
TED日本語
TED Talks(英語 日本語字幕付き動画)
TED日本語 - クリス・アームソン: 自動運転車は周りの世界をどう見ているのか
TED Talks
自動運転車は周りの世界をどう見ているのか
How a driverless car sees the road
クリス・アームソン
Chris Urmson
内容
統計的に自動車おいて、信頼性の最も低い要素が何かというと、それは運転手です。現在進められている、運転席から人間をなくそうという企ての1つ、Googleの自動運転車プログラムを率いるクリス・アームソンが、自動運転車の現状について語り、自動運転車はどのように道路を見、次にどうすべきか自律的に判断しているのか、興味深い映像を使って紹介します。
字幕
SCRIPT
Script
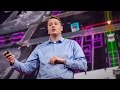
So in 1885, Karl Benz invented the automobile. Later that year, he took it out for the first public test drive, and -- true story -- crashed into a wall. For the last 130 years, we've been working around that least reliable part of the car, the driver. We've made the car stronger. We've added seat belts, we've added air bags, and in the last decade, we've actually started trying to make the car smarter to fix that bug, the driver.
Now, today I'm going to talk to you a little bit about the difference between patching around the problem with driver assistance systems and actually having fully self-driving cars and what they can do for the world. I'm also going to talk to you a little bit about our car and allow you to see how it sees the world and how it reacts and what it does, but first I'm going to talk a little bit about the problem. And it's a big problem: 1.2 million people are killed on the world's roads every year. In America alone,33,000 people are killed each year. To put that in perspective, that's the same as a 737 falling out of the sky every working day. It's kind of unbelievable. Cars are sold to us like this, but really, this is what driving's like. Right? It's not sunny, it's rainy, and you want to do anything other than drive. And the reason why is this: Traffic is getting worse. In America, between 1990 and 2010, the vehicle miles traveled increased by 38 percent. We grew by six percent of roads, so it's not in your brains. Traffic really is substantially worse than it was not very long ago.
And all of this has a very human cost. So if you take the average commute time in America, which is about 50 minutes, you multiply that by the 120 million workers we have, that turns out to be about six billion minutes wasted in commuting every day. Now, that's a big number, so let's put it in perspective. You take that six billion minutes and you divide it by the average life expectancy of a person, that turns out to be 162 lifetimes spent every day, wasted, just getting from A to B. It's unbelievable. And then, there are those of us who don't have the privilege of sitting in traffic. So this is Steve. He's an incredibly capable guy, but he just happens to be blind, and that means instead of a 30-minute drive to work in the morning, it's a two-hour ordeal of piecing together bits of public transit or asking friends and family for a ride. He doesn't have that same freedom that you and I have to get around. We should do something about that.
Now, conventional wisdom would say that we'll just take these driver assistance systems and we'll kind of push them and incrementally improve them, and over time, they'll turn into self-driving cars. Well, I'm here to tell you that's like me saying that if I work really hard at jumping,one day I'll be able to fly. We actually need to do something a little different. And so I'm going to talk to you about three different ways that self-driving systems are different than driver assistance systems. And I'm going to start with some of our own experience.
So back in 2013, we had the first test of a self-driving car where we let regular people use it. Well, almost regular -- they were 100 Googlers, but they weren't working on the project. And we gave them the car and we allowed them to use it in their daily lives. But unlike a real self-driving car, this one had a big asterisk with it: They had to pay attention, because this was an experimental vehicle. We tested it a lot, but it could still fail. And so we gave them two hours of training, we put them in the car, we let them use it, and what we heard back was something awesome, as someone trying to bring a product into the world. Every one of them told us they loved it. In fact, we had a Porsche driver who came in and told us on the first day, "This is completely stupid. What are we thinking?" But at the end of it, he said, "Not only should I have it, everyone else should have it, because people are terrible drivers." So this was music to our ears, but then we started to look at what the people inside the car were doing, and this was eye-opening. Now, my favorite story is this gentleman who looks down at his phone and realizes the battery is low, so he turns around like this in the car and digs around in his backpack, pulls out his laptop, puts it on the seat, goes in the back again, digs around, pulls out the charging cable for his phone, futzes around, puts it into the laptop, puts it on the phone. Sure enough, the phone is charging. All the time he's been doing 65 miles per hour down the freeway. Right? Unbelievable. So we thought about this and we said, it's kind of obvious, right? The better the technology gets, the less reliable the driver is going to get. So by just making the cars incrementally smarter, we're probably not going to see the wins we really need.
Let me talk about something a little technical for a moment here. So we're looking at this graph, and along the bottom is how often does the car apply the brakes when it shouldn't. You can ignore most of that axis, because if you're driving around town, and the car starts stopping randomly, you're never going to buy that car. And the vertical axis is how often the car is going to apply the brakes when it's supposed to to help you avoid an accident. Now, if we look at the bottom left corner here, this is your classic car. It doesn't apply the brakes for you, it doesn't do anything goofy, but it also doesn't get you out of an accident. Now, if we want to bring a driver assistance system into a car, say with collision mitigation braking, we're going to put some package of technology on there, and that's this curve, and it's going to have some operating properties, but it's never going to avoid all of the accidents, because it doesn't have that capability. But we'll pick some place along the curve here, and maybe it avoids half of accidents that the human driver misses, and that's amazing, right? We just reduced accidents on our roads by a factor of two. There are now 17,000 less people dying every year in America.
But if we want a self-driving car, we need a technology curve that looks like this. We're going to have to put more sensors in the vehicle, and we'll pick some operating point up here where it basically never gets into a crash. They'll happen, but very low frequency. Now you and I could look at this and we could argue about whether it's incremental, and I could say something like "80-20 rule," and it's really hard to move up to that new curve. But let's look at it from a different direction for a moment. So let's look at how often the technology has to do the right thing. And so this green dot up here is a driver assistance system. It turns out that human drivers make mistakes that lead to traffic accidents about once every 100,000 miles in America. In contrast, a self-driving system is probably making decisions about 10 times per second, so order of magnitude, that's about 1,000 times per mile. So if you compare the distance between these two, it's about 10 to the eighth, right? Eight orders of magnitude. That's like comparing how fast I run to the speed of light. It doesn't matter how hard I train, I'm never actually going to get there. So there's a pretty big gap there.
And then finally, there's how the system can handle uncertainty. So this pedestrian here might be stepping into the road, might not be. I can't tell, nor can any of our algorithms, but in the case of a driver assistance system, that means it can't take action, because again, if it presses the brakes unexpectedly, that's completely unacceptable. Whereas a self-driving system can look at that pedestrian and say, I don't know what they're about to do, slow down, take a better look, and then react appropriately after that.
So it can be much safer than a driver assistance system can ever be. So that's enough about the differences between the two. Let's spend some time talking about how the car sees the world.
So this is our vehicle. It starts by understanding where it is in the world, by taking a map and its sensor data and aligning the two, and then we layer on top of that what it sees in the moment. So here, all the purple boxes you can see are other vehicles on the road, and the red thing on the side over there is a cyclist, and up in the distance, if you look really closely, you can see some cones. Then we know where the car is in the moment, but we have to do better than that: we have to predict what's going to happen. So here the pickup truck in top right is about to make a left lane change because the road in front of it is closed, so it needs to get out of the way. Knowing that one pickup truck is great, but we really need to know what everybody's thinking, so it becomes quite a complicated problem. And then given that, we can figure out how the car should respond in the moment, so what trajectory it should follow, how quickly it should slow down or speed up. And then that all turns into just following a path: turning the steering wheel left or right, pressing the brake or gas. It's really just two numbers at the end of the day. So how hard can it really be?
Back when we started in 2009, this is what our system looked like. So you can see our car in the middle and the other boxes on the road, driving down the highway. The car needs to understand where it is and roughly where the other vehicles are. It's really a geometric understanding of the world. Once we started driving on neighborhood and city streets, the problem becomes a whole new level of difficulty. You see pedestrians crossing in front of us, cars crossing in front of us, going every which way, the traffic lights, crosswalks. It's an incredibly complicated problem by comparison. And then once you have that problem solved, the vehicle has to be able to deal with construction. So here are the cones on the left forcing it to drive to the right, but not just construction in isolation, of course. It has to deal with other people moving through that construction zone as well. And of course, if anyone's breaking the rules, the police are there and the car has to understand that that flashing light on the top of the car means that it's not just a car, it's actually a police officer. Similarly, the orange box on the side here, it's a school bus, and we have to treat that differently as well.
When we're out on the road, other people have expectations: So, when a cyclist puts up their arm, it means they're expecting the car to yield to them and make room for them to make a lane change. And when a police officer stood in the road, our vehicle should understand that this means stop, and when they signal to go, we should continue.
Now, the way we accomplish this is by sharing data between the vehicles. The first, most crude model of this is when one vehicle sees a construction zone, having another know about it so it can be in the correct lane to avoid some of the difficulty. But we actually have a much deeper understanding of this. We could take all of the data that the cars have seen over time, the hundreds of thousands of pedestrians, cyclists, and vehicles that have been out there and understand what they look like and use that to infer what other vehicles should look like and other pedestrians should look like. And then, even more importantly, we could take from that a model of how we expect them to move through the world. So here the yellow box is a pedestrian crossing in front of us. Here the blue box is a cyclist and we anticipate that they're going to nudge out and around the car to the right. Here there's a cyclist coming down the road and we know they're going to continue to drive down the shape of the road. Here somebody makes a right turn, and in a moment here, somebody's going to make a U-turn in front of us, and we can anticipate that behavior and respond safely.
Now, that's all well and good for things that we've seen, but of course, you encounter lots of things that you haven't seen in the world before. And so just a couple of months ago, our vehicles were driving through Mountain View, and this is what we encountered. This is a woman in an electric wheelchair chasing a duck in circles on the road. (Laughter) Now it turns out, there is nowhere in the DMV handbook that tells you how to deal with that, but our vehicles were able to encounter that, slow down, and drive safely. Now, we don't have to deal with just ducks. Watch this bird fly across in front of us. The car reacts to that. Here we're dealing with a cyclist that you would never expect to see anywhere other than Mountain View. And of course, we have to deal with drivers, even the very small ones. Watch to the right as someone jumps out of this truck at us. And now, watch the left as the car with the green box decides he needs to make a right turn at the last possible moment. Here, as we make a lane change, the car to our left decides it wants to as well. And here, we watch a car blow through a red light and yield to it. And similarly, here, a cyclist blowing through that light as well. And of course, the vehicle responds safely. And of course, we have people who do I don't know what sometimes on the road, like this guy pulling out between two self-driving cars. You have to ask, "What are you thinking?" (Laughter)
Now, I just fire-hosed you with a lot of stuff there, so I'm going to break one of these down pretty quickly. So what we're looking at is the scene with the cyclist again, and you might notice in the bottom, we can't actually see the cyclist yet, but the car can: it's that little blue box up there, and that comes from the laser data. And that's not actually really easy to understand, so what I'm going to do is I'm going to turn that laser data and look at it, and if you're really good at looking at laser data, you can see a few dots on the curve there, right there, and that blue box is that cyclist. Now as our light is red, the cyclist's light has turned yellow already, and if you squint, you can see that in the imagery. But the cyclist, we see, is going to proceed through the intersection. Our light has now turned green, his is solidly red, and we now anticipate that this bike is going to come all the way across. Unfortunately the other drivers next to us were not paying as much attention. They started to pull forward, and fortunately for everyone, this cyclists reacts, avoids, and makes it through the intersection. And off we go.
Now, as you can see, we've made some pretty exciting progress, and at this point we're pretty convinced this technology is going to come to market. We do three million miles of testing in our simulators every single day, so you can imagine the experience that our vehicles have. We are looking forward to having this technology on the road, and we think the right path is to go through the self-driving rather than driver assistance approach because the urgency is so large. In the time I have given this talk today,34 people have died on America's roads.
How soon can we bring it out? Well, it's hard to say because it's a really complicated problem, but these are my two boys. My oldest son is 11, and that means in four and a half years, he's going to be able to get his driver's license. My team and I are committed to making sure that doesn't happen.
Thank you.
(Laughter) (Applause) Chris Anderson: Chris, I've got a question for you.
Chris Urmson: Sure.
CA: So certainly, the mind of your cars is pretty mind-boggling. On this debate between driver-assisted and fully driverless -- I mean, there's a real debate going on out there right now. So some of the companies, for example, Tesla, are going the driver-assisted route. What you're saying is that that's kind of going to be a dead end because you can't just keep improving that route and get to fully driverless at some point, and then a driver is going to say, "This feels safe," and climb into the back, and something ugly will happen.
CU: Right. No, that's exactly right, and it's not to say that the driver assistance systems aren't going to be incredibly valuable. They can save a lot of lives in the interim, but to see the transformative opportunity to help someone like Steve get around, to really get to the end case in safety, to have the opportunity to change our cities and move parking out and get rid of these urban craters we call parking lots, it's the only way to go.
CA: We will be tracking your progress with huge interest. Thanks so much, Chris. CU: Thank you. (Applause)
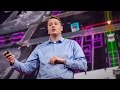
1885年 カール・ベンツが 自動車を発明しました その年 初の公開試乗会が 行われたとき 本当の話ですが 壁に激突したそうです それ以来 130年の間 私たちは この自動車の最も信頼性の低い部分 運転手をどうにかしようとしてきました 車体を強化したり シートベルトを付けたり エアバッグを付けたり この10年ほどは 自動車を賢くすることによって この運転手という欠陥部分を 正そうとするようになりました
今日 お話しするのは 運転支援システムによって この問題を手当てするアプローチと 車を完全に自動運転にする アプローチの違いと それでどんな恩恵があるのか ということです また私たちが開発している 自動運転車と それが周りの世界をどう見 どう反応しているのかも お見せしますが その前にまず 何が問題なのか話しましょう 大きな問題です 世界で毎年120万人が 交通事故によって死んでいます アメリカだけでも 毎年3万3千人が死んでいるんです 分かりやすく言うと これは737型旅客機が平日に毎日 墜落していることに相当します 信じがたいことです 自動車はこんなイメージで 売られていますが 実際の運転はこんな感じです 天気は悪く 運転中にみんな 他のことをしたくなります その理由は 交通事情が悪化しているからです アメリカでは1990年から 2010年までの間に 自動車の総走行距離は 38%増加しましたが 道路の増加は6%に留まります だから交通事情の変化は 単なる感覚的なものではなく この20年で現実に はっきり悪化しているんです
これは人々にコストとして かかってきます アメリカにおける平均通勤時間は 約50分ですが これを労働人口の1億2千万人と 掛け合わせると 毎日通勤のために 60億分という時間が 浪費されていることになります 大きな数字なので 分かりやすく言い換えましょう 60億分を 人の平均寿命で割ると 毎日162人分の命が この単なる移動時間のために 無駄になっている計算です 信じ難い話です それに加え この渋滞の中に座るという 特権を持たない人たちもいます 彼はスティーブといい とても有能な人ですが 目が見えません 仕事に行くのに 朝30分運転する代わりに 2時間かけて 交通機関を乗り継ぐか 送ってもらえるよう 友人や家族に頼まなければなりません 私たちのような 移動の自由を持っていないのです これはどうにかする必要があります
多くの人は 運転支援システムを作って 徐々に改良していけば いつか自動運転車ができると 考えます でもそれは 一生懸命ジャンプしてれば いつか空を飛べるようになると言うのと 同じようなものです 基本的に違うことを する必要があります 自動運転車が 運転支援システムと異なる点を 3つ示しますが まず私たちが経験したことを お話ししましょう
2013年に 初めて一般人を載せて 自動運転車のテストを行いました 100人のGoogle社員ではありますが このプロジェクト外の人たちです 自動運転車を渡して 日常的に使ってもらいました 自動運転車と言っても 留保付きです まだ実験段階のもので 運転席の人に注意を払ってもらう 必要がありました 十分テストはしていましたが それでも誤動作することがあったんです それで彼らに 2時間のトレーニングの後に 運転席についてもらい 使ってもらいました そうして得られた声は 新製品を世に送り出そうとしている者にとって 勇気づけられるものでした 誰もがとても 気に入ってくれたのです 普段ポルシェに 乗っている人がいて 最初は「こんなの馬鹿げている 何考えてんのか分からない」と言っていたんですが 終いにはこう言ったのです 「自分だけでなく すべての人がこの車を持つべきだ みんなひどいドライバーだから」 これは私たちの耳に 心地よい言葉でしたが 自動運転車に乗った人たちが 車内でやっていることには 驚かせられることになりました これは私のお気に入りの 逸話なんですが ある人が運転中に携帯を見て バッテリーが切れかけているのに気付き こんな風に後ろを向いて バックパックの中を探って ノートPCを取り出し 横の座席に置き また振り向いて バックパックを漁って 充電ケーブルを取り出し ノートPCと携帯を繋いで 携帯を充電したんですが この間車はずっと 時速100キロ以上で走っていました 信じられないような話です これを見て 答えは出たと思いましたね 技術が進むほど 運転手の信頼性は 逆に下がっていくので 自動車を徐々に 賢くしていっても 望むような結果には ならないだろうと
少し技術的な話をしましょう このグラフの横軸は 車がブレーキを かけるべきでない時にかける頻度です こちらの軸は概ね 無視して構いません 街中を運転していて車が ランダムに停止するようなら そんな車は誰も買わないからです 縦軸は事故を防ぐために 車がブレーキを かけるべき時にかける頻度です 左下隅が 昔ながらの自動車です 車が自分でブレーキをかけることはなく 馬鹿なことをすることもなければ 事故を防いでくれることも ありません 衝突回避自動ブレーキのような 運転支援システムを 作ろうと思ったら 色々な技術を取り込んで こんなグラフになり ある種の動作特性を 実現できるでしょうが 事故をすべて防げるわけではなく そこまでの能力はありません それでもこの曲線上の いずれかの点を選んで 事故の数を 半分にできるかもしれません これはすごいことです 交通事故が 半分になるんですから アメリカで毎年死ぬ人の数が 1万7千人も減らせます
しかし自動運転車を 作ろうと思ったら 曲線をこのようなものにする 技術が必要になります 車にもっとセンサーを付け 基本的に衝突が起こらないという 点を選ぶことになります 衝突は起きたとしても 極めて低い頻度です これを見て 移行は段階的であるべきか 議論ができるでしょう 80:20の法則というのもありますが 上の曲線に移るのは 極めて難しいのです これを別の角度から 見てみましょう この技術はどれくらいの精度で 正しく振る舞わなければならないのか? 緑の点は運転支援システムです アメリカでは 人間の運転手は 事故に繋がるようなミスを 10万マイル(16万km)に1度 犯しています これに対して自動運転車は 1秒間に10回くらい 判断を行っています 1マイルあたりだと およそ千回です この2点は対数目盛りで 8つ離れています 10の8乗倍です これは私が走る速さと 光の速さを 比較するようなものです 私がいかに頑張ったところで そこに到達することはありません とても大きなギャップが あるということです
最後にシステムが不確定さを いかに扱うかという問題があります この歩行者は 道を渡るのかどうか 私には分からないし どんなアルゴリズムでも分かりません 運転支援システムは 行動を起こせない ということです 予期しないところでブレーキをかけるというのは 受け入れられないからです 一方自動運転車の場合は 歩行者がどうしようとしているのか 分からないという場合 スピードを落として様子を見 それから適切に反応します
だから運転支援システムよりも ずっと安全だということです 2つのシステムの 違いについては分かったので 自動運転車は世界をどう見ているのか という話をしましょう
白いのが私たちの車です まず自分が世界のどこにいるのかを 知るところから始めます 地図とセンサーデータを突き合わせ 地図に現在見えているものを 重ね合わせます 紫色の箱は 道路上の他の車です 道の端にある赤い箱は 自転車です それからよく見てもらうと ずっと向こうに ロードコーンがあります これで車がいる状況については 分かりますが それだけでは駄目で この後何が起きるか予測する必要があります 右手前方にいる小型トラックは 左に車線変更しようとしています 道路の先が塞がっているのを 迂回するためです 1台のトラックの動きを 読むだけじゃいけません みんなの考えを読む必要があり これはとても複雑な問題です それを元に どう動くべきか判断します どういう経路を取り どうスピードを変えるか 道に沿って進む 左か右にハンドルを切る ブレーキやアクセルを踏む 詰まるところ 2つの数値に集約できます 難しくはなさそうでしょう?
2009年に取り組み始めた頃は こんな感じでした 私たちの車が中央にあり 道を走る他の車が 箱として描かれています 自分がどこにいて 他の車がどこにいるか おおよそ把握している必要があります 世界を幾何学的に理解するのです 街中を走行するようになって 問題は格段に難しくなりました 車の前を歩行者が横断したり 車が目の前を横切ったり 様々な方向に進みます 信号があり 横断歩道があります 前と比べて 遙かに複雑になっています それに対応できる ようになったら 今度は工事現場に 対応できなければいけません ここでは左側のロードコーンによって 右に移動させられています 工事現場そのものだけでなく その周囲を行く人にも 注意を払う必要があります 交通違反があれば警察が来ます 屋根に点滅する ライトが付いている車は ただの車ではなく警察車両だと 理解できる必要があります 道路の端にいる オレンジ色の箱は スクールバスです これも特別な扱いを 必要とします
道路上の人々には それぞれ期待することがあります 自転車の人が手を挙げているのは 車線変更したいので 道を譲ってほしいということです 警官が路上に立って こんなポーズをしていたら 止まれという意味だと 理解する必要があり 進めという合図をしたら 進む必要があります
このために私たちの取っている方法は 車同士で情報共有するということです 最初の荒削りなモデルでは 1台が工事現場を見つけたら 他の車にも知らせ 車線変更して問題を回避できるようにする というものでした しかし私たちはもっと 深い理解をしています これまで観察してきた 他の車のデータ ― 何十万という歩行者 自転車 自動車のデータから それぞれがどんな姿を しているか理解し それを元に 他の車や歩行者が どう見えるか推測します さらに重要なのは それぞれがどう動くと予想されるかという モデルを作れたことです ここで黄色い箱は 前を横切る歩行者です 青い箱は自転車で こちらの車を右に避けると 予想しています 向こうからやってくる自転車は 道に沿って進んでいくだろうと 予想できます ここでは車が右折していて こちらでは 目の前に Uターンしようとしている車がいて その動きを予期し それに応じて安全な動きをします
見たことのあるものばかりなら 良いのですが 現実の世界では 見たことのないものにも 出くわします これはほんの2ヶ月前に マウンテンビューを 走行していて 出会ったものですが 電動車椅子の女性が 道の真ん中で ぐるぐる鴨を 追いかけていたんです (笑) 陸運局のマニュアルの どこを見ても この状況にどう対処すべきか 書いてありません しかし私たちの車は この時 スピードを落として 無事やり過ごせました 対応しなければならないのは 鴨ばかりではありません 鳥が突然前に飛び出しましたが 車はちゃんと対応しています ここではマウンテンビュー以外では およそお目にかからないような 自転車に対応しています もちろん他の車にも 対応する必要があります こんなミニサイズのものまで含めて 右手を見てください 誰かトラックから降りてきます 左にいる車の緑の箱が ぎりぎりになって 右折してきます 車線変更しようとしたら 左手の車も同時に 車線変更してきました 車が赤信号を 突っ込んでくるので 道を譲っています こちらでは自転車が 信号無視して入ってきます もちろん 危険がないように対応します そして道路では時々 理解できないことをする人たちがいます 2台の自動運転車の間に 真横から車が入ってきました 「何考えてんだ?」と 言いたくなるでしょう (笑)
様々なケースを 立て続けにお見せしたので 1つのケースを 少しだけ細かく見てみましょう 先ほどの自転車のケースですが 下の映像で分かるように この自転車はまだ視界に入っていません しかし車は自転車を把握しています 左端の青い箱です レーザーを使って 捉えたものです これは少しわかりにくいので 向きを変えてレーザーによるデータを よく見てみましょう 目をこらして見ると 道の角にいくつか点があり この部分ですが 青い箱が自転車を示しています こちらの信号は赤ですが 自転車の信号は既に 黄色になっています 映像をよく見ると分かります しかし自転車は 交差点に入ってきます こちらの信号が青に変わり 向こうは赤になっていますが この自転車が道を横切ってくることを 予期しています あいにく隣の車は我々ほど 注意を払っていなかったため 進み始めます 幸い自転車はうまくよけて 交差点を渡りきりました これで前に進めます
ご覧いただいたように この技術はとても素晴らしい進歩を遂げ 市場に送り出せると 強い自信を持っています 日々行っているシミュレーターによるテスト走行は 500万キロにも及びます この車がどれほど経験を積んできたか お分かりになるでしょう 私たちはこの技術を道路にもたらせる日を 待ち焦がれています 運転者支援システムではなく 自動運転車が正しい道であると 信じています これはとても緊急性の 高い問題なんです この講演をしている間にも アメリカでは34人が 交通事故で死んでいる計算です
これを世に出せるのは いつになるのか? 非常に難しい問題なので 確答するは難しいです この写真は 私の2人の息子です 長男は11歳で それはあと4年半で運転免許を 取れるようになるということです 私たちのチームでは そんなことにならないよう 全力を尽くしています (笑)
ありがとうございました
(拍手) (クリス・アンダーソン) 1つ質問していいですか
(クリス・アームソン) どうぞ
(アンダーソン) あなた方の車の能力は 驚くばかりです 運転支援システムか完全な自動運転車か という点については 現在さかんに議論が行われています テスラのような会社は 運転支援システムの道を選んでいます あなたのお話では それでは行き詰まり 運転支援システムを改善していっても 自動運転車には到らないということでした 運転している人が 「大丈夫なようだ」と思って 後ろの方を向いて まずい事態になるだろうと
(アームソン) その通りです 運転支援システムなど価値がないと 言うのではありません 過渡的には多くの命を 救うことができるでしょう しかしスティーブのような盲目の 人がどこにでも行けるようにするとか 安全性を究極まで高めるとか 駐車場所を車が自分で 見つけに行くことで 都心から駐車場という名のクレーターをなくすといった 抜本的な変化を目指すなら 自動運転車が唯一の道です
(アンダーソン) 大きな興味を持って 今後を見守っています (アームソン) ありがとうございます (拍手)
品詞分類
- 主語
- 動詞
- 助動詞
- 準動詞
- 関係詞等
TED 日本語
TED Talks
関連動画
フォーミュラ1が赤ちゃんを救う?ピーター・ヴァン・マネン
2013.08.013Dプリンタで作るジャンボ・ジェット機?バスティアン・シェーファー
2013.07.29もし車が話せたら事故は避けられるジェニファー・ヒーリー
2013.04.25原付スケートボードサンジェイ・ダストゥア
2013.04.02未来のレーシングカークリス・ガーデス
2012.07.11道路を走れる飛行機をつくるアナ・ムラチェク・ディートリック
2011.11.02視覚障害者が運転できる車を作るデニス・ホン
2011.06.03Googleの自動運転車で目指していることセバスチャン・スラン
2011.03.31スーパー電気バイクのデザインイヴ・べアール
2009.05.22シャイ・アガシの電気自動車に対する大胆な計画
2009.04.13
洋楽 おすすめ
RECOMMENDS
洋楽歌詞
ダイナマイトビーティーエス
洋楽最新ヒット2020.08.20ディス・イズ・ミーグレイテスト・ショーマン・キャスト
洋楽人気動画2018.01.11グッド・ライフGイージー、ケラーニ
洋楽人気動画2017.01.27ホワット・ドゥ・ユー・ミーン?ジャスティン・ビーバー
洋楽人気動画2015.08.28ファイト・ソングレイチェル・プラッテン
洋楽人気動画2015.05.19ラヴ・ミー・ライク・ユー・ドゥエリー・ゴールディング
洋楽人気動画2015.01.22アップタウン・ファンクブルーノ・マーズ、マーク・ロンソン
洋楽人気動画2014.11.20ブレイク・フリーアリアナ・グランデ
洋楽人気動画2014.08.12ハッピーファレル・ウィリアムス
ポップス2014.01.08カウンティング・スターズワンリパブリック
ロック2013.05.31ア・サウザンド・イヤーズクリスティーナ・ペリー
洋楽人気動画2011.10.26ユー・レイズ・ミー・アップケルティック・ウーマン
洋楽人気動画2008.05.30ルーズ・ユアセルフエミネム
洋楽人気動画2008.02.21ドント・ノー・ホワイノラ・ジョーンズ
洋楽人気動画2008.02.15オンリー・タイムエンヤ
洋楽人気動画2007.10.03ミス・ア・シングエアロスミス
ロック2007.08.18タイム・トゥ・セイ・グッバイサラ・ブライトマン
洋楽人気動画2007.06.08シェイプ・オブ・マイ・ハートスティング
洋楽人気動画2007.03.18ウィ・アー・ザ・ワールド(U.S.A. フォー・アフリカ)マイケル・ジャクソン
洋楽人気動画2006.05.14ホテル・カリフォルニアイーグルス
ロック2005.07.06