
TED日本語
TED Talks(英語 日本語字幕付き動画)
TED日本語 - ローラ・シュルツ: 驚くほど論理的な、赤ちゃんの心
TED Talks
驚くほど論理的な、赤ちゃんの心
The surprisingly logical minds of babies
ローラ・シュルツ
Laura Schulz
内容
赤ちゃんはどうやって、わずかな材料から多くのことを非常に速く学ぶのでしょうか。認知科学者ローラ・シュルツは、面白い話の中で多くの実験を紹介しながら、赤ちゃんたちがしゃべり始めるずっと前から、驚くほど強力な論理感覚を駆使して決断を下す姿を明らかにしていきます。
字幕
SCRIPT
Script
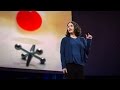
Mark Twain summed up what I take to be one of the fundamental problems of cognitive science with a single witticism. He said, "There's something fascinating about science. One gets such wholesale returns of conjecture out of such a trifling investment in fact." (Laughter)
Twain meant it as a joke, of course, but he's right: There's something fascinating about science. From a few bones, we infer the existence of dinosuars. From spectral lines, the composition of nebulae. From fruit flies, the mechanisms of heredity, and from reconstructed images of blood flowing through the brain, or in my case, from the behavior of very young children, we try to say something about the fundamental mechanisms of human cognition. In particular, in my lab in the Department of Brain and Cognitive Sciences at MIT, I have spent the past decade trying to understand the mystery of how children learn so much from so little so quickly. Because, it turns out that the fascinating thing about science is also a fascinating thing about children, which, to put a gentler spin on Mark Twain, is precisely their ability to draw rich, abstract inferences rapidly and accurately from sparse, noisy data. I'm going to give you just two examples today. One is about a problem of generalization, and the other is about a problem of causal reasoning. And although I'm going to talk about work in my lab, this work is inspired by and indebted to a field. I'm grateful to mentors, colleagues, and collaborators around the world.
Let me start with the problem of generalization. Generalizing from small samples of data is the bread and butter of science. We poll a tiny fraction of the electorate and we predict the outcome of national elections. We see how a handful of patients responds to treatment in a clinical trial, and we bring drugs to a national market. But this only works if our sample is randomly drawn from the population. If our sample is cherry-picked in some way -- say, we poll only urban voters, or say, in our clinical trials for treatments for heart disease, we include only men -- the results may not generalize to the broader population.
So scientists care whether evidence is randomly sampled or not, but what does that have to do with babies? Well, babies have to generalize from small samples of data all the time. They see a few rubber ducks and learn that they float, or a few balls and learn that they bounce. And they develop expectations about ducks and balls that they're going to extend to rubber ducks and balls for the rest of their lives. And the kinds of generalizations babies have to make about ducks and balls they have to make about almost everything: shoes and ships and sealing wax and cabbages and kings.
So do babies care whether the tiny bit of evidence they see is plausibly representative of a larger population? Let's find out. I'm going to show you two movies,one from each of two conditions of an experiment, and because you're going to see just two movies, you're going to see just two babies, and any two babies differ from each other in innumerable ways. But these babies, of course, here stand in for groups of babies, and the differences you're going to see represent average group differences in babies' behavior across conditions. In each movie, you're going to see a baby doing maybe just exactly what you might expect a baby to do, and we can hardly make babies more magical than they already are. But to my mind the magical thing, and what I want you to pay attention to, is the contrast between these two conditions, because the only thing that differs between these two movies is the statistical evidence the babies are going to observe. We're going to show babies a box of blue and yellow balls, and my then-graduate student, now colleague at Stanford, Hyowon Gweon, is going to pull three blue balls in a row out of this box, and when she pulls those balls out, she's going to squeeze them, and the balls are going to squeak. And if you're a baby, that's like a TED Talk. It doesn't get better than that. (Laughter) But the important point is it's really easy to pull three blue balls in a row out of a box of mostly blue balls. You could do that with your eyes closed. It's plausibly a random sample from this population. And if you can reach into a box at random and pull out things that squeak, then maybe everything in the box squeaks. So maybe babies should expect those yellow balls to squeak as well. Now, those yellow balls have funny sticks on the end, so babies could do other things with them if they wanted to. They could pound them or whack them. But let's see what the baby does.
(Video) Hyowon Gweon: See this? (Ball squeaks) Did you see that? (Ball squeaks) Cool. See this one? (Ball squeaks) Wow.
Laura Schulz: Told you. (Laughs)
(Video) HG: See this one? (Ball squeaks) Hey Clara, this one's for you. You can go ahead and play. (Laughter)
LS: I don't even have to talk, right? All right, it's nice that babies will generalize properties of blue balls to yellow balls, and it's impressive that babies can learn from imitating us, but we've known those things about babies for a very long time. The really interesting question is what happens when we show babies exactly the same thing, and we can ensure it's exactly the same because we have a secret compartment and we actually pull the balls from there, but this time, all we change is the apparent population from which that evidence was drawn. This time, we're going to show babies three blue balls pulled out of a box of mostly yellow balls, and guess what? You [ probably won't ] randomly draw three blue balls in a row out of a box of mostly yellow balls. That is not plausibly randomly sampled evidence. That evidence suggests that maybe Hyowon was deliberately sampling the blue balls. Maybe there's something special about the blue balls. Maybe only the blue balls squeak. Let's see what the baby does.
(Video) HG: See this? (Ball squeaks) See this toy? (Ball squeaks) Oh, that was cool. See? (Ball squeaks) Now this one's for you to play. You can go ahead and play.
LS: So you just saw two 15-month-old babies do entirely different things based only on the probability of the sample they observed. Let me show you the experimental results. On the vertical axis, you'll see the percentage of babies who squeezed the ball in each condition, and as you'll see, babies are much more likely to generalize the evidence when it's plausibly representative of the population than when the evidence is clearly cherry-picked. And this leads to a fun prediction: Suppose you pulled just one blue ball out of the mostly yellow box. You [ probably won't ] pull three blue balls in a row at random out of a yellow box, but you could randomly sample just one blue ball. That's not an improbable sample. And if you could reach into a box at random and pull out something that squeaks, maybe everything in the box squeaks. So even though babies are going to see much less evidence for squeaking, and have many fewer actions to imitate in this one ball condition than in the condition you just saw, we predicted that babies themselves would squeeze more, and that's exactly what we found. So 15-month-old babies, in this respect, like scientists, care whether evidence is randomly sampled or not, and they use this to develop expectations about the world: what squeaks and what doesn't, what to explore and what to ignore.
Let me show you another example now, this time about a problem of causal reasoning. And it starts with a problem of confounded evidence that all of us have, which is that we are part of the world. And this might not seem like a problem to you, but like most problems, it's only a problem when things go wrong. Take this baby, for instance. Things are going wrong for him. He would like to make this toy go, and he can't. I'll show you a few-second clip. And there's two possibilities, broadly: Maybe he's doing something wrong, or maybe there's something wrong with the toy. So in this next experiment, we're going to give babies just a tiny bit of statistical data supporting one hypothesis over the other, and we're going to see if babies can use that to make different decisions about what to do.
Here's the setup. Hyowon is going to try to make the toy go and succeed. I am then going to try twice and fail both times, and then Hyowon is going to try again and succeed, and this roughly sums up my relationship to my graduate students in technology across the board. But the important point here is it provides a little bit of evidence that the problem isn't with the toy, it's with the person. Some people can make this toy go, and some can't. Now, when the baby gets the toy, he's going to have a choice. His mom is right there, so he can go ahead and hand off the toy and change the person, but there's also going to be another toy at the end of that cloth, and he can pull the cloth towards him and change the toy. So let's see what the baby does.
(Video) HG: Two,three. Go! (Music) LS: One,two,three, go! Arthur, I'm going to try again. One,two,three, go! YG: Arthur, let me try again, okay? One,two,three, go! (Music) Look at that. Remember these toys? See these toys? Yeah, I'm going to put this one over here, and I'm going to give this one to you. You can go ahead and play. LS: Okay, Laura, but of course, babies love their mommies. Of course babies give toys to their mommies when they can't make them work. So again, the really important question is what happens when we change the statistical data ever so slightly. This time, babies are going to see the toy work and fail in exactly the same order, but we're changing the distribution of evidence. This time, Hyowon is going to succeed once and fail once, and so am I. And this suggests it doesn't matter who tries this toy, the toy is broken. It doesn't work all the time. Again, the baby's going to have a choice. Her mom is right next to her, so she can change the person, and there's going to be another toy at the end of the cloth. Let's watch what she does.
(Video) HG: Two,three, go! (Music) Let me try one more time. One,two,three, go! Hmm.
LS: Let me try, Clara. One,two,three, go! Hmm, let me try again. One,two,three, go! (Music) HG: I'm going to put this one over here, and I'm going to give this one to you. You can go ahead and play. (Applause)
LS: Let me show you the experimental results. On the vertical axis, you'll see the distribution of children's choices in each condition, and you'll see that the distribution of the choices children make depends on the evidence they observe. So in the second year of life, babies can use a tiny bit of statistical data to decide between two fundamentally different strategies for acting in the world: asking for help and exploring. I've just shown you two laboratory experiments out of literally hundreds in the field that make similar points, because the really critical point is that children's ability to make rich inferences from sparse data underlies all the species-specific cultural learning that we do. Children learn about new tools from just a few examples. They learn new causal relationships from just a few examples. They even learn new words, in this case in American Sign Language.
I want to close with just two points. If you've been following my world, the field of brain and cognitive sciences, for the past few years,three big ideas will have come to your attention. The first is that this is the era of the brain. And indeed, there have been staggering discoveries in neuroscience: localizing functionally specialized regions of cortex, turning mouse brains transparent, activating neurons with light. A second big idea is that this is the era of big data and machine learning, and machine learning promises to revolutionize our understanding of everything from social networks to epidemiology. And maybe, as it tackles problems of scene understanding and natural language processing, to tell us something about human cognition. And the final big idea you'll have heard is that maybe it's a good idea we're going to know so much about brains and have so much access to big data, because left to our own devices, humans are fallible, we take shortcuts, we err, we make mistakes, we're biased, and in innumerable ways, we get the world wrong. I think these are all important stories, and they have a lot to tell us about what it means to be human, but I want you to note that today I told you a very different story. It's a story about minds and not brains, and in particular, it's a story about the kinds of computations that uniquely human minds can perform, which involve rich, structured knowledge and the ability to learn from small amounts of data, the evidence of just a few examples. And fundamentally, it's a story about how starting as very small children and continuing out all the way to the greatest accomplishments of our culture, we get the world right.
Folks, human minds do not only learn from small amounts of data. Human minds think of altogether new ideas. Human minds generate research and discovery, and human minds generate art and literature and poetry and theater, and human minds take care of other humans: our old, our young, our sick. We even heal them. In the years to come, we're going to see technological innovations beyond anything I can even envision, but we are very unlikely to see anything even approximating the computational power of a human child in my lifetime or in yours. If we invest in these most powerful learners and their development, in babies and children and mothers and fathers and caregivers and teachers the ways we invest in our other most powerful and elegant forms of technology, engineering and design, we will not just be dreaming of a better future, we will be planning for one.
Thank you very much.
(Applause)
Chris Anderson: Laura, thank you. I do actually have a question for you. First of all, the research is insane. I mean, who would design an experiment like that? (Laughter) I've seen that a couple of times, and I still don't honestly believe that that can truly be happening, but other people have done similar experiments; it checks out. The babies really are that genius.
LS: You know, they look really impressive in our experiments, but think about what they look like in real life, right? It starts out as a baby. Eighteen months later, it's talking to you, and babies' first words aren't just things like balls and ducks, they're things like "all gone," which refer to disappearance, or "uh-oh," which refer to unintentional actions. It has to be that powerful. It has to be much more powerful than anything I showed you. They're figuring out the entire world. A four-year-old can talk to you about almost anything. (Applause)
CA: And if I understand you right, the other key point you're making is, we've been through these years where there's all this talk of how quirky and buggy our minds are, that behavioral economics and the whole theories behind that that we're not rational agents. You're really saying that the bigger story is how extraordinary, and there really is genius there that is underappreciated.
LS: One of my favorite quotes in psychology comes from the social psychologist Solomon Asch, and he said the fundamental task of psychology is to remove the veil of self-evidence from things. There are orders of magnitude more decisions you make every day that get the world right. You know about objects and their properties. You know them when they're occluded. You know them in the dark. You can walk through rooms. You can figure out what other people are thinking. You can talk to them. You can navigate space. You know about numbers. You know causal relationships. You know about moral reasoning. You do this effortlessly, so we don't see it, but that is how we get the world right, and it's a remarkable and very difficult-to-understand accomplishment.
CA: I suspect there are people in the audience who have this view of accelerating technological power who might dispute your statement that never in our lifetimes will a computer do what a three-year-old child can do, but what's clear is that in any scenario, our machines have so much to learn from our toddlers. LS: I think so. You'll have some machine learning folks up here. I mean, you should never bet against babies or chimpanzees or technology as a matter of practice, but it's not just a difference in quantity, it's a difference in kind. We have incredibly powerful computers, and they do do amazingly sophisticated things, often with very big amounts of data. Human minds do, I think, something quite different, and I think it's the structured, hierarchical nature of human knowledge that remains a real challenge.
CA: Laura Schulz, wonderful food for thought. Thank you so much.
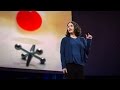
マーク・トウェインは 私が 認知科学の本質的な問題の 1つと考えることを 機知に富む言葉でまとめています 「科学には魅力的な面がある 事実に対して ほんのわずかに投資するだけで これほど大量に 予想という利益を得るのだから」 (笑)
これはジョークでしょうが 彼の言葉は的を射ています 科学には魅力的な面があります わずか数本の骨から 恐竜の存在を推測し スペクトル線から 銀河の組成を推測します ショウジョウバエからは 遺伝のメカニズムを そして脳の血流を再現した画像や 私の場合なら 小さな子どもの行動から 人間の認知の 本質的なメカニズムについて 説明しようとするのです 私は その中でも MIT脳認知科学科の研究室で 過去10年に渡って ある謎を解こうとしてきました 子どもはどうやって わずかな情報から これほど速く学ぶのかということです その理由は 科学がもつ魅力は 子どもたちがもつ魅力と 同じものだからです つまり トウェインの言葉を 少しひねって言えば 子どもたちは わずかな量の ノイズだらけのデータから 速く正確に 豊かな抽象的推論をする 能力をもっているからです 今日は その例を2つ紹介しましょう ひとつは「一般化」の問題 ― もうひとつは 「因果的思考」の問題です お話しするのは 私の研究室で行っていることですが これは ある研究分野に 動機付けられ 多くを負っています 世界中の恩師や同僚や 協力者に感謝しています
まず「一般化」の問題から はじめましょう 抽出したデータの標本から 一般化することは科学の基本です 国政選挙では 有権者のほんの一部から 聞き取り調査をして 結果を予測します 臨床試験では数人の被験者に 治療の効果があるかを調べた上で 薬を市場に出します ただ これが上手くいくのは標本が 母集団から無作為に抽出された時だけです 標本の抽出方法が偏っている場合 たとえば ― 都市部の有権者だけ調査した場合や 心臓病治療の臨床試験で 被験者が男性だけの場合には 結果を より広範な集団に 一般化できない可能性があります
だから科学者にとって データが 無作為抽出かどうかは重要です ただ それが赤ちゃんと どう結びつくのでしょうか? 赤ちゃんは 常に少数の標本から 一般化せざるを得ません ゴムのアヒルをいくつか見て それが浮くことを学び ボールをいくつか見て それが弾むことを学びます こうして赤ちゃんは アヒルやボールについて予想を立て その予想を生涯に渡って 当てはめ続けるのです そして 赤ちゃんが アヒルとボールに対して行う一般化は 他のほぼあらゆるもの すなわち 靴にも 船にも 封蝋にも キャベツにも 王様にも適用する必要があります
では 赤ちゃんにとって 自分が見た わずかな証拠が より大きな集団の典型例かどうかは 重要なのでしょうか? 調べてみましょう これから見ていただく2本のビデオは ある実験での 2つの条件のものです ご覧いただくのは2本だけなので 赤ちゃんは2人しか登場しません どの赤ちゃんにも あらゆる面で違いがありますが この2人の赤ちゃんは 集団を代表していて これから見ていただく違いは それぞれの条件における 赤ちゃんの 標準的な行動の違いを表しています ビデオでは赤ちゃんが いかにも赤ちゃんらしく 振舞っていて これ以上 可愛く見せることなんて できないくらいです ただ私にとって魅力的なこと ― そして皆さんに注目してほしいことは 2つの条件における違いです というのも 2本のビデオで 唯一 違う点は 赤ちゃんが目にする 統計的な証拠だけなのです 私たちは 赤ちゃんに 青と黄色のボールが入った箱を見せ 当時 大学院生だった スタンフォードの同僚ユウァン・グァンが 青いボールを3つ続けて取り出します そして取り出すたびに ボールを握ると 音が鳴ります これは赤ちゃんにとって TEDトークと同じように 最高の体験なんです (笑) さて 重要なのは ほぼ青いボールしか入っていない箱から 青を3つ続けて取るのは 簡単だという点です 見なくてもできるかもしれません これは母集団からの無作為抽出と 言っていいでしょう そして 箱に手を入れて 無作為に音が鳴るものを取り出せるなら 箱の中のボールは みんな音が出るかもしれません だから赤ちゃんは 黄色のボールも音が鳴ると思うはずです ただし黄色のボールには ヘンな棒がついていて やろうと思えば 他のことだってできます トントン打ったり 叩いたりしてもいいのです では赤ちゃんの行動を見てみましょう
(ユウァン・グァン)見える? (ボールが鳴る) 今の見た? (ボールが鳴る) 素敵ね これはどう? (ボールが鳴る) わあ
(ローラ・シュルツ) 可愛いでしょう (笑)
(ユウァン)これはどう? (ボールが鳴る) クララ これはあなたにあげる 遊んでいいわよ (笑)
(ローラ)言葉は必要ないですね さて 赤ちゃんが 青いボールの性質を 黄色のボールへと 一般化する様子は素晴らしいし 赤ちゃんが私たちの真似をして 学ぶのは すごいことです ただ このような赤ちゃんの能力は 昔から知られていました 一方 本当に興味深いのは 赤ちゃんに まったく同じものを 見せると どうなるかです 箱には見えない仕切りがあり 実際には そこからボールを取るので 条件はまったく同じになりますが 今度は標本を取り出す ― 母集団の見た目だけを変えます 今度は赤ちゃんに ほぼ黄色のボールしか入っていない箱から 青いボールを 3つ取るところを見せると どうなるでしょう? ほとんど黄色だけの箱から 無作為で青いボールを 3つ連続で取ることは まずないでしょう つまり無作為に抽出された データとは言えないのです この場合 ユウァンが意図的に 青いボールだけ取ったことを示しています あるいは 青いボールが 特別なのかもしれません 鳴るのは青だけかもしれません さあ赤ちゃんはどうするでしょうか
(ユウァン)見える? (ボールが鳴る) このおもちゃが見える? (ボールが鳴る) 素敵でしょう? (ボールが鳴る) さあ あなたはこれで遊んで さあ遊んでね
(不満の表情) (笑)
(ローラ)さて皆さんには 15か月になる2人の赤ちゃんが 観察した確率だけにもとづいて まったく違う行動をとる様子を 見ていただきました 実験結果をご覧ください 縦軸は それぞれの条件で ボールを握った赤ちゃんの 割合を表しています ご覧の通り データの選び方が 明らかに偏っている場合より 母集団の代表とみなせる場合の方が そのデータを一般化する傾向が はるかに強いのです ここから 面白い予想を立てられます 今度は ほぼ黄色ばかりの箱から 青いボールを1つだけ取るとします この箱から青いボールを無作為に 3つ連続で取り出すのは無理でしょうが 1つだけなら取り出せるかもしれません 標本として ありえる話です さらに 箱に手を入れて無作為で 音が鳴るものを取り出せるなら 箱の中身は全部鳴るかもしれません つまり ボールを1つだけ取り出す場合 先ほどのビデオの場合と比べて 音が鳴る例や 真似すべき行動を 赤ちゃんが わずかな回数しか 見ていなくても ボールを握ることが 多くなると予想することができ 結果は まさに その通りでした したがって このような場面では 15か月の赤ちゃんにとって データが無作為に抽出されたかは 科学者と同様に重要で それをもとに世界について 予想を立てているのです どれが音が鳴って どれが鳴らないか 何を調べるべきで 何を無視すべきか・・・
次に別の例を紹介しましょう 今度は「因果的思考」の問題です この問題は 私たち全員が経験する データの混乱の問題 すなわち 人間が世界の一部であることから生じます 一見 問題とは思えないでしょうが ほとんどの問題と同様に 何かがうまくいかない時 はじめて問題になります 例えば この赤ちゃん ― 彼にとって 物事はうまくいきません おもちゃを鳴らしたいのに できないのです 数秒のビデオを ご覧ください 大まかに可能性は2つあります 間違ったことをしているか おもちゃに問題があるか です ですから次の実験では 赤ちゃんに片方の仮説を支持する ちょっとした統計的データを見せます その後 赤ちゃんが 自分の行動を決定する時に そのデータを使うか 観察するのです
仕組みはこうです ユウァンがおもちゃを 鳴らそうとして成功します 次に私が2回試して 2回とも失敗します その後ユウァンが また試して成功します これはテクノロジー全般に関する 院生たちと私の関係を 表していますね ただ ここで重要なのは おもちゃではなく 人のほうに 問題があるということを 証拠として示している点です おもちゃを鳴らせる人と 鳴らせない人が いるのです さて おもちゃを手にした時 赤ちゃんは選択することになります 近くにお母さんがいるので おもちゃを渡して 代わりにやってもらえます 一方 布の端には 別のおもちゃがあり 布を引けば おもちゃを 取り替えることもできます 赤ちゃんがどうするか 見てみましょう
(ユウァン)2 3 ゴー! (音楽) (ローラ)1 2 3 ゴー! アーサー もう一度やってみるわね 1 2 3 ゴー! (ユウァン)アーサー もう一度 私にやらせて 1 2 3 ゴー! (音楽) これを見て これ覚えてる? おもちゃよ これは こっちに置いて これは あなたにあげるわ 遊んでね (ローラ)当然 赤ちゃんは お母さんのことが大好きです おもちゃが鳴らなければ 当たり前のように お母さんに渡します でも ここで重要な点は 統計的データを わずかに変えたら どうなるかということです 今度は 同じ順でおもちゃが鳴ったり 鳴らなかったりするところを見せますが 証拠の分布は変えます 今度はユウァンが成功した後 失敗し 私の時も同じにします ここからわかるのは 使う人は無関係で おもちゃが壊れており 常に鳴るとは限らないということです 今度も赤ちゃんには選択肢があります お母さんは隣にいるので 代わりをしてもらうこともできるし 布の端には別のおもちゃもあります どうするか見てみましょう
(ユウァン)2 3 ゴー! (音楽) もう一度やらせて 1 2 3 ゴー! うーん
(ローラ)私にやらせて クララ 1 2 3 ゴー! もう一度やらせて 1 2 3 ゴー! (音楽) (ユウァン)これは こっちに置いて これは あなたにあげるわ 遊んでね (拍手)
(ローラ)実験の結果をお見せします 縦軸には それぞれの条件における 子どもたちの選択の分布を示しています 選択の分布は 赤ちゃんが目にした データに基づいているのが わかるでしょう 赤ちゃんたちは生まれて2年で わずかな統計的なデータを利用して 世界で行動するために 根本的に異なる2つの戦略から 1つ選ぶのです つまり 助けを求めるか 自分で試すかです 研究室での実験を 2つだけお見せしましたが まさに何百もの研究で 同様の結果が出ています そして本当に重要な点は わずかなデータから 豊かに推測するという子どもの能力が 人間に特有の あらゆる文化的学習の 根底にあることです 子どもたちは わずかな例をもとに 新たな道具について学び わずかな例をもとに 新たな因果関係を学びます 新たな言葉さえ学びます これはアメリカ手話です
最後に2点だけお話しします もし私の世界 すなわち 脳科学と認知科学の分野を ここ数年 追いかけていれば 3つの壮大な発想に 気づくでしょう 1つは現在が「脳の時代」だという点です 実際 神経科学の分野では 驚異的な発見が続いています 機能ごとに特化した 大脳皮質の領域の特定や マウスの脳の透明化 光によるニューロンの活性化などです 2つ目の壮大な発想とは 現代が「ビッグデータと 機械学習の時代」だという点です 機械学習は SNSから疫学まで ― あらゆるものの理解を 大きく変えるはずです そして機械が 場面認識や 自然言語処理といった 課題に取り組むことで 人間の認知について わかってくるかもしれません そして最後の壮大な発想とは 脳への理解を深めていき ビッグデータを十分 利用できるのは いいことかもしれないという点です なぜなら人間は 好きなようにさせておくと 誤りがちで 楽をしようとし 判断を誤り 間違いを犯し 偏った見方をし さらにあらゆる面で 世界を間違って捉えてしまいます 私は これらすべてが 重要な事だと思っていて そこから人間であることの意味を いろいろ知ることができます ただ注意してほしいのですが 今日の話は それとは別のことです 今日の話題は 脳のことではなく 心についてです その中でも 人間の心だけが実行できる 様々な計算に関する話です この計算には 豊かで構造化された知識と わずかなデータ 少ない例から得た 証拠をもとに学ぶ能力が伴います 今日の話の本質は どうやって 赤ちゃんに始まり 遥かな道をたどって 文化という偉業を成し遂げる中で 私たちが世界を 正しく捉えているかということです
人間の心は 単にわずかなデータから 学習するだけではありません 心はまったく新しい発想も生み出します 心が研究や発見を生み 芸術や 文学や 詩や 劇を生み 心が お年寄りや若者や 病んだ人々を思いやるのです 私たちは そんな人々を癒しさえします 今後 数年で私たちは 想像をはるかに超えた 技術的な革新を 目の当たりにすることでしょう でも とても残念なことに 私や皆さんが生きている間には 人間の子どもの計算能力に 近いものすら 目にすることはないでしょう 私たちは この極めて優れた学習者の 発達に対する投資を惜しんではいけません 赤ちゃんや子どもたち ― 母親や父親 ― 子どもの世話をする人々や 教師への投資を惜しんではいけません 私たちは 他の極めて優れた エレガントな技術や工学やデザインには 投資を惜しみませんが 同じように投資することで よりよい未来を ただ夢見るだけではなく そんな未来の青写真を描けるはずです
ありがとうございます
(拍手)
(クリス・アンダーソン)ありがとう ローラ ぜひ 尋ねたいことがあります まず この研究は常識外れですね 一体誰が こんな実験方法を 考えるんですか? (笑) 私は実験の様子を2回見ても 本当に こんな風になるなんて 信じられません 一方 他の研究者たちも 同様の実験で実証しています 赤ちゃんは まさに天才ですね
(ローラ)実験での 赤ちゃん達は すごく優秀に見えますが 実際の赤ちゃんの様子を 思い出してください 最初は赤ん坊です 18か月経つと話し始めますが 初めて話す言葉には 「ボール」や「アヒル」だけではなく 失くなったことを表す「ない」や 意図せぬ行為を指す 「あれれ」まであります 彼らの能力は それほど強力ですし お見せしたものより はるかに強力なはずです 子どもは世界全体を理解していき 4歳になれば ほぼ何でも話せるようになるのです (拍手)
(クリス)私の理解が正しければ あなたの主張には他に重要な点があります ここ数年 言われ続けているのは 人間の心が いかに気まぐれで 誤りが多いか そして行動経済学と その背景にある理論によれば 人間は理性的主体ではないという点です あなたが言おうとしている より大きな物語とは まだ正当に評価されていない才能があり それが いかに素晴らしいかということですね
(ローラ)心理学の分野で 私が好きな言葉は 社会心理学者S・アッシュのものです 彼によると 心理学の作業の本質とは 事物から自明という名の ベールを取り除くことです 人間は 世界を正しく捉えるために 日々 膨大な回数の 決断を下します 人は物体や その性質を知っていて 見えなくても 暗闇でも それが何かわかります 部屋を歩き回ることもできます 他の人の考えも分かりますし 彼らに話しかけることもできます 空間を移動でき 数について知っています 因果関係や 倫理的な推論について知っています こういうことは自然にできるので 気づきませんが 人間は こうやって世界を捉えます これは驚くべき偉業ですが 理解するのは とても難しいのです
(クリス)観客のみなさんの中には 技術は さらに加速すると考えていて 我々の世代で コンピュータに 3歳児と同じことをさせるのは 不可能だという主張に 反対の人もいるでしょう ただ はっきりしているのは どうなっていくにせよ 機械には 子どもから学ぶことが まだたくさんあるという点です (ローラ)そうですね ここには 機械学習の研究者もいるでしょう ただ 赤ちゃんや チンパンジーや テクノロジーを 単なる実践上の問題として 片付けるべきではありません それは ただ量的に 異なっているだけではなく 別の種類のものなのです 驚くほど強力なコンピュータは しばしば非常に大量のデータを使い 極めて洗練されたことを やってのけます 一方 人間の心は これとはまったく異なっていて 本当の課題として残るのは 構造的かつ階層的な 人間の知識の性質だと思います
(クリス)シュルツさん 考える材料になりました ありがとう
(ローラ)ありがとう (拍手)
品詞分類
- 主語
- 動詞
- 助動詞
- 準動詞
- 関係詞等
TED 日本語
TED Talks
関連動画
うつやPTSDを予防できる新種の薬 | TED Talkレベッカ・ブラックマン
2019.04.17騒音が健康に有害な理由 ― そして私たちにできることマティアス・バスナー
2019.02.26さまよう心を鎮めるには | TED Talkアミシ・ジャー
2018.04.18鬱の友達と心を通わせるにはビル・バーナット
2018.03.23脳に良い変化をもたらす運動の効果ウェンディー・スズキ
おすすめ 12018.03.21物事の「良し悪し」は思い込みに過ぎないヘザー・ラニエ
おすすめ 12018.01.19脳が深い睡眠から更なる恩恵を得る方法ダン・ガーテンバーグ
2018.01.04うつを一人で抱え込まないでニッキー・ウェバー・アレン
2017.10.26長寿の秘訣は周囲の人との交流かもスーザン・ピンカー
2017.09.04脳はどのように美しさを判定するか?アンジャン・チャタジー
2017.08.22脳が「意識された現実」という幻覚を作り出す仕組みアニル・セス
2017.07.18注意を向けた時、脳では何が起きているのかメディ・オディカニ=セイドラー
2017.07.12ティーンエイジャーの登校時間を遅らせるべき理由とはウェンディ・トロクセル
2017.06.09死に直面したとき、人生に生きる価値を与えてくれるのはルーシー・カラニシ
おすすめ 12017.06.07最善の自己と最悪の自己の生物学ロバート・サポルスキー
2017.05.31メンタルヘルスを気遣うのは恥ずかしくなんかないサング・デリ
2017.05.26
洋楽 おすすめ
RECOMMENDS
洋楽歌詞
ステイザ・キッド・ラロイ、ジャスティン・ビーバー
洋楽最新ヒット2021.08.20スピーチレス~心の声ナオミ・スコット
洋楽最新ヒット2019.05.23シェイプ・オブ・ユーエド・シーラン
洋楽人気動画2017.01.30フェイデッドアラン・ウォーカー
洋楽人気動画2015.12.03ウェイティング・フォー・ラヴアヴィーチー
洋楽人気動画2015.06.26シー・ユー・アゲインウィズ・カリファ
洋楽人気動画2015.04.06シュガーマルーン5
洋楽人気動画2015.01.14シェイク・イット・オフテイラー・スウィフト
ポップス2014.08.18オール・アバウト・ザット・ベースメーガン・トレイナー
ポップス2014.06.11ストーリー・オブ・マイ・ライフワン・ダイレクション
洋楽人気動画2013.11.03コール・ミー・メイビーカーリー・レイ・ジェプセン
洋楽人気動画2012.03.01美しき生命コールドプレイ
洋楽人気動画2008.08.04バッド・デイ~ついてない日の応援歌ダニエル・パウター
洋楽人気動画2008.05.14サウザンド・マイルズヴァネッサ・カールトン
洋楽人気動画2008.02.19イッツ・マイ・ライフボン・ジョヴィ
ロック2007.10.11アイ・ウォント・イット・ザット・ウェイバックストリート・ボーイズ
洋楽人気動画2007.09.14マイ・ハート・ウィル・ゴー・オンセリーヌ・ディオン
洋楽人気動画2007.07.12ヒーローマライア・キャリー
洋楽人気動画2007.03.21オールウェイズ・ラヴ・ユーホイットニー・ヒューストン
洋楽人気動画2007.02.19オネスティビリー・ジョエル
洋楽人気動画2005.09.16