
TED日本語
TED Talks(英語 日本語字幕付き動画)
TED日本語 - シーラ・ニーレンバーグ: 失明した網膜への新たな補綴治療
TED Talks
失明した網膜への新たな補綴治療
A prosthetic eye to treat blindness
シーラ・ニーレンバーグ
Sheila Nirenberg
内容
TEDMEDにて、特定の失明状態を抱える患者に対して視覚回復が望める大胆なアプローチを、シーラ・ニーレンバーグがご紹介します。補綴装置を視神経に直接つないで、網膜から電気信号を脳に送信するという方法です。
字幕
SCRIPT
Script
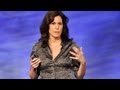
I study how the brain processes information. That is, how it takes information in from the outside world, and converts it into patterns of electrical activity, and then how it uses those patterns to allow you to do things -- to see, hear, to reach for an object. So I'm really a basic scientist, not a clinician, but in the last year and a half I've started to switch over, to use what we've been learning about these patterns of activity to develop prosthetic devices, and what I wanted to do today is show you an example of this. It's really our first foray into this. It's the development of a prosthetic device for treating blindness.
So let me start in on that problem. There are 10 million people in the U.S. and many more worldwide who are blind or are facing blindness due to diseases of the retina, diseases like macular degeneration, and there's little that can be done for them. There are some drug treatments, but they're only effective on a small fraction of the population. And so, for the vast majority of patients, their best hope for regaining sight is through prosthetic devices. The problem is that current prosthetics don't work very well. They're still very limited in the vision that they can provide. And so, you know, for example, with these devices, patients can see simple things like bright lights and high contrast edges, not very much more, so nothing close to normal vision has been possible.
So what I'm going to tell you about today is a device that we've been working on that I think has the potential to make a difference, to be much more effective, and what I wanted to do is show you how it works. Okay, so let me back up a little bit and show you how a normal retina works first so you can see the problem that we were trying to solve. Here you have a retina. So you have an image, a retina, and a brain. So when you look at something, like this image of this baby's face, it goes into your eye and it lands on your retina, on the front-end cells here, the photoreceptors. Then what happens is the retinal circuitry, the middle part, goes to work on it, and what it does is it performs operations on it, it extracts information from it, and it converts that information into a code. And the code is in the form of these patterns of electrical pulses that get sent up to the brain, and so the key thing is that the image ultimately gets converted into a code. And when I say code, I do literally mean code. Like this pattern of pulses here actually means "baby's face," and so when the brain gets this pattern of pulses, it knows that what was out there was a baby's face, and if it got a different pattern it would know that what was out there was, say, a dog, or another pattern would be a house. Anyway, you get the idea.
And, of course, in real life, it's all dynamic, meaning that it's changing all the time, so the patterns of pulses are changing all the time because the world you're looking at is changing all the time too. So, you know, it's sort of a complicated thing. You have these patterns of pulses coming out of your eye every millisecond telling your brain what it is that you're seeing. So what happens when a person gets a retinal degenerative disease like macular degeneration? What happens is is that, the front-end cells die, the photoreceptors die, and over time, all the cells and the circuits that are connected to them, they die too. Until the only things that you have left are these cells here, the output cells, the ones that send the signals to the brain, but because of all that degeneration they aren't sending any signals anymore. They aren't getting any input, so the person's brain no longer gets any visual information -- that is, he or she is blind.
So, a solution to the problem, then, would be to build a device that could mimic the actions of that front-end circuitry and send signals to the retina's output cells, and they can go back to doing their normal job of sending signals to the brain. So this is what we've been working on, and this is what our prosthetic does. So it consists of two parts, what we call an encoder and a transducer. And so the encoder does just what I was saying: it mimics the actions of the front-end circuitry -- so it takes images in and converts them into the retina's code. And then the transducer then makes the output cells send the code on up to the brain, and the result is a retinal prosthetic that can produce normal retinal output. So a completely blind retina, even one with no front-end circuitry at all, no photoreceptors, can now send out normal signals, signals that the brain can understand. So no other device has been able to do this.
Okay, so I just want to take a sentence or two to say something about the encoder and what it's doing, because it's really the key part and it's sort of interesting and kind of cool. I'm not sure "cool" is really the right word, but you know what I mean. So what it's doing is, it's replacing the retinal circuitry, really the guts of the retinal circuitry, with a set of equations, a set of equations that we can implement on a chip. So it's just math. In other words, we're not literally replacing the components of the retina. It's not like we're making a little mini-device for each of the different cell types. We've just abstracted what the retina's doing with a set of equations. And so, in a way, the equations are serving as sort of a codebook. An image comes in, goes through the set of equations, and out comes streams of electrical pulses, just like a normal retina would produce.
Now let me put my money where my mouth is and show you that we can actually produce normal output, and what the implications of this are. Here are three sets of firing patterns. The top one is from a normal animal, the middle one is from a blind animal that's been treated with this encoder-transducer device, and the bottom one is from a blind animal treated with a standard prosthetic. So the bottom one is the state-of-the-art device that's out there right now, which is basically made up of light detectors, but no encoder. So what we did was we presented movies of everyday things -- people, babies, park benches, you know, regular things happening -- and we recorded the responses from the retinas of these three groups of animals. Now just to orient you, each box is showing the firing patterns of several cells, and just as in the previous slides, each row is a different cell, and I just made the pulses a little bit smaller and thinner so I could show you a long stretch of data.
So as you can see, the firing patterns from the blind animal treated with the encoder-transducer really do very closely match the normal firing patterns -- and it's not perfect, but it's pretty good -- and the blind animal treated with the standard prosthetic, the responses really don't. And so with the standard method, the cells do fire, they just don't fire in the normal firing patterns because they don't have the right code. How important is this? What's the potential impact on a patient's ability to see? So I'm just going to show you one bottom-line experiment that answers this, and of course I've got a lot of other data, so if you're interested I'm happy to show more. So the experiment is called a reconstruction experiment. So what we did is we took a moment in time from these recordings and asked, what was the retina seeing at that moment? Can we reconstruct what the retina was seeing from the responses from the firing patterns?
So, when we did this for responses from the standard method and from our encoder and transducer. So let me show you, and I'm going to start with the standard method first. So you can see that it's pretty limited, and because the firing patterns aren't in the right code, they're very limited in what they can tell you about what's out there. So you can see that there's something there, but it's not so clear what that something is, and this just sort of circles back to what I was saying in the beginning, that with the standard method, patients can see high-contrast edges, they can see light, but it doesn't easily go further than that. So what was the image? It was a baby's face. So what about with our approach, adding the code? And you can see that it's much better. Not only can you tell that it's a baby's face, but you can tell that it's this baby's face, which is a really challenging task. So on the left is the encoder alone, and on the right is from an actual blind retina, so the encoder and the transducer. But the key one really is the encoder alone, because we can team up the encoder with the different transducer.
This is just actually the first one that we tried. I just wanted to say something about the standard method. When this first came out, it was just a really exciting thing, the idea that you even make a blind retina respond at all. But there was this limiting factor, the issue of the code, and how to make the cells respond better, produce normal responses, and so this was our contribution. Now I just want to wrap up, and as I was mentioning earlier of course I have a lot of other data if you're interested, but I just wanted to give this sort of basic idea of being able to communicate with the brain in its language, and the potential power of being able to do that. So it's different from the motor prosthetics where you're communicating from the brain to a device. Here we have to communicate from the outside world into the brain and be understood, and be understood by the brain.
And then the last thing I wanted to say, really, is to emphasize that the idea generalizes. So the same strategy that we used to find the code for the retina we can also use to find the code for other areas, for example, the auditory system and the motor system, so for treating deafness and for motor disorders. So just the same way that we were able to jump over the damaged circuitry in the retina to get to the retina's output cells, we can jump over the damaged circuitry in the cochlea to get the auditory nerve, or jump over damaged areas in the cortex, in the motor cortex, to bridge the gap produced by a stroke.
I just want to end with a simple message that understanding the code is really, really important, and if we can understand the code, the language of the brain, things become possible that didn't seem obviously possible before. Thank you.
(Applause)
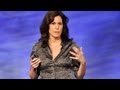
私は脳の情報処理系の 研究をしています 外界から受け取った情報を 電気活動の信号配列に変換することで 人は見たり 聞いたり ものに手を伸ばしたりと 行動を取っているのです 私は臨床医ではなく 基礎医学者なのですが この1年半で得た信号配列の 知識を応用して補綴装置の 開発を始めました 信号配列解析の応用例を お見せします これは失明に対して 補綴装置を利用する 私たちの初の試みです
失明で苦しんでいる方と 黄斑変性などの網膜の 疾病により失明のリスクを 抱える人は米国に1000万人 世界中にも数多くいます 現在この症状への 対処法はほとんどありません 薬物療法もありますが 効力を発揮するのは 一握りの患者だけです ですから大抵の場合は補綴装置による 視覚回復が頼みの綱となります 現在出回っている装置では 視覚は限定されてしまい 真の補綴とは言えません 例えば 明るい光や 高コントラストの部分なら 見えるようになりますが これが限界で 正常な視覚には ほど遠いというのが現状です
本日 紹介するのは 私たちが研究を続けてきた より効果的で 失明治療の 分岐点になりうる装置です その仕組みをお見せします 少し詳しくなりますが まず私たちが解決を目指す課題を 理解頂くために健康な網膜の 働きを簡単に説明します 左側が画像で 次に網膜― 右側が脳です 目にこの赤ん坊のような 画像が飛び込んできた際に 網膜最前列の光受容器という 視細胞がこれを受け取ります 次に中心部の 網膜回路が作動します 受信した画像を計算処理し 光情報を抽出して これをコード(一連の信号)に書き換えます この電気パルス信号が 脳に送られます ここで画像が最終的には コード化されるという点が 大切になります 文字通りコード化されます 画面上のコードは”赤ん坊の顔”を表しており 脳がこの信号配列を受け取ると 脳は目に映ったものは 赤ん坊の顔だと認識して 異なる信号配列を受信すれば 犬や家といった 異なる像を認識します 大まかにはこんなところです
実際には画像は 動的で常に変化しています 目に映る外界が常に変るように パルスの信号配列も常に 変化します まぁ 少し複雑なものなんです パルスの信号配列はミリ秒単位で 目から送信され脳に 何を見ているのかを伝えます さて黄斑変性のような 網脈絡膜変性疾患を患った場合には 網膜に何が起こるのでしょう? 最前列の細胞と 光受容器が死滅するだけでなく 繋がっている細胞や網膜回路も 全て死滅してしまいます 最終的に残るのは 脳に情報を送信する 出力細胞のみです しかし変性の後は シグナルを送ることもなくなります インプットがなくなるので 脳に送信されるはずであった 視覚情報が失われます これが失明という状態です
さて対処法はというと 網膜の光受容器と網膜回路を 模倣する装置をつくり 網膜の出力細胞に信号を送り 脳への視覚情報の送信を 復旧するというものです これが私たちの研究課題でした そしてこれがその補綴装置です エンコーダとトランスデューサ(変換器)で 構成されています このエンコーダが網膜前部の 画像の受信と そのコード化の プロセスを代行します 次にトランスデューサが コードを出力細胞へ渡し 脳へコードが送られます こうして この補綴装置は 通常の網膜出力を再現します こうすることで 網膜回路や 光受容器を失った 失明状態であっても 脳が画像と解釈できるように 通常通り信号を送れるのです これは今まで成し得なかった 初の快挙なんです
さてこの装置の 中核であるエンコーダについて もう少しお話しします 興味深く いかした部分でもあります ”いかした”というのは 適切な言葉かどうか分かりませんが 言いたいことは伝わるでしょう ここでは網膜回路と その中核で行われている 複雑な計算を電子チップで 再現しているのです 単純な計算処理なんですよ つまり網膜の代替物を埋め込んでいるのでもなく 異なる役割の全ての細胞に対応する マイクロデバイスを 作っているのでもありません 網膜の働きを一連の計算として 抽出し再現しているのです ある意味この計算式が 電信暗号帳の役割を果たしています 通常の網膜が行うように 画像が受信され 一連の計算を通り抜け 電気信号に書き換えられるのです
それではこの装置を利用して 正常な出力ができることと この進歩が一体何を意味するのか お見せしようと思います これは3種類の発信信号記録です 一番上は盲目でない動物の記録で 真ん中が私たちの 補綴装置で治療を受けた 盲目状態の信号記録です 下が従来の補綴装置を使用した 盲目状態の信号記録です 下の記録はエンコーダなしの 光検出器を利用した 現在利用されている中では 最先端機器を使用した信号記録です それぞれに対して 人間・赤ん坊 公園のベンチなど日常生活で 目にする画像を見せた後の 先ほどの3種類の状態の 網膜の反応を記録しました 3つの図には 種類毎の 発信信号が示されており 先ほどのスライドと同様に 縦軸は 5~6個のそれぞれの細胞に対応しています データ全体を見やすくする 便宜上 パルス信号を 縮小して表示してあります
ご覧の通りエンコーダと トランスデューサを使用した 2番目の盲目グループの発信信号は 完璧ではないものの 正常なものと大差有りません 従来の補綴装置を 使用した3番目のグループは 信号がほとんど一致しません つまり従来の方法は送信を可能にするものの 正しくコード化されていないために 正常な発信信号とは 異なる情報を送信してしまうのです これはどういうことでしょうか? 利用者の視覚能力に どのような影響を及ぼすのでしょう? この質問にお答えするため ある重要な実験をお見せします 他にもデータはたくさんありますので 興味の有る方にはお見せいたします これは再構築実験と 呼ばれています 動画の部分部分を抜き出し そこで網膜は何を見ていたのかを 確認するというものです さて発信信号から 網膜が見ていた画像を 再現できるのでしょうか?
この実験では私たちの装置と 従来の装置の両方で 再現性を確認しました それでは結果ですが 従来の装置ではこうなりました 限定的であることが伺えます 発信信号が正しく コード化されておらず 何かがあるのは分かりますが 像はとても不明瞭であり 情報量は貧弱です 先ほどお話しした通り 高コントラスト部や 明るい部分しか見えないために このような不明瞭な画像が 生成されてしまうのです 実際の画像はなんだったのでしょう? 赤ん坊の顔です さて コードに変換する私たちの アプローチはどうでしょうか? かなりの改善が見られれますね 赤ん坊の顔だと分かるだけでなく 元画像と同じ赤ん坊だと認識できます これは本当に大変な作業なんですよ 左側はエンコーダ単体を使用したもので 右側はエンコーダとトランスデューサを 両方を使用した場合の画像です しかしここでの鍵はエンコーダです というのもこれは色々な トランスデューサと組み合わせられます
実はこれが初めての試作品なんですよ 従来の方法にもコメントしておきましょう 盲目の網膜が画像を伝達できるなんて 発表された当時は 本当に心躍らされました しかし コードもなく信号精度の 改善も必要で 健康な網膜と同じ様に 忠実に再現するという点では 制約を抱えていましたが 私たちがこれらを解決しました まとめると 本日はコードを介して 脳に正しく伝達できることと その手段の一例として 私たちの装置を紹介しました 先ほど申し上げたとおり データは他にいくらでもありますので 興味のある方には お見せいたします この補綴装置は運動系装置のように 脳からの信号を装置に伝えている わけではありません 外界の情報を脳に 伝え これを脳が 理解することが重要なのです
最後に強調してお伝えしたいのは この考え方は汎用的に 適用できるという点です 私たちが網膜のコードを解明した手順を 聴覚や運動系に対応する コード発見に活用することで 聴覚消失症や運動不全障害も 治療することが 十分可能です 網膜回路を飛び越えて その先の出力細胞に たどり着いたのと同じ方法で 蝸牛殻の先の 聴覚神経にたどり着いたり 脳卒中によって生じた 大脳皮質内と 運動皮質との間の溝を 埋めることもできます
最後にコードの理解こそが 大きな意味を持つということは 覚えておいて下さい この脳の言語であるコードを 解明できれば以前は明らかに 不可能であったことが可能となるのです ご静聴ありがとうございました
(拍手)
品詞分類
- 主語
- 動詞
- 助動詞
- 準動詞
- 関係詞等
TED 日本語
TED Talks
関連動画
うつやPTSDを予防できる新種の薬 | TED Talkレベッカ・ブラックマン
2019.04.17騒音が健康に有害な理由 ― そして私たちにできることマティアス・バスナー
2019.02.26さまよう心を鎮めるには | TED Talkアミシ・ジャー
2018.04.18鬱の友達と心を通わせるにはビル・バーナット
2018.03.23脳に良い変化をもたらす運動の効果ウェンディー・スズキ
おすすめ 12018.03.21物事の「良し悪し」は思い込みに過ぎないヘザー・ラニエ
おすすめ 12018.01.19脳が深い睡眠から更なる恩恵を得る方法ダン・ガーテンバーグ
2018.01.04うつを一人で抱え込まないでニッキー・ウェバー・アレン
2017.10.26長寿の秘訣は周囲の人との交流かもスーザン・ピンカー
2017.09.04脳はどのように美しさを判定するか?アンジャン・チャタジー
2017.08.22脳が「意識された現実」という幻覚を作り出す仕組みアニル・セス
2017.07.18注意を向けた時、脳では何が起きているのかメディ・オディカニ=セイドラー
2017.07.12ティーンエイジャーの登校時間を遅らせるべき理由とはウェンディ・トロクセル
2017.06.09死に直面したとき、人生に生きる価値を与えてくれるのはルーシー・カラニシ
おすすめ 12017.06.07最善の自己と最悪の自己の生物学ロバート・サポルスキー
2017.05.31メンタルヘルスを気遣うのは恥ずかしくなんかないサング・デリ
2017.05.26
洋楽 おすすめ
RECOMMENDS
洋楽歌詞
ステイザ・キッド・ラロイ、ジャスティン・ビーバー
洋楽最新ヒット2021.08.20スピーチレス~心の声ナオミ・スコット
洋楽最新ヒット2019.05.23シェイプ・オブ・ユーエド・シーラン
洋楽人気動画2017.01.30フェイデッドアラン・ウォーカー
洋楽人気動画2015.12.03ウェイティング・フォー・ラヴアヴィーチー
洋楽人気動画2015.06.26シー・ユー・アゲインウィズ・カリファ
洋楽人気動画2015.04.06シュガーマルーン5
洋楽人気動画2015.01.14シェイク・イット・オフテイラー・スウィフト
ポップス2014.08.18オール・アバウト・ザット・ベースメーガン・トレイナー
ポップス2014.06.11ストーリー・オブ・マイ・ライフワン・ダイレクション
洋楽人気動画2013.11.03コール・ミー・メイビーカーリー・レイ・ジェプセン
洋楽人気動画2012.03.01美しき生命コールドプレイ
洋楽人気動画2008.08.04バッド・デイ~ついてない日の応援歌ダニエル・パウター
洋楽人気動画2008.05.14サウザンド・マイルズヴァネッサ・カールトン
洋楽人気動画2008.02.19イッツ・マイ・ライフボン・ジョヴィ
ロック2007.10.11アイ・ウォント・イット・ザット・ウェイバックストリート・ボーイズ
洋楽人気動画2007.09.14マイ・ハート・ウィル・ゴー・オンセリーヌ・ディオン
洋楽人気動画2007.07.12ヒーローマライア・キャリー
洋楽人気動画2007.03.21オールウェイズ・ラヴ・ユーホイットニー・ヒューストン
洋楽人気動画2007.02.19オネスティビリー・ジョエル
洋楽人気動画2005.09.16