
TED日本語
TED Talks(英語 日本語字幕付き動画)
TED日本語 - ニコラス・クリスタキス: いかに社会的ネットワークが流行を予想するか
TED Talks
いかに社会的ネットワークが流行を予想するか
How social networks predict epidemics
ニコラス・クリスタキス
Nicholas Christakis
内容
人々の複雑な社会的ネットワークを可視化したニコラス・クリスタキスとジェームス・フォウラーは、この情報をもとにして生活の改善をする方法の研究に着手しました。ここではクリスタキスが、革新的なアイデアやリスクのある行動、新型インフルエンザのようなウイルス等の流行は、社会的ネットワークを利用することによって従来より早期に感知できるという最新の研究結果を紹介します。
字幕
SCRIPT
Script
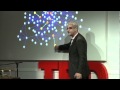
For the last 10 years, I've been spending my time trying to figure out how and why human beings assemble themselves into social networks. And the kind of social network I'm talking about is not the recent online variety, but rather, the kind of social networks that human beings have been assembling for hundreds of thousands of years, ever since we emerged from the African savannah. So, I form friendships and co-worker and sibling and relative relationships with other people who in turn have similar relationships with other people. And this spreads on out endlessly into a distance. And you get a network that looks like this. Every dot is a person. Every line between them is a relationship between two people -- different kinds of relationships. And you can get this kind of vast fabric of humanity, in which we're all embedded.
And my colleague, James Fowler and I have been studying for quite sometime what are the mathematical, social, biological and psychological rules that govern how these networks are assembled and what are the similar rules that govern how they operate, how they affect our lives. But recently, we've been wondering whether it might be possible to take advantage of this insight, to actually find ways to improve the world, to do something better, to actually fix things, not just understand things. So one of the first things we thought we would tackle would be how we go about predicting epidemics.
And the current state of the art in predicting an epidemic -- if you're the CDC or some other national body -- is to sit in the middle where you are and collect data from physicians and laboratories in the field that report the prevalence or the incidence of certain conditions. So, so and so patients have been diagnosed with something, or other patients have been diagnosed, and all these data are fed into a central repository, with some delay. And if everything goes smoothly,one to two weeks from now you'll know where the epidemic was today. And actually, about a year or so ago, there was this promulgation of the idea of Google Flu Trends, with respect to the flu, where by looking at people's searching behavior today, we could know where the flu -- what the status of the epidemic was today, what's the prevalence of the epidemic today.
But what I'd like to show you today is a means by which we might get not just rapid warning about an epidemic, but also actually early detection of an epidemic. And, in fact, this idea can be used not just to predict epidemics of germs, but also to predict epidemics of all sorts of kinds. For example, anything that spreads by a form of social contagion could be understood in this way, from abstract ideas on the left like patriotism, or altruism, or religion to practices like dieting behavior, or book purchasing, or drinking, or bicycle-helmet [ and ] other safety practices, or products that people might buy, purchases of electronic goods, anything in which there's kind of an interpersonal spread. A kind of a diffusion of innovation could be understood and predicted by the mechanism I'm going to show you now.
So, as all of you probably know, the classic way of thinking about this is the diffusion-of-innovation, or the adoption curve. So here on the Y-axis, we have the percent of the people affected, and on the X-axis, we have time. And at the very beginning, not too many people are affected, and you get this classic sigmoidal, or S-shaped, curve. And the reason for this shape is that at the very beginning, let's say one or two people are infected, or affected by the thing and then they affect, or infect,two people, who in turn affect four,eight,16 and so forth, and you get the epidemic growth phase of the curve. And eventually, you saturate the population. There are fewer and fewer people who are still available that you might infect, and then you get the plateau of the curve, and you get this classic sigmoidal curve. And this holds for germs, ideas, product adoption, behaviors, and the like. But things don't just diffuse in human populations at random. They actually diffuse through networks. Because, as I said, we live our lives in networks, and these networks have a particular kind of a structure.
Now if you look at a network like this -- this is 105 people. And the lines represent -- the dots are the people, and the lines represent friendship relationships. You might see that people occupy different locations within the network. And there are different kinds of relationships between the people. You could have friendship relationships, sibling relationships, spousal relationships, co-worker relationships, neighbor relationships and the like. And different sorts of things spread across different sorts of ties. For instance, sexually transmitted diseases will spread across sexual ties. Or, for instance, people's smoking behavior might be influenced by their friends. Or their altruistic or their charitable giving behavior might be influenced by their coworkers, or by their neighbors. But not all positions in the network are the same.
So if you look at this, you might immediately grasp that different people have different numbers of connections. Some people have one connection, some have two, some have six, some have 10 connections. And this is called the "degree" of a node, or the number of connections that a node has. But in addition, there's something else. So, if you look at nodes A and B, they both have six connections. But if you can see this image [ of the network ] from a bird's eye view, you can appreciate that there's something very different about nodes A and B. So, let me ask you this -- I can cultivate this intuition by asking a question -- who would you rather be if a deadly germ was spreading through the network, A or B? (Audience: B.) Nicholas Christakis: B, it's obvious. B is located on the edge of the network. Now, who would you rather be if a juicy piece of gossip were spreading through the network? A. And you have an immediate appreciation that A is going to be more likely to get the thing that's spreading and to get it sooner by virtue of their structural location within the network. A, in fact, is more central, and this can be formalized mathematically. So, if we want to track something that was spreading through a network, what we ideally would like to do is to set up sensors on the central individuals within the network, including node A, monitor those people that are right there in the middle of the network, and somehow get an early detection of whatever it is that is spreading through the network.
So if you saw them contract a germ or a piece of information, you would know that, soon enough, everybody was about to contract this germ or this piece of information. And this would be much better than monitoring six randomly chosen people, without reference to the structure of the population. And in fact, if you could do that, what you would see is something like this. On the left-hand panel, again, we have the S-shaped curve of adoption. In the dotted red line, we show what the adoption would be in the random people, and in the left-hand line, shifted to the left, we show what the adoption would be in the central individuals within the network. On the Y-axis is the cumulative instances of contagion, and on the X-axis is the time. And on the right-hand side, we show the same data, but here with daily incidence. And what we show here is -- like, here -- very few people are affected, more and more and more and up to here, and here's the peak of the epidemic. But shifted to the left is what's occurring in the central individuals. And this difference in time between the two is the early detection, the early warning we can get, about an impending epidemic in the human population.
The problem, however, is that mapping human social networks is not always possible. It can be expensive, not feasible, unethical, or, frankly, just not possible to do such a thing. So, how can we figure out who the central people are in a network without actually mapping the network? What we came up with was an idea to exploit an old fact, or a known fact, about social networks, which goes like this: Do you know that your friends have more friends than you do? Your friends have more friends than you do, and this is known as the friendship paradox. Imagine a very popular person in the social network -- like a party host who has hundreds of friends -- and a misanthrope who has just one friend, and you pick someone at random from the population; they were much more likely to know the party host. And if they nominate the party host as their friend, that party host has a hundred friends, therefore, has more friends than they do. And this, in essence, is what's known as the friendship paradox. The friends of randomly chosen people have higher degree, and are more central than the random people themselves.
And you can get an intuitive appreciation for this if you imagine just the people at the perimeter of the network. If you pick this person, the only friend they have to nominate is this person, who, by construction, must have at least two and typically more friends. And that happens at every peripheral node. And in fact, it happens throughout the network as you move in, everyone you pick, when they nominate a random -- when a random person nominates a friend of theirs, you move closer to the center of the network. So, we thought we would exploit this idea in order to study whether we could predict phenomena within networks. Because now, with this idea we can take a random sample of people, have them nominate their friends, those friends would be more central, and we could do this without having to map the network.
And we tested this idea with an outbreak of H1N1 flu at Harvard College in the fall and winter of 2009, just a few months ago. We took 1,300 randomly selected undergraduates, we had them nominate their friends, and we followed both the random students and their friends daily in time to see whether or not they had the flu epidemic. And we did this passively by looking at whether or not they'd gone to university health services. And also, we had them [ actively ] email us a couple of times a week. Exactly what we predicted happened. So the random group is in the red line. The epidemic in the friends group has shifted to the left, over here. And the difference in the two is 16 days. By monitoring the friends group, we could get 16 days advance warning of an impending epidemic in this human population.
Now, in addition to that, if you were an analyst who was trying to study an epidemic or to predict the adoption of a product, for example, what you could do is you could pick a random sample of the population, also have them nominate their friends and follow the friends and follow both the randoms and the friends. Among the friends, the first evidence you saw of a blip above zero in adoption of the innovation, for example, would be evidence of an impending epidemic. Or you could see the first time the two curves diverged, as shown on the left. When did the randoms -- when did the friends take off and leave the randoms, and [ when did ] their curve start shifting? And that, as indicated by the white line, occurred 46 days before the peak of the epidemic. So this would be a technique whereby we could get more than a month-and-a-half warning about a flu epidemic in a particular population.
I should say that how far advanced a notice one might get about something depends on a host of factors. It could depend on the nature of the pathogen -- different pathogens, using this technique, you'd get different warning -- or other phenomena that are spreading, or frankly, on the structure of the human network. Now in our case, although it wasn't necessary, we could also actually map the network of the students.
So, this is a map of 714 students and their friendship ties. And in a minute now, I'm going to put this map into motion. We're going to take daily cuts through the network for 120 days. The red dots are going to be cases of the flu, and the yellow dots are going to be friends of the people with the flu. And the size of the dots is going to be proportional to how many of their friends have the flu. So bigger dots mean more of your friends have the flu. And if you look at this image -- here we are now in September the 13th -- you're going to see a few cases light up. You're going to see kind of blooming of the flu in the middle. Here we are on October the 19th. The slope of the epidemic curve is approaching now, in November. Bang, bang, bang, bang, bang -- you're going to see lots of blooming in the middle, and then you're going to see a sort of leveling off, fewer and fewer cases towards the end of December. And this type of a visualization can show that epidemics like this take root and affect central individuals first, before they affect others.
Now, as I've been suggesting, this method is not restricted to germs, but actually to anything that spreads in populations. Information spreads in populations, norms can spread in populations, behaviors can spread in populations. And by behaviors, I can mean things like criminal behavior, or voting behavior, or health care behavior, like smoking, or vaccination, or product adoption, or other kinds of behaviors that relate to interpersonal influence. If I'm likely to do something that affects others around me, this technique can get early warning or early detection about the adoption within the population. The key thing is that for it to work, there has to be interpersonal influence. It can not be because of some broadcast mechanism affecting everyone uniformly.
Now the same insights can also be exploited -- with respect to networks -- can also be exploited in other ways, for example, in the use of targeting specific people for interventions. So, for example, most of you are probably familiar with the notion of herd immunity. So, if we have a population of a thousand people, and we want to make the population immune to a pathogen, we don't have to immunize every single person. If we immunize 960 of them, it's as if we had immunized a hundred [ percent ] of them. Because even if one or two of the non-immune people gets infected, there's no one for them to infect. They are surrounded by immunized people. So 96 percent is as good as 100 percent. Well, some other scientists have estimated what would happen if you took a 30 percent random sample of these 1000 people,300 people and immunized them. Would you get any population-level immunity? And the answer is no. But if you took this 30 percent, these 300 people and had them nominate their friends and took the same number of vaccine doses and vaccinated the friends of the 300 -- the 300 friends -- you can get the same level of herd immunity as if you had vaccinated 96 percent of the population at a much greater efficiency, with a strict budget constraint.
And similar ideas can be used, for instance, to target distribution of things like bed nets in the developing world. If we could understand the structure of networks in villages, we could target to whom to give the interventions to foster these kinds of spreads. Or, frankly, for advertising with all kinds of products. If we could understand how to target, it could affect the efficiency of what we're trying to achieve. And in fact, we can use data from all kinds of sources nowadays [ to do this ] .
This is a map of eight million phone users in a European country. Every dot is a person, and every line represents a volume of calls between the people. And we can use such data, that's being passively obtained, to map these whole countries and understand who is located where within the network. Without actually having to query them at all, we can get this kind of a structural insight. And other sources of information, as you're no doubt aware are available about such features, from email interactions, online interactions, online social networks and so forth. And in fact, we are in the era of what I would call "massive-passive" data collection efforts. They're all kinds of ways we can use massively collected data to create sensor networks to follow the population, understand what's happening in the population, and intervene in the population for the better. Because these new technologies tell us not just who is talking to whom, but where everyone is, and what they're thinking based on what they're uploading on the Internet, and what they're buying based on their purchases. And all this administrative data can be pulled together and processed to understand human behavior in a way we never could before.
So, for example, we could use truckers' purchases of fuel. So the truckers are just going about their business, and they're buying fuel. And we see a blip up in the truckers' purchases of fuel, and we know that a recession is about to end. Or we can monitor the velocity with which people are moving with their phones on a highway, and the phone company can see, as the velocity is slowing down, that there's a traffic jam. And they can feed that information back to their subscribers, but only to their subscribers on the same highway located behind the traffic jam! Or we can monitor doctors prescribing behaviors, passively, and see how the diffusion of innovation with pharmaceuticals occurs within [ networks of ] doctors. Or again, we can monitor purchasing behavior in people and watch how these types of phenomena can diffuse within human populations.
And there are three ways, I think, that these massive-passive data can be used. One is fully passive, like I just described -- as in, for instance, the trucker example, where we don't actually intervene in the population in any way. One is quasi-active, like the flu example I gave, where we get some people to nominate their friends and then passively monitor their friends -- do they have the flu, or not? -- and then get warning. Or another example would be, if you're a phone company, you figure out who's central in the network and you ask those people, "Look, will you just text us your fever every day? Just text us your temperature." And collect vast amounts of information about people's temperature, but from centrally located individuals. And be able, on a large scale, to monitor an impending epidemic with very minimal input from people. Or, finally, it can be more fully active -- as I know subsequent speakers will also talk about today -- where people might globally participate in wikis, or photographing, or monitoring elections, and upload information in a way that allows us to pool information in order to understand social processes and social phenomena.
In fact, the availability of these data, I think, heralds a kind of new era of what I and others would like to call "computational social science." It's sort of like when Galileo invented -- or, didn't invent -- came to use a telescope and could see the heavens in a new way, or Leeuwenhoek became aware of the microscope -- or actually invented -- and could see biology in a new way. But now we have access to these kinds of data that allow us to understand social processes and social phenomena in an entirely new way that was never before possible. And with this science, we can understand how exactly the whole comes to be greater than the sum of its parts. And actually, we can use these insights to improve society and improve human well-being.
Thank you.
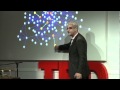
私はこの10年間 人はどのようにそしてなぜ 社会的ネットワークを 形成するのか解明しようと努力してきました ここで言う社会的ネットワークとは 最近のインターネット上のものでなく どちらかというと アフリカのサバンナに出現して以来何十万年もの間 人類が築いてきた社会的つながりです つまり私が友人関係や同僚関係 そして兄弟関係や親類関係を持ち その人達が似た関係を他の人達と持ち これが果てしなくずっと広がっていって このようなネットワークができます それぞれの点は人で 間の線は二人が関係していることを表します いろいろな人間関係です このような広大な人間社会の構造ができ 私達は皆その一部となっています
私は同僚のジェームス フォウラーと かなり以前から どのような数学的 社会的 生物学的そして心理学的な法則が これらのネットワークの構築を左右するのか またどんな法則がどうネットワークを動かし 人々の生活に影響するのかについて研究してきました そして最近は 解明するだけでなく その洞察を利用して実際に 世の中を改善する方法を見つけ もっと役立つことをして 何かを解決したりできないかと考えています そこでまず取り組もうと思ったのが 疫病の流行を予想することでした
疫病対策センターやその他の国家機関での 感染症流行の予測技術の現状は 現場の医師や研究所が報告する 特定の疾患の有病率や 発生率のデータを 機関の拠点から収集するというものです 患者の誰々さんが何かの病気だと診断された 他にも発症した患者がいた こうしたデータが情報センターにいくらか遅れて入るわけです 滞りなくすべて進めば 今日どこで疫病が流行っていたか 1~2週間後に分かるのです 実のところ 1年ほど前に 「インフルトレンド」という グーグルのツールが広まりました 人々の現在の検索パターンを見て インフルエンザの発生地域 現在の流行状況や 有病率が把握できるのです
でも今日皆さんにお見せしたいのは 伝染病の発生を 迅速に警告するだけでなく 実際にその流行を 早期に感知できるかもしれない ひとつの方法です 事実 このアイデアは 細菌による感染症を予測するだけでなく 様々なタイプの流行の予想に応用できます 例えば社会的感染という形で広まるものは すべてこうして理解できます 図の左に示した愛国心や 利他主義や宗教のような抽象的な概念から 食生活や書籍購入 そして飲酒などの習慣 自転車ヘルメット着用などの安全習慣や 売れる商品 電子機器の購入などまで 人を通して広がるものすべてです 新しいアイデアの普及なども 今からご覧いただく方法によって 理解し予測することが可能です
おそらく皆さんご存知だと思いますが 普及を表すには従来 イノベーション普及率という 採用曲線を使用します Y軸は何%の人が影響されているか そしてX軸は時間を表します 最初の時点ではあまり多くの人が影響されておらず 典型的なS字型カーブの グラフになります なぜこのような形になるのかと言うと 一番初めに1人か2人が 影響または感染されているとすると その2人が次の2人を感染させ 次に感染されるのは4人 そして8人 16人と増え 流行の増殖期のカーブを形成するからです 最終的には人口のほとんどが感染され まだ感染されていない人が どんどん少なくなり カーブは頭打ちとなります そして典型的なS字型カーブとなるのです これは病原菌やアイデア 製品普及や習慣のようなものでも 同じです でも物事は人々の間でランダムに普及しません 普及はネットワークを通して行なわれます 私達は皆 ネットワークの中で生きているからです そしてこれらのネットワークには特定の構造があります
こちらのネットワークを見てください 105人います 点は人を表し 線は友人関係を表します 人によってネットワーク内の位置が 違うことが分かると思います また人間関係も多様です 友人関係 兄弟関係 夫婦関係 同僚関係 隣人関係などいろいろあります そして関係によって 違うものが広がります 例えば性感染症は 性的つながりをもって広がります 喫煙習慣は 友人関係に影響されるかもしれません 利他的または慈善的行為だと 同僚に感化されてかもしれませんし 隣人の影響かもしれません でもネットワーク内の位置のすべてが平等というわけではありません
これを見てもらえばすぐ分かりますが つながりの数は人によって違います 1つの人もいれば2つの人もいて 6つの人もいれば10個の人もいます これはノードの度数とも言われ 節点の持つつながりの数です しかしそれだけではありません 節点AとBを見てもらうと 両者とも6つのつながりを持っています でもこの図を全体的に見ると 節点AとBには大きな違いがあると 気づくと思います こう考えたら分かりやすいと思います もし致死的な病原菌が ネットワーク内で広まっていたらAとBのどちらになりたいですか? (聴衆:B) クリスタキス:もちろんBですね Bはネットワークの端に位置しています では気になる噂話が ネットワーク内で流れていたらどちらになりたいですか? Aですね 一見して Aの方がいち早く 広まる噂を耳にする可能性が高いと分かります これはネットワーク構造上の位置のおかげです 実際にAは中心寄りに位置しており これは数式で表すことができます ですから ネットワークを通じて 広がっている何かを追跡したい場合 節点Aも含んだ ネットワークの中心部の人々に センサーをつけ その人々を観察することによって ネットワークを介して広がっている何かを 早期発見するのが理想です
この人々が病気に感染したり情報を得たら 近いうちに 全員にこの病原菌または情報が 伝わるだろうと分かるのです この方法は集団の構造を踏まえずに ランダムに選出した 6人を観察するよりずっと効果的です 実際 中心部の人々を観察できれば このような結果が見られる筈です 左の図には前に見たS字型の採用曲線があります 赤の点線は ランダムに選出された人々の間での普及です 左側の左にずれている線は ネットワーク中心部の人々の間での 普及を表します Y軸は感染者の累積人数です X軸は時間です 右にあるのは同じデータですが 1日ごとの発症件数です ここにご覧いただけるのは たった数人の感染者からどんどん増えて ここで流行のピークとなることです 左にずれたグラフが中心部の人々の状態です そしてこの2つの間の時間差が 兆しとなり この人々の間で流行が起こる 早期警告となるのです
しかし問題は 社会的ネットワークを図にするのが いつも可能なわけでないことです コストが高すぎたり実施が難しかったり 倫理的でなかったり ただ単にそんなことは不可能な場合もあります では実際にネットワークを図にしないで どのように中心にいるのは誰かを 調べることができるのでしょうか? 我々が思いついたのは 社会的ネットワークについて前から 知られている現象を利用することでした このような現象です あなたの友人にはあなたより たくさん友人がいると知っていましたか? あなたの友人にはあなたより友人がいるのです 「友人関係のパラドックス」と言われています 社会的ネットワークの中でとても人気があり 友人が多いパーティのホストと 友人は1人だけの人間嫌いがいるとします ここからランダムに選ばれた人はパーティのホストを 知っている確率の方が高いのです 彼らがパーティのホストを友人として挙げたら パーティのホストには大勢の友人がいるので 彼らよりも友人が多いということになります 基本的にこれが「友人関係のパラドックス」というものです ランダムに選ばれた人達よりその友人達の方が より多くのつながりを持ち 中心寄りの位置にいるのです
ネットワークの端の方にいる人々に注目すると このことが自然に理解できると思います この人を見ると 友人として挙げられるのはこの人しかいません そして この人にはネットワークの構造上 最低2人 通常はそれ以上の友人がいることになります 端の節点のどれをとってもこの現象は見られ 実際ネットワークの中心に向かって全体的に見られます 誰を選出してもです ランダムに選出された人が友人を挙げると ネットワークの中心に近づくわけです そこで我々はこのアイデアを利用して ネットワーク内の現象を予測できるか研究しようと考えました このアイデアをもとにすれば ネットワークの図がなくても 集団からランダムに誰かを選び 友人を挙げてもらって 中央寄りの人の選出ができるからです
我々はハーバード大学での新型インフルエンザの発生で これを検証しました つい2~3ヶ月前の2009年秋から冬でした ランダムに選出した学部生1300人に 友人を挙げてもらい そのランダムの学生と友人の両方を 毎日 追跡調査して 流行のインフルエンザへの感染を調べました 大学内診療所の利用監視と 週に数回のメール報告での調査です すると我々が予想した通りのことが起こりました 赤い線がランダムのグループです 友人グループの中での流行は左のこちらへ寄っています 2つのグループの違いは16日です 友人グループを追跡することによって この集団における感染流行を 16日前に警告できるわけです
またそれだけでなく アナリストが流行の研究や 新製品の普及の予測をしようするときに 集団からランダムに選んだサンプルと さらに挙げてもらった友人の 両方のグループを追跡することができます 例えばその友人グループで イノベーション普及に急上昇があれば 流行の兆しとなります また左にあるように2つの線が 分岐し始めるのもサインです 友人グループの線が急上昇し ランダムサンプルのグループに差をつけて 開き始めたのはどの時点か? それはこの白い線が示す時点で 流行のピークの 46日前でした つまりこの方法を使えば 一定の集団の中で起こる インフルエンザの流行を1ヵ月半以上前に察知できるのです
どのくらい前の時点で そのような兆しが見られるかは 様々な要素により異なると思います 病原体の特性によることもあり得ます この方法で違う種類の病原体を 見た場合 異なる兆候が出ると思います 他の広がっている現象でもそうです 人のネットワークの構造が違うからということもあります 我々の実例では必要ではなかったのですが 実際に 学生のネットワークを図にすることが出来ました
これが714人の学生と 彼らの友人のつながりを示した図です これからこの図の移り変わりを見せます ネットワークの日々の変化を 120日分見てみましょう 赤い点がインフルエンザの感染を示します そして黄色い点がインフルエンザ感染者の友人です 点の大きさはインフルエンザに感染している 友人の数に応じて大きくなります つまり大きい点はインフルエンザに感染した友人が多い人です この図を見てください 9月13日の状態です いくつか色のついた点がみられます インフルエンザが中心でポツポツ見られます 今10月19日の状態です 11月になると流行のカーブが立ち上がり パッパッと中心部で次々に感染が広がります そしてだんだん頭打ちになっていきます 12月末に近づくにつれて感染がどんどん少なくなります このような可視化によって こういった流行はまず 中央部の人間から感染して 他の人々に感染することが明らかになります
それで今まで申し上げてきたように この方法は細菌だけでなく 人々の間で伝染するもの何にでも使えます 情報は人々を通じて広がります 常識も人から人へと広がります 言動も人々の間で広がります 言動というのは犯罪行為や 選挙投票もあれば 健康管理行為で 喫煙や予防接種のようなこともあり 製品普及やその他の行動で 人間同士が影響し合うものもあります 言動によって回りの人間が影響される傾向があったら この方法により その集団における流行の発生や 兆しを早期に知り得ることができるわけです この方法が成り立つポイントは 人間同士の影響があることです 一斉に実施され全員が 同じように影響されるような仕組みでは駄目です
さてこの同じ洞察を違うやり方で ネットワークに関連するものに対して 活用することもできます 介入目的のために特定の人々を 対象として選ぶのに利用するのが一例です 例えば皆さん集団免疫については たぶん知っていると思いますが 1000人のグループがいたとして このグループをある病原体から守りたい場合 全員に予防接種する必要はありません このうち960人に免疫ができれば 1000人に予防接種したのと同じになります たとえ1人か2人の免疫のない人が感染しても その人達が病気をうつす相手がいないからです 免疫のある人ばかりに囲まれているわけです このように96%は100%と同じくらい効果的です 1000人の中から30%をランダムで選出し 予防接種をしたらどうなるか 計算した科学者達がいましたが 集団レベルでの免疫が得られるかと言うと 得られません でもこの同じ30%の300人に 友人を挙げてもらって 同じ数の予防接種を 300人が挙げた友人達300人に 実施すると 集団免疫と同等の免疫ができます 集団の96%に予防接種したのと同じ効果を 厳しい予算でもずっと効率よく得られるのです
似たようなアイデアを使って 発展途上国で蚊帳などを配布する際に 対象者を限定することもできます 村のネットワークの構造が分かっていれば 蚊帳などの普及を促進する中心部の 人々をターゲットにして介入援助できます また率直に言って これはどんな商品の宣伝にも使えます 対象者の選定の仕方が分かれば 目的を達成する効率を 上げることができます 事実 現在ありとあらゆるところで 集められているデータを利用できます
こちらはヨーロッパにおける 800万人の電話利用者の関係図です それぞれの点は人を表し 線はその人達の間の電話回数を表します 私達はこのような自動的に集められたデータによって これらの国の全体像を見たり ネットワークのどこに誰がいるか理解できます 特別なデータ処理などしなくても このような構造の洞察を得ることができるのです お気づきと思いますが このようなデータは 他の情報源からも手にすることができます メールやインターネット上のやりとり ソーシャルネットワークなどです 実際 今の時代は 大量のデータが自動的に蓄積されています 大量に収集されたデータの使い道は幾通りもあります 集団を追跡するためのセンサーとなる 中心部の人々を特定したり その集団の中で何が起こっているか理解したり 改善の為に介入したりできます 最近の技術では 誰と誰がしゃべっているかだけでなく 人々がどこにいるのかも分かるからです アップロードされるものから人々が考えていることが分かり 購入記録から商品の売れ筋も分かります これらすべての管理データを合わせて処理すれば 人々の行動を 以前はできなかった方法で理解できます
トラックの運転手による燃料購入を例にします 運転手達は普段通りに仕事をして 燃料を購入します 私たちは燃料の購入量が急上昇するのを見て 経済低迷期の終わりが近いと分かります または人々が高速道路を 移動している速度を携帯電話で計測することもできます 電話会社は 速度が落ちるのを見て 渋滞を感知できます 更にその情報を携帯電話ユーザーに提供できるわけです それも同じ高速道路上で その渋滞の後続のユーザーに限定できます 医師の薬品処方状況を観察することもできます 新規の医薬品がどのように医師の間で普及するのか 理解することができます 人々の商品購入状況の観察をして このようなタイプの現象がどうやって 人々の間で普及するのか確認することができます
自動蓄積された大量データの利用法は 3つあると思います 1つ目は完全に受身的な 先ほど説明したようなものです トラックの運転手の例のような 実際には集団に一切介入しないしないものです そして半能動的な 例に挙げたインフルエンザのような 人々に友人を挙げてもらい 彼らがインフルエンザに感染しないか 観察して警告を受けるものもあります 別の例として 電話会社がネットワークの中心に位置する人を調べて 「毎日熱を測って携帯メールで送ってもらえますか?」 「体温だけでかまいません」と頼み 大量の体温データを中心部の人々に限定して 収集することも考えられます こうして人々の最低限の情報提供だけで 伝染病の流行の兆しを 広範囲に監視できるのです またはもっと積極的なアプローチもできます このあとの講演者も話しますが 人々が世界中からウィキに参加したり 写真や選挙の追跡をしたりして 情報をアップロードしたものを 社会的プロセスや現象を理解するために 収集することもできます
事実これらのデータが入手できるのは 専門家が言うところの 「計算社会科学」のような一種の新たな 時代の到来を告げています これはガリレオが望遠鏡を使って それまでにないやり方で 天空の観察ができたことや レーウェンフクが顕微鏡を発明し 生物学に新たな見解を もたらしたことに似ています 今度は大量データが入手できるようになり 社会的プロセスや現象を 以前にはなかったやり方で 理解することができるようになったわけです そしてこの科学により私達は 社会全体が具体的にどうやって ただ一人ひとりを足しただけよりも 偉大となるのか理解することができるのです そして実際にこれらの洞察を利用して 社会および人々の生活を改善できるのです
ありがとうございました
品詞分類
- 主語
- 動詞
- 助動詞
- 準動詞
- 関係詞等
TED 日本語
TED Talks
関連動画
十代の科学者が考えた、傷の治癒を促す発明アヌシュカ・ナイクナーレ
2018.06.19自分の声が嫌だと感じるのはなぜかレベッカ・クラインバーガー
2018.05.24若い発明家の発泡スチロールリサイクル計画アシュトン・コーファー
2017.04.18においの「自撮り」やその他の合成生物学的な実験アニー・リュー
2017.03.17物理学の未解決問題をいかに探求するかジェームズ・ビーチャム
2017.01.20世界を理解する奥義としての数学ロジャー・アントンセン
2016.12.13機械に奪われる仕事 ― そして残る仕事アンソニー・ゴールドブルーム
2016.08.31次なる科学の大発見とは?エリック・ヘイゼルティン
2016.08.18数学の何がそれほど魅惑的なのかセドリック・ヴィラニ
2016.06.28飛行機内の病原菌の動き方と対処法レイモンド・ワン
2016.01.11科学を良い方向に進める知られざる頭脳ジャダイダ・アイスラー
2016.01.07量子生物学は生命の最大の謎を解明するか?ジム・アルカリリ
2015.09.16バスケットボールの激しい動きの背後にある数学ラジブ・マヒシュワラン
2015.07.06笑わせ、そして考えさせる科学賞マーク・エイブラハムズ
2014.10.24科学者を信頼すべき理由ナオミ・オレスケス
2014.06.25知能の方程式アレックス・ウィスナー=グロス
2014.02.06
洋楽 おすすめ
RECOMMENDS
洋楽歌詞
ステイザ・キッド・ラロイ、ジャスティン・ビーバー
洋楽最新ヒット2021.08.20スピーチレス~心の声ナオミ・スコット
洋楽最新ヒット2019.05.23シェイプ・オブ・ユーエド・シーラン
洋楽人気動画2017.01.30フェイデッドアラン・ウォーカー
洋楽人気動画2015.12.03ウェイティング・フォー・ラヴアヴィーチー
洋楽人気動画2015.06.26シー・ユー・アゲインウィズ・カリファ
洋楽人気動画2015.04.06シュガーマルーン5
洋楽人気動画2015.01.14シェイク・イット・オフテイラー・スウィフト
ポップス2014.08.18オール・アバウト・ザット・ベースメーガン・トレイナー
ポップス2014.06.11ストーリー・オブ・マイ・ライフワン・ダイレクション
洋楽人気動画2013.11.03コール・ミー・メイビーカーリー・レイ・ジェプセン
洋楽人気動画2012.03.01美しき生命コールドプレイ
洋楽人気動画2008.08.04バッド・デイ~ついてない日の応援歌ダニエル・パウター
洋楽人気動画2008.05.14サウザンド・マイルズヴァネッサ・カールトン
洋楽人気動画2008.02.19イッツ・マイ・ライフボン・ジョヴィ
ロック2007.10.11アイ・ウォント・イット・ザット・ウェイバックストリート・ボーイズ
洋楽人気動画2007.09.14マイ・ハート・ウィル・ゴー・オンセリーヌ・ディオン
洋楽人気動画2007.07.12ヒーローマライア・キャリー
洋楽人気動画2007.03.21オールウェイズ・ラヴ・ユーホイットニー・ヒューストン
洋楽人気動画2007.02.19オネスティビリー・ジョエル
洋楽人気動画2005.09.16