
TED日本語
TED Talks(英語 日本語字幕付き動画)
TED日本語 - アンソニー・ゴールドブルーム: 機械に奪われる仕事 ― そして残る仕事
TED Talks
機械に奪われる仕事 ― そして残る仕事
The jobs we'll lose to machines -- and the ones we won't
アンソニー・ゴールドブルーム
Anthony Goldbloom
内容
機械学習は、もはや信用リスク評価や、手紙の仕分けといった単純な仕事だけをこなすわけではありません。今では小論文の採点や病気の診断といった、ずっと複雑なこともできるようになっています。このような進歩は不安を覚える疑問を提起します ― 将来私たちの仕事はロボットに取られてしまうのでしょうか?
字幕
SCRIPT
Script
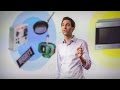
So this is my niece. Her name is Yahli. She is nine months old. Her mum is a doctor, and her dad is a lawyer. By the time Yahli goes to college, the jobs her parents do are going to look dramatically different.
In 2013, researchers at Oxford University did a study on the future of work. They concluded that almost one in every two jobs have a high risk of being automated by machines. Machine learning is the technology that's responsible for most of this disruption. It's the most powerful branch of artificial intelligence. It allows machines to learn from data and mimic some of the things that humans can do. My company, Kaggle, operates on the cutting edge of machine learning. We bring together hundreds of thousands of experts to solve important problems for industry and academia. This gives us a unique perspective on what machines can do, what they can't do and what jobs they might automate or threaten.
Machine learning started making its way into industry in the early '90s. It started with relatively simple tasks. It started with things like assessing credit risk from loan applications, sorting the mail by reading handwritten characters from zip codes. Over the past few years, we have made dramatic breakthroughs. Machine learning is now capable of far, far more complex tasks. In 2012, Kaggle challenged its community to build an algorithm that could grade high-school essays. The winning algorithms were able to match the grades given by human teachers. Last year, we issued an even more difficult challenge. Can you take images of the eye and diagnose an eye disease called diabetic retinopathy? Again, the winning algorithms were able to match the diagnoses given by human ophthalmologists.
Now, given the right data, machines are going to outperform humans at tasks like this. A teacher might read 10,000 essays over a 40-year career. An ophthalmologist might see 50,000 eyes. A machine can read millions of essays or see millions of eyes within minutes. We have no chance of competing against machines on frequent, high-volume tasks.
But there are things we can do that machines can't do. Where machines have made very little progress is in tackling novel situations. They can't handle things they haven't seen many times before. The fundamental limitations of machine learning is that it needs to learn from large volumes of past data. Now, humans don't. We have the ability to connect seemingly disparate threads to solve problems we've never seen before.
Percy Spencer was a physicist working on radar during World War II, when he noticed the magnetron was melting his chocolate bar. He was able to connect his understanding of electromagnetic radiation with his knowledge of cooking in order to invent -- any guesses? -- the microwave oven.
Now, this is a particularly remarkable example of creativity. But this sort of cross-pollination happens for each of us in small ways thousands of times per day. Machines can not compete with us when it comes to tackling novel situations, and this puts a fundamental limit on the human tasks that machines will automate.
So what does this mean for the future of work? The future state of any single job lies in the answer to a single question: To what extent is that job reducible to frequent, high-volume tasks, and to what extent does it involve tackling novel situations? On frequent, high-volume tasks, machines are getting smarter and smarter. Today they grade essays. They diagnose certain diseases. Over coming years, they're going to conduct our audits, and they're going to read boilerplate from legal contracts. Accountants and lawyers are still needed. They're going to be needed for complex tax structuring, for pathbreaking litigation. But machines will shrink their ranks and make these jobs harder to come by.
Now, as mentioned, machines are not making progress on novel situations. The copy behind a marketing campaign needs to grab consumers' attention. It has to stand out from the crowd. Business strategy means finding gaps in the market, things that nobody else is doing. It will be humans that are creating the copy behind our marketing campaigns, and it will be humans that are developing our business strategy.
So Yahli, whatever you decide to do, let every day bring you a new challenge. If it does, then you will stay ahead of the machines.
Thank you.
(Applause)
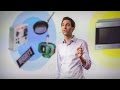
この子は私の姪で 名前をヤーリといいます 生まれて9カ月です 母親は医者で 父親は弁護士ですが ヤーリが大学に行く頃には 両親の仕事の様子は 劇的に変わっていることでしょう
2013年オックスフォード大学の研究者達が 未来の仕事についての研究を行いました 職の2つに1つは 機械により自動化されるリスクが高いと 彼らは結論付けました 機械学習の技術こそ そのような変化の 主な原因となるものです これは人工知能分野の中でも 最も有力な領域です この技術により 機械がデータから学習して ある種のことを 人間のようにできるようになります 私の会社Kaggleでは 最先端の機械学習技術に取り組んでいて 産業や学問上の重要な問題を 解決するために 何十万というエキスパートを 集めています そのお陰で 独特な知見が得られます 機械には何ができ 何ができないのか? どんな仕事に 自動化や 消失の怖れがあるのか?
機械学習が産業界で使われ出したのは 1990年代前半です まずは比較的単純な タスクから始まりました ローン申し込みに対する 信用リスクの評価や 手書きの郵便番号を読み取って 手紙を仕分けるといったことです ここ数年の間に 飛躍的な進歩がありました 機械学習が はるかに複雑なタスクを こなせるようになったのです 2012年 Kaggleは 高校生の書いた小論文を採点できる アルゴリズムを作るという課題を 専門家コミュニティに提示しました 優勝したアルゴリズムは 人間の教師の採点と 一致する評価を することができました 昨年には さらに難しい 課題を出しました 「眼球の写真から 糖尿病性網膜症の診断をできるか?」 というものです この時も 優勝した アルゴリズムは 人間の眼科医の診断と 一致する結果を出せました
適切なデータが与えられれば このようなタスクで 機械は人間より優れた結果を 出し始めています 教師は40年の経歴において 小論文を1万本読むかもしれません 眼科医は眼を5万個 診断するかもしれません しかし 機械なら数分のうちに 数百万の小論文を読み 数百万の眼を診ることができます 頻度が高く 多量のデータを 処理するタスクでは 人間が機械に勝てる見込みはありません
しかし 我々に出来て 機械に出来ないことがあります 機械の技術が ほとんど進歩していないのは 経験のない状況で 判断する技術です 機械は前にほとんど見たことがない状況を うまく処理できないのです 機械学習に根本的な限界があるのは 大量の過去データから 学ぶ必要があるという点です 人間は違います 我々は ほとんど共通点のない 手掛かりを繋ぎ合わせ 見たことのない問題を 解決することができます
パーシー・スペンサーは第2次世界大戦中 レーダー開発の任務に就いていた物理学者で その時 マグネトロンがチョコバーを 溶かすことに気づきました 電磁波に関する理解と 料理に関する知識を結びつけることで 彼の発明したのが ― 何か分かりますか? 電子レンジです
これこそ創造力の 素晴らしい一例です このような分野を超えた発想は 些細な形であれば 誰にでも毎日何千回と ひらめいています 経験のない状況においては 機械は人間には勝てず それが人間の行うことを 機械で自動化する際の 基本的な限界を与えます
これが将来の仕事に 意味することは何でしょう? 各々の仕事の未来の運命は ある1つの問への答えにかかっています 高頻度多量データ処理に 還元できる部分がどの程度あり 前例無き状況への対応を求められる部分が どの程度あるのか? 高頻度多量データ処理については 機械はどんどん賢くなっていきます 今では 機械が小論文の採点をし ある種の病気の診断をします 数年内には 監査をしたり 法律上の契約書から一般的な表現を 解釈出来るようになるでしょう それでも会計士や弁護士が いらなくはなりません 複雑な税務対策や 前例のない訴訟の対応には 必要とされるのです 機械により 能力のある者だけが残され これらの職に就くことは 難しくなります
さて 前にも述べましたが 経験のない状況に対応する技術は 進歩していません マーケティング活動における宣伝文句は 消費者の関心を引く必要があります 数ある中で 目立っていなければなりません ビジネス戦略とは 他社がやっていない 市場のニッチを探り出すことです マーケティング活動における宣伝文句を 創り出すのは人間の役目であり ビジネス戦略を考え出すのも 人間です
だからね ヤーリちゃん どんな仕事を選ぶにせよ 常日頃 新しいことに挑戦することです そうすれば 機械に先んじることが出来るでしょう
ありがとうございました
(拍手)
品詞分類
- 主語
- 動詞
- 助動詞
- 準動詞
- 関係詞等
TED 日本語
TED Talks
関連動画
十代の科学者が考えた、傷の治癒を促す発明アヌシュカ・ナイクナーレ
2018.06.19自分の声が嫌だと感じるのはなぜかレベッカ・クラインバーガー
2018.05.24若い発明家の発泡スチロールリサイクル計画アシュトン・コーファー
2017.04.18においの「自撮り」やその他の合成生物学的な実験アニー・リュー
2017.03.17物理学の未解決問題をいかに探求するかジェームズ・ビーチャム
2017.01.20世界を理解する奥義としての数学ロジャー・アントンセン
2016.12.13次なる科学の大発見とは?エリック・ヘイゼルティン
2016.08.18数学の何がそれほど魅惑的なのかセドリック・ヴィラニ
2016.06.28飛行機内の病原菌の動き方と対処法レイモンド・ワン
2016.01.11科学を良い方向に進める知られざる頭脳ジャダイダ・アイスラー
2016.01.07量子生物学は生命の最大の謎を解明するか?ジム・アルカリリ
2015.09.16バスケットボールの激しい動きの背後にある数学ラジブ・マヒシュワラン
2015.07.06笑わせ、そして考えさせる科学賞マーク・エイブラハムズ
2014.10.24科学者を信頼すべき理由ナオミ・オレスケス
2014.06.25知能の方程式アレックス・ウィスナー=グロス
2014.02.06子犬たち!さあ、複合性理論だよ!ニコラ・ペロニー
2014.01.30
洋楽 おすすめ
RECOMMENDS
洋楽歌詞
ダイナマイトビーティーエス
洋楽最新ヒット2020.08.20ディス・イズ・ミーグレイテスト・ショーマン・キャスト
洋楽人気動画2018.01.11グッド・ライフGイージー、ケラーニ
洋楽人気動画2017.01.27ホワット・ドゥ・ユー・ミーン?ジャスティン・ビーバー
洋楽人気動画2015.08.28ファイト・ソングレイチェル・プラッテン
洋楽人気動画2015.05.19ラヴ・ミー・ライク・ユー・ドゥエリー・ゴールディング
洋楽人気動画2015.01.22アップタウン・ファンクブルーノ・マーズ、マーク・ロンソン
洋楽人気動画2014.11.20ブレイク・フリーアリアナ・グランデ
洋楽人気動画2014.08.12ハッピーファレル・ウィリアムス
ポップス2014.01.08カウンティング・スターズワンリパブリック
ロック2013.05.31ア・サウザンド・イヤーズクリスティーナ・ペリー
洋楽人気動画2011.10.26ユー・レイズ・ミー・アップケルティック・ウーマン
洋楽人気動画2008.05.30ルーズ・ユアセルフエミネム
洋楽人気動画2008.02.21ドント・ノー・ホワイノラ・ジョーンズ
洋楽人気動画2008.02.15オンリー・タイムエンヤ
洋楽人気動画2007.10.03ミス・ア・シングエアロスミス
ロック2007.08.18タイム・トゥ・セイ・グッバイサラ・ブライトマン
洋楽人気動画2007.06.08シェイプ・オブ・マイ・ハートスティング
洋楽人気動画2007.03.18ウィ・アー・ザ・ワールド(U.S.A. フォー・アフリカ)マイケル・ジャクソン
洋楽人気動画2006.05.14ホテル・カリフォルニアイーグルス
ロック2005.07.06