likely to produce a cheer, and if you look really closely, we can tease out traffic patterns. You see the skaters often trick in this direction. The obstacles are easier.
And in the middle of the recording, the mics pick this up, but later in the recording, this kid shows up, and he starts using a line at the top of the park to do some very advanced tricks on something called the tall rail. And it's fascinating. At this moment in time, all the rest of the skaters turn their lines 90 degrees to stay out of his way. You see, there's a subtle etiquette in the skate park, and it's led by key influencers, and they tend to be the kids who can do the best tricks, or wear red pants, and on this day the mics picked that up.
All right, from skate physics to theoretical physics. I'm a big fan of Stephen Hawking, and I wanted to use all eight hours of his Cambridge lecture series to create an homage. Now, in this series he's speaking with the aid of a computer, which actually makes identifying the ends of sentences fairly easy. So I wrote a steering algorithm. It listens to the lecture, and then it uses the amplitude of each word to move a point on the x-axis, and it uses the inflection of sentences to move a same point up and down on the y-axis.
And these trend lines, you can see, there's more questions than answers in the laws of physics, and when we reach the end of a sentence, we place a star at that location. So there's a lot of sentences, so a lot of stars, and after rendering all of the audio, this is what we get. This is Stephen Hawking's universe.
(Applause)
It's all eight hours of the Cambridge lecture series taken in as a single visual impression, and I really like this image, but a lot of people think it's fake. So I made a more interactive version, and the way I did that is I used their position in time in the lecture to place these stars into 3D space, and with some custom software and a Kinect, I can walk right into the lecture. I'm going to wave through the Kinect here and take control, and now I'm going to reach out and I'm going to touch a star, and when I do, it will play the sentence that generated that star.
Stephen Hawking: There is one, and only one, arrangement in which the pieces make a complete picture.
Jared Ficklin: Thank you. (Applause) There are 1,400 stars. It's a really fun way to explore the lecture, and, I hope, a fitting homage.
All right. Let me close with a work in progress. I think, after 30 years, the opportunity exists to create an enhanced version of closed captioning. Now, we've all seen a lot of TEDTalks online, so let's watch one now with the sound turned off and the closed captioning turned on.
There's no closed captioning for the TED theme song, and we're missing it, but if you've watched enough of these, you hear it in your mind's ear, and then applause starts. It usually begins here, and it grows and then it falls. Sometimes you get a little star applause, and then I think even Bill Gates takes a nervous breath, and the talk begins.
All right, so let's watch this clip again. This time, I'm not going to talk at all. There's still going to be no audio, but what I am going to do is I'm going to render the sound visually in real time at the bottom of the screen. So watch closely and see what your eyes can hear.
This is fairly amazing to me. Even on the first view, your eyes will successfully pick out patterns, but on repeated views, your brain actually gets better at turning these patterns into information. You can get the tone and the timbre and the pace of the speech, things that you can't get out of closed captioning. That famous scene in horror movies where someone is walking up from behind is something you can see, and I believe this information would be something that is useful at times when the audio is turned off or not heard at all, and I speculate that deaf audiences might actually even be better at seeing sound than hearing audiences. I don't know. It's a theory right now. Actually, it's all just an idea.
And let me end by saying that sound moves in all directions, and so do ideas. Thank you. (Applause)
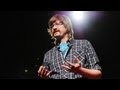
私が 情熱を注いでいるのは音楽とテクノロジーと物作りです これらを組み合わせて 音を視覚化することが趣味になりました 時には 火も扱います
これはルーベンスチューブです 私が作った物の一つです ご覧ください 2.4mほどの金属管で約100個の穴があり ― 片側はスピーカ反対はタンクへの配管です プロパンガスです それでは火をつけてみましょう 次に周波数550Hzの音を流します ご覧ください
(音)
ありがとう (拍手) 物理法則に拍手するのはいいとしてここで起きたことは ― (笑) 音のエネルギーが 空気とガスの分子を通して プロパンの燃焼性に影響を与え 波形を作り出します 圧力の高い部分と低い部分が 交互に ― つまり周波数が見えます 高さは振幅を表します それでは音の周波数を変えて 炎がどう変化するか見てみましょう
(周波数を上げる)
共振周波数になると定在波が生じて 炎のサイン曲線が表れます 室内なので もう消しましょう ありがとう (拍手)
「フレイムテーブル」も持ってきました ルーベンスチューブと同様に 音の物理特性を視覚化できます たとえば固有モードです 火を点けて どうなるか見てみましょう
おっ (笑) テーブルの圧力が上がる間に指摘しておきたいのは 音は直線状に進むのではなく あらゆる方向に進むということです ルーベンスチューブが音波を線状に切り出すのに対して フレイムテーブルは音波を 平面的に切り出すと言えます 複雑さを細かく表せるのでジェフ・ファリーナのギター演奏を 見るにはうってつけです
(音楽)
繊細なダンスのようです よく見ると ― (拍手) 固有モードが見えたかも知れません 炎とジャズは 相性がいいことにも気づくでしょう 火と相性がいいものはたくさんあります ただ 火は土台に過ぎません 目でも聞けることを 火が示しているのです 私が面白いと思うのはテクノロジーを使って 目の特性を生かしながら音を見えるようにできること ― たとえば時間を無視できることです
私はレンダリングアルゴリズムを使って ニルヴァーナの曲「スメルズ・ライク・ティーン・スピリット」の 周波数を 目で見えるように1つのイメージにしました この技術を使うと大脳視覚野が パターン認識に優れていることもわかります 同じアルバムの別の曲を見るとニルヴァーナが反復を用いていることが よくわかります 周波数の分布 つまり色から このバンドの特長である清-濁-清 という音のパターンがわかります これはアルバム全体を1つのイメージにしたものです とても力強いイメージです
どのくらい力強いかというと 次の4曲を見て ― ちなみにこれが「スメルズ・ライク・ティーン・スピリット」ですが ― 音を聞かなくても おおむね正しく 筋金入りのニルヴァーナ・ファンが気に入りそうなのはこの曲 ― フー・ファイターズの「アイル・スティック・アラウンド」だと言える程です リード・シンガーのデイヴ・グロールは ニルヴァーナのドラマーをしていました 2曲は確かに似ていますが もっぱら私が関心があるのは 曲を見た目で買う日が来るかもしれないという発想です
さらに音のデータを見ましょう これはスケートボード場で録音しました テキサス州オースチンにある ― メイベル・デイビス・スケートパークです(スケートボードの音) 今 聞こえる音は 障害物に取り付けた8つのマイクで録りました 雑音のようですが 実際は どの技も特徴的な音で始まり ― 成功するとポンという音 ― 失敗すると こすれる音や 転がる音で終わります レールに乗る技はゴングのように響き 人の声は特徴的な周波数を占めます
だから ここの音を視覚化したら こんな感じになります これが40分にわたる録音の全てです このアルゴリズムでわかることは 技の多くは失敗し レールを使った技は 歓声を伴うことが多いということですさらに細かく見ると 移動パターンを知ることができます スケーターはこの方向に滑りますこの方が障害が簡単だからです
これは録音中盤でマイクがとらえました ところが後半で少年が現れ ― 上にあるコースを使いトール・レールの上で とても難しい技を始めました 興味深いことに この瞬間 ― 他のスケーター全員がコースの方向を90度変えて彼に場所を譲ったのです パークには細かい作法があり 影響力をもつ人がいます そういう人は 腕がすごいとか赤いパンツ姿と相場は決まっています この日マイクが捉えたのはそんな作法です
次はスケートボードの物理から理論物理学へ移りましょう 私はスティーブン・ホーキングの大ファンで ケンブリッジでの講義8時間分を使ってオマージュを制作しようと考えました この連続講義で ホーキング氏はコンピュータを使って話しています だから文の終わりを特定するのは簡単です そこで私はステアリングアルゴリズムを作りました これで講義を取り込み ― 個々の語の振幅を用いてX軸上の点を動かします さらに文の音調の変化をとらえて その点をY軸にそって上下させます
この傾向線を見ると 物理法則について 解答より 疑問の方が多いことがわかります 文末に達すると そこに星を付けます 文が多いので たくさん星があります 音声を全て表示すると こうなります これがスティーブン・ホーキングの宇宙です
(拍手)
8時間に渡るケンブリッジでの講義を 一枚のイメージにしたものです 私はこれを大変気に入っていますが いい加減だと考える人が多いので インタラクティブ版を作りました 講義における時間的な位置を使って 三次元空間に 星を配置し 自作のソフトとKinectで 講義の中に入ることができるようにしました Kinectに向けて手を振り 操作します 手を伸ばして星に触れると その星を生成した元の文が再生できます
「各部分が完全な像を構成するような 唯一の配置があります」
ありがとう (拍手) ここには1,400個の星があります 講義を楽しく探究できます オマージュにふさわしいでしょうか
最後に制作中の作品をご覧ください クローズド・キャプションの登場から30年 ― 強化版を作る機会を得ました みんなTEDトークをオンラインで見ますが 音を消して クローズド・キャプションを ― ONにしたものを見てみましょう
TEDのテーマ曲には字幕がありません 字幕がなくても たくさん見ていれば 頭の中で テーマと拍手が聞こえるでしょう ここで始まり 盛り上がって消えます 拍手が特に大きい時もあります ビル・ゲイツですら不安そうに息をしてから トークが始まります
もう一度ビデオを見ましょう 今度は私はしゃべりません 音声はありませんが 音を視覚的に描画して 画面下部にリアルタイムで表示します 皆さんの目は何を「聞く」ことができるでしょうか
すごいことだと思います 1度見ただけでもパターンが見つけられますが 何度か見ると 皆さんの脳は パターンを情報に置き換えることに慣れ 音調や音質 話すペースがわかるようになります 字幕からはとらえられないものです ホラー映画でありがちな ― 何者かが後ろから迫ってくる場面も 見えるようになります これらの情報は 音声がオフの場合や聞こえない場合に役立つでしょう また耳が聞こえない人は聞こえる人に比べて 音を見ることに 長けていると思うのです まだ仮説で はっきりわかりません 単なるアイデアです
最後になりますが ―音はあらゆる方向に広がります アイデアも同じです ありがとうございます (拍手)
品詞分類
- 主語
- 動詞
- 助動詞
- 準動詞
- 関係詞等
TED 日本語
TED Talks
関連動画
十代の科学者が考えた、傷の治癒を促す発明アヌシュカ・ナイクナーレ
2018.06.19自分の声が嫌だと感じるのはなぜかレベッカ・クラインバーガー
2018.05.24若い発明家の発泡スチロールリサイクル計画アシュトン・コーファー
2017.04.18においの「自撮り」やその他の合成生物学的な実験アニー・リュー
2017.03.17物理学の未解決問題をいかに探求するかジェームズ・ビーチャム
2017.01.20世界を理解する奥義としての数学ロジャー・アントンセン
2016.12.13機械に奪われる仕事 ― そして残る仕事アンソニー・ゴールドブルーム
2016.08.31次なる科学の大発見とは?エリック・ヘイゼルティン
2016.08.18数学の何がそれほど魅惑的なのかセドリック・ヴィラニ
2016.06.28飛行機内の病原菌の動き方と対処法レイモンド・ワン
2016.01.11科学を良い方向に進める知られざる頭脳ジャダイダ・アイスラー
2016.01.07量子生物学は生命の最大の謎を解明するか?ジム・アルカリリ
2015.09.16バスケットボールの激しい動きの背後にある数学ラジブ・マヒシュワラン
2015.07.06笑わせ、そして考えさせる科学賞マーク・エイブラハムズ
2014.10.24科学者を信頼すべき理由ナオミ・オレスケス
2014.06.25知能の方程式アレックス・ウィスナー=グロス
2014.02.06
洋楽 おすすめ
RECOMMENDS
洋楽歌詞
ダイナマイトビーティーエス
洋楽最新ヒット2020.08.20ディス・イズ・ミーグレイテスト・ショーマン・キャスト
洋楽人気動画2018.01.11グッド・ライフGイージー、ケラーニ
洋楽人気動画2017.01.27ホワット・ドゥ・ユー・ミーン?ジャスティン・ビーバー
洋楽人気動画2015.08.28ファイト・ソングレイチェル・プラッテン
洋楽人気動画2015.05.19ラヴ・ミー・ライク・ユー・ドゥエリー・ゴールディング
洋楽人気動画2015.01.22アップタウン・ファンクブルーノ・マーズ、マーク・ロンソン
洋楽人気動画2014.11.20ブレイク・フリーアリアナ・グランデ
洋楽人気動画2014.08.12ハッピーファレル・ウィリアムス
ポップス2014.01.08カウンティング・スターズワンリパブリック
ロック2013.05.31ア・サウザンド・イヤーズクリスティーナ・ペリー
洋楽人気動画2011.10.26ユー・レイズ・ミー・アップケルティック・ウーマン
洋楽人気動画2008.05.30ルーズ・ユアセルフエミネム
洋楽人気動画2008.02.21ドント・ノー・ホワイノラ・ジョーンズ
洋楽人気動画2008.02.15オンリー・タイムエンヤ
洋楽人気動画2007.10.03ミス・ア・シングエアロスミス
ロック2007.08.18タイム・トゥ・セイ・グッバイサラ・ブライトマン
洋楽人気動画2007.06.08シェイプ・オブ・マイ・ハートスティング
洋楽人気動画2007.03.18ウィ・アー・ザ・ワールド(U.S.A. フォー・アフリカ)マイケル・ジャクソン
洋楽人気動画2006.05.14ホテル・カリフォルニアイーグルス
ロック2005.07.06