
TED日本語
TED Talks(英語 日本語字幕付き動画)
TED日本語 - デブ・ロイ: 初めて言えた時
TED Talks
初めて言えた時
The birth of a word
デブ・ロイ
Deb Roy
内容
MIT研究員デブ・ロイは、まだ赤ちゃんの息子がどうやって言語を習得するのか解明すべく家中にカメラを取り付けました。子どもの日常を(例外を除いて)すべて撮影し、9万時間に及ぶホームビデオを整理し、「ガー」という発音が時間をかけてゆっくりと「ウォーター」に変わりゆく軌跡を聴かせてくれます。人はどう学習するのでしょう。驚愕するようなデータにあふれた研究成果を深い含蓄を織り交ぜて語ります。
字幕
SCRIPT
Script
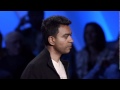
Imagine if you could record your life -- everything you said, everything you did, available in a perfect memory store at your fingertips, so you could go back and find memorable moments and relive them, or sift through traces of time and discover patterns in your own life that previously had gone undiscovered. Well that's exactly the journey that my family began five and a half years ago. This is my wife and collaborator, Rupal. And on this day, at this moment, we walked into the house with our first child, our beautiful baby boy. And we walked into a house with a very special home video recording system.
Deb Roy: This moment and thousands of other moments special for us were captured in our home because in every room in the house, if you looked up, you'd see a camera and a microphone, and if you looked down, you'd get this bird's-eye view of the room. Here's our living room, the baby bedroom, kitchen, dining room and the rest of the house. And all of these fed into a disc array that was designed for a continuous capture. So here we are flying through a day in our home as we move from sunlit morning through incandescent evening and, finally, lights out for the day. Over the course of three years, we recorded eight to 10 hours a day, amassing roughly a quarter-million hours of multi-track audio and video.
So you're looking at a piece of what is by far the largest home video collection ever made. (Laughter) And what this data represents for our family at a personal level, the impact has already been immense, and we're still learning its value. Countless moments of unsolicited natural moments, not posed moments, are captured there, and we're starting to learn how to discover them and find them.
But there's also a scientific reason that drove this project, which was to use this natural longitudinal data to understand the process of how a child learns language -- that child being my son. And so with many privacy provisions put in place to protect everyone who was recorded in the data, we made elements of the data available to my trusted research team at MIT so we could start teasing apart patterns in this massive data set, trying to understand the influence of social environments on language acquisition. So we're looking here at one of the first things we started to do. This is my wife and I cooking breakfast in the kitchen, and as we move through space and through time, a very everyday pattern of life in the kitchen.
In order to convert this opaque,90,000 hours of video into something that we could start to see, we use motion analysis to pull out, as we move through space and through time, what we call space-time worms. And this has become part of our toolkit for being able to look and see where the activities are in the data, and with it, trace the pattern of, in particular, where my son moved throughout the home, so that we could focus our transcription efforts, all of the speech environment around my son -- all of the words that he heard from myself, my wife, our nanny, and over time, the words he began to produce. So with that technology and that data and the ability to, with machine assistance, transcribe speech, we've now transcribed well over seven million words of our home transcripts. And with that, let me take you now for a first tour into the data.
So you've all, I'm sure, seen time-lapse videos where a flower will blossom as you accelerate time. I'd like you to now experience the blossoming of a speech form. My son, soon after his first birthday, would say "gaga" to mean water. And over the course of the next half-year, he slowly learned to approximate the proper adult form, "water." So we're going to cruise through half a year in about 40 seconds. No video here, so you can focus on the sound, the acoustics, of a new kind of trajectory: gaga to water.
(Audio) Baby: Gagagagagaga Gaga gaga gaga guga guga guga wada gaga gaga guga gaga wader guga guga water water water water water water water water water.
DR: He sure nailed it, didn't he.
(Applause)
So he didn't just learn water. Over the course of the 24 months, the first two years that we really focused on, this is a map of every word he learned in chronological order. And because we have full transcripts, we've identified each of the 503 words that he learned to produce by his second birthday. He was an early talker. And so we started to analyze why. Why were certain words born before others? This is one of the first results that came out of our study a little over a year ago that really surprised us. The way to interpret this apparently simple graph is, on the vertical is an indication of how complex caregiver utterances are based on the length of utterances. And the [ horizontal ] axis is time.
And all of the data, we aligned based on the following idea: Every time my son would learn a word, we would trace back and look at all of the language he heard that contained that word. And we would plot the relative length of the utterances. And what we found was this curious phenomena, that caregiver speech would systematically dip to a minimum, making language as simple as possible, and then slowly ascend back up in complexity. And the amazing thing was that bounce, that dip, lined up almost precisely with when each word was born -- word after word, systematically. So it appears that all three primary caregivers -- myself, my wife and our nanny -- were systematically and, I would think, subconsciously restructuring our language to meet him at the birth of a word and bring him gently into more complex language. And the implications of this -- there are many, but one I just want to point out, is that there must be amazing feedback loops. Of course, my son is learning from his linguistic environment, but the environment is learning from him. That environment, people, are in these tight feedback loops and creating a kind of scaffolding that has not been noticed until now.
But that's looking at the speech context. What about the visual context? We're not looking at -- think of this as a dollhouse cutaway of our house. We've taken those circular fish-eye lens cameras, and we've done some optical correction, and then we can bring it into three-dimensional life. So welcome to my home. This is a moment,one moment captured across multiple cameras. The reason we did this is to create the ultimate memory machine, where you can go back and interactively fly around and then breathe video-life into this system. What I'm going to do is give you an accelerated view of 30 minutes, again, of just life in the living room. That's me and my son on the floor. And there's video analytics that are tracking our movements. My son is leaving red ink. I am leaving green ink. We're now on the couch, looking out through the window at cars passing by. And finally, my son playing in a walking toy by himself.
Now we freeze the action,30 minutes, we turn time into the vertical axis, and we open up for a view of these interaction traces we've just left behind. And we see these amazing structures -- these little knots of two colors of thread we call "social hot spots." The spiral thread we call a "solo hot spot." And we think that these affect the way language is learned. What we'd like to do is start understanding the interaction between these patterns and the language that my son is exposed to to see if we can predict how the structure of when words are heard affects when they're learned -- so in other words, the relationship between words and what they're about in the world.
So here's how we're approaching this. In this video, again, my son is being traced out. He's leaving red ink behind. And there's our nanny by the door.
(Video) Nanny: You want water? (Baby: Aaaa.) Nanny: All right. (Baby: Aaaa.)
DR: She offers water, and off go the two worms over to the kitchen to get water. And what we've done is use the word "water" to tag that moment, that bit of activity. And now we take the power of data and take every time my son ever heard the word water and the context he saw it in, and we use it to penetrate through the video and find every activity trace that co-occurred with an instance of water. And what this data leaves in its wake is a landscape. We call these wordscapes. This is the wordscape for the word water, and you can see most of the action is in the kitchen. That's where those big peaks are over to the left. And just for contrast, we can do this with any word. We can take the word "bye" as in "good bye." And we're now zoomed in over the entrance to the house. And we look, and we find, as you would expect, a contrast in the landscape where the word "bye" occurs much more in a structured way. So we're using these structures to start predicting the order of language acquisition, and that's ongoing work now.
In my lab, which we're peering into now, at MIT -- this is at the media lab. This has become my favorite way of videographing just about any space. Three of the key people in this project, Philip DeCamp, Rony Kubat and Brandon Roy are pictured here. Philip has been a close collaborator on all the visualizations you're seeing. And Michael Fleischman was another Ph.D. student in my lab who worked with me on this home video analysis, and he made the following observation: that "just the way that we're analyzing how language connects to events which provide common ground for language, that same idea we can take out of your home, Deb, and we can apply it to the world of public media." And so our effort took an unexpected turn.
Think of mass media as providing common ground and you have the recipe for taking this idea to a whole new place. We've started analyzing television content using the same principles -- analyzing event structure of a TV signal -- episodes of shows, commercials, all of the components that make up the event structure. And we're now, with satellite dishes, pulling and analyzing a good part of all the TV being watched in the United States. And you don't have to now go and instrument living rooms with microphones to get people's conversations, you just tune into publicly available social media feeds.
So we're pulling in about three billion comments a month, and then the magic happens. You have the event structure, the common ground that the words are about, coming out of the television feeds; you've got the conversations that are about those topics; and through semantic analysis -- and this is actually real data you're looking at from our data processing -- each yellow line is showing a link being made between a comment in the wild and a piece of event structure coming out of the television signal. And the same idea now can be built up. And we get this wordscape, except now words are not assembled in my living room. Instead, the context, the common ground activities, are the content on television that's driving the conversations. And what we're seeing here, these skyscrapers now, are commentary that are linked to content on television. Same concept, but looking at communication dynamics in a very different sphere.
And so fundamentally, rather than, for example, measuring content based on how many people are watching, this gives us the basic data for looking at engagement properties of content. And just like we can look at feedback cycles and dynamics in a family, we can now open up the same concepts and look at much larger groups of people. This is a subset of data from our database -- just 50,000 out of several million -- and the social graph that connects them through publicly available sources. And if you put them on one plain, a second plain is where the content lives. So we have the programs and the sporting events and the commercials, and all of the link structures that tie them together make a content graph. And then the important third dimension. Each of the links that you're seeing rendered here is an actual connection made between something someone said and a piece of content. And there are, again, now tens of millions of these links that give us the connective tissue of social graphs and how they relate to content. And we can now start to probe the structure in interesting ways.
So if we, for example, trace the path of one piece of content that drives someone to comment on it, and then we follow where that comment goes, and then look at the entire social graph that becomes activated and then trace back to see the relationship between that social graph and content, a very interesting structure becomes visible. We call this a co-viewing clique, a virtual living room if you will. And there are fascinating dynamics at play. It's not one way. A piece of content, an event, causes someone to talk. They talk to other people. That drives tune-in behavior back into mass media, and you have these cycles that drive the overall behavior.
Another example -- very different -- another actual person in our database -- and we're finding at least hundreds, if not thousands, of these. We've given this person a name. This is a pro-amateur, or pro-am media critic who has this high fan-out rate. So a lot of people are following this person -- very influential -- and they have a propensity to talk about what's on TV. So this person is a key link in connecting mass media and social media together.
One last example from this data: Sometimes it's actually a piece of content that is special. So if we go and look at this piece of content, President Obama's State of the Union address from just a few weeks ago, and look at what we find in this same data set, at the same scale, the engagement properties of this piece of content are truly remarkable. A nation exploding in conversation in real time in response to what's on the broadcast. And of course, through all of these lines are flowing unstructured language. We can X-ray and get a real-time pulse of a nation, real-time sense of the social reactions in the different circuits in the social graph being activated by content.
So, to summarize, the idea is this: As our world becomes increasingly instrumented and we have the capabilities to collect and connect the dots between what people are saying and the context they're saying it in, what's emerging is an ability to see new social structures and dynamics that have previously not been seen. It's like building a microscope or telescope and revealing new structures about our own behavior around communication. And I think the implications here are profound, whether it's for science, for commerce, for government, or perhaps most of all, for us as individuals.
And so just to return to my son, when I was preparing this talk, he was looking over my shoulder, and I showed him the clips I was going to show to you today, and I asked him for permission -- granted. And then I went on to reflect, "Isn't it amazing, this entire database, all these recordings, I'm going to hand off to you and to your sister" -- who arrived two years later -- "and you guys are going to be able to go back and re-experience moments that you could never, with your biological memory, possibly remember the way you can now?" And he was quiet for a moment. And I thought, "What am I thinking? He's five years old. He's not going to understand this." And just as I was having that thought, he looked up at me and said, "So that when I grow up, I can show this to my kids?" And I thought, "Wow, this is powerful stuff."
So I want to leave you with one last memorable moment from our family. This is the first time our son took more than two steps at once -- captured on film. And I really want you to focus on something as I take you through. It's a cluttered environment; it's natural life. My mother's in the kitchen, cooking, and, of all places, in the hallway, I realize he's about to do it, about to take more than two steps. And so you hear me encouraging him, realizing what's happening, and then the magic happens. Listen very carefully. About three steps in, he realizes something magic is happening, and the most amazing feedback loop of all kicks in, and he takes a breath in, and he whispers "wow" and instinctively I echo back the same. And so let's fly back in time to that memorable moment.
(Video) DR: Hey. Come here. Can you do it? Oh, boy. Can you do it? Baby: Yeah. DR: Ma, he's walking.
(Laughter)
(Applause)
DR: Thank you.
(Applause)
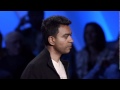
人生を記録できるとしたらどうでしょう どんな発言も どんな振る舞いも 手近な記憶装置に残しておけたら 過去に戻って 心に残る思い出を 再生したり 時間の流れを精査することで 見過ごしていた 生活パターンを見つけたりできます それこそ まさに 私たち一家が 5年半前に始めた旅なのです 妻と 協力者ルーパルです この日 この時 第一子を迎え入れました かわいい男の子です 家には かなり特殊な ホームビデオの撮影機材を取り付けました
「じゃあ撮るよ」
ほかにも 我が家の貴重な瞬間を たっぷり撮影しました どの部屋にも 見上げればカメラとマイクがあって そこから下を眺めれば 部屋を一望できます リビング 赤ちゃんの寝室 キッチン ダイニング 残りの部屋も ディスク記録装置に全部送って 継続的に記録しました 一日の流れを見渡せます 日の差す明け方から 燃えるような夕刻を迎え 消灯して 一日を終えます 3年間 毎日 8から10時間 合計 約25万時間に及ぶ マルチトラックの音声と映像の記録です
史上初の 壮大な ホームビデオ全集なのです (笑い) 私たち家族は 意義ある記録だと感じています 大きな衝撃を受けながら 今も 真価を探っています 意識せず 自然体で 身構えもしない膨大な時間が 記録されていますので どう調べるか検討を始めたところです
この取り組みには 科学的理由もあります 時系列に連なる この生データから 子どもの言語習得の過程を 把握したいのです 対象は 私の息子です プライバシー保護規定を設けて 被写体のプライバシーを守ったうえで データを 部分的に参照可能にして MITの信頼できる研究チームに開放しました こうして 一連の膨大なデータから パターンを抽出できるようになり 言語習得過程における社会環境の影響を探る― 挑戦が始まりました ご覧いただいているのは 初めて手をつけた解析です キッチンで妻と私が朝食を作っています 空間的・時間的に眺めると キッチンでの毎日の生活パターンが現れます
この とらえ所のない 9万時間の映像を 理解可能にするため 動作分析の手法を使って 空間軸と時間軸でとらえ 「時空の虫」を描き出しました このツールを使うと 活動が生じたデータ位置を 把握できます 特に 子どもの動き回るパターンを 追跡できるので 書き起こしに専念できました 息子を取り巻く会話環境 私 妻 おばあさんが口にした言葉 やがて息子の口から出る言葉 すべてです この技術があって データがあって 装置も使って 会話を書き起こせたので 家族の発した言葉を 700万以上書き起こせました その成果を使って 初めて データの旅へご案内いたします
低速度撮影の映像は 経験があると思います 早送りで開花を見せる映像です 今回は 言葉の開花に 立ち会っていただきます 息子は1歳になってすぐ ウォーター (water) をガガ (gaga)と言い それから半年かけて ゆっくりと 大人のように正確な ウォーター (water)に近づきました では 半年間を 40秒で体験します 映像はありません 初となる 音の軌跡に 耳を澄ませてください ガガ (gaga) から ウォーター (water) へ
(赤ちゃん)「Gagagagagaga」 「Gaga gaga gaga」 「guga guga guga」 「wada gaga gaga guga gaga」 「wader guga guga」 「water water water」 「water water water」 「water water」 「water.」
大成功です
(拍手)
ウォーターだけではありません 24ヶ月 つまり 最初の2年間に絞って 習得した言葉を 年代順に並べました 一字一句 書き起こして 2歳になるまでに 口にした 全503語を確認しました 息子は 早い方です こんな分析も始めました なぜ ある語が ほかの語より先なのか? こちらは 1年少し前の 初期の研究成果の一つです 驚きの結果です 一見シンプルですが 説明しますと 縦軸は 周囲の人が話す 会話の複雑さです 基準は会話の長さです 横軸は時間です
データはすべて こんな手法で導き出しました 習得した言葉ごとに その言葉を使った過去の会話を すべて洗い出して 会話文の相対的な長さをプロットしたのです すると 面白いことに きまって 大人は語数を減らし できるだけ短くしてから ゆっくりと複雑な言葉遣いに戻します 驚いたことに 折り返し地点は その言葉を口にした時期と ほぼ一致するのです どの言葉も 同じです つまり 3人の大人たち― 私 妻 おばあさん のだれもが いつも 何げなく 言葉遣いを調整してあげて 息子がその言葉を口にしたら もっと複雑な言葉へ そっと導いていたのです 示唆に富む結果ですが 伝えたいのは一点 見事なフィードバック・ループが形成されていること 息子が 周りの言語環境から 学習している一方で 言語環境も 息子から学習しているのです 言語環境 つまり人が 堅固なループに加わり 足場を固めているのです 今まで 気付いた人はいません
以上は 話の環境です 視覚的な環境はどうか? 模型のように 我が家をのぞいてみます 魚眼レンズで撮影した映像を 光学的に修正して 三次元の世界に仕上げました ようこそ 我が家へ これは ある瞬間を 複数台で横断的にとらえた映像です 過去に戻って 自由に飛び回れる 究極の記憶装置を 実現させたかったのです 今から 30分を早送りで見ていただきます リビングの様子です 私と息子が 床にいます 映像解析で 二人の動きを追います 息子は赤 私は緑 ソファーに移りました 窓から 車が走るのを眺めています 最後は一人で歩行器に乗りました
30分の動きを一つにまとめて 上下に時間軸をとれば ふれあいの軌跡を 見ることができます 驚くような形状が現れました 二色が絡み合っているのは ふれあいの強い場所です らせんになっているのは 単独行動の場所です これは言語学習過程に影響を及ぼすと 考えられます このパターンと 息子の耳に入る言葉との 関係を探ることによって 言葉を耳にする時期が 習得時期に及ぼす影響を 予測できるか探りたいのです つまり 言葉と 言葉が表す現実との関係です
やり方を紹介しましょう この映像でも 息子を追います 赤い線です おばあさんはドアの前です
「ウォーター(water)いる?」「アァー(Aaaa)」 「いいわよ」「アー(Aaaa)」
水が欲しいか尋ねてから 二つの「虫」が キッチンまでつながりました そこで「ウォーター」の語を この瞬間と結びつけました データの力を活用して ウォーターを耳にした 時と場所に 注目して 映像全体から ウォーターに絡む行動の軌跡を すべて抽出しました データ処理後に残る― ランドスケープ(地形)が ワードスケープ(言葉の地形)です ウォーターの場合 キッチンを中心に動いています 左奥の高いピークの所です ほかの言葉と対比することもできます グッバーイ(good bye)の バーイ(bye)はどうでしょう 今回は玄関を見てみます 予想どおりのランドスケープが現れます バーイ(bye)の軌跡は 一層わかりやすい形をしています これらの形状を参考に 言葉の習得順序を 予測することにしました これは現在進行中です
これは今の 私の研究室です MITメディアラボ内です どこでも こうやって 撮影してしまいます プロジェクトの主要メンバーは フィリップ・ド・キャンプ ロニー・クバート ブランドン・ロイです フィリップが協力してくれたのは 先ほどの視覚化です マイケル・フライシュマンは 博士課程の学生で 映像解析を手伝っています マイケルはこんな予測を立てました 「共通基盤であるイベントに 言葉がどう関係するか分析するという この手法は 家庭内だけでなく 公共のメディアにも応用できる」 研究は 思わぬ方向に転がりました
共通基盤を提供するのは マスメディアです これまでのアイデアを 新境地に応用するやり方は分かっています テレビの番組コンテンツを 同じ原理で 解析しました テレビ信号で送られる要素 つまり ドラマのストーリーや コマーシャルなど イベント構造を構築する全要素を解析します アメリカで視聴可能な大半の番組は アンテナで拾えます 会話は リビングのマイクで録音する必要など ありません だれでも見られるソーシャルメディアから拾うだけです
月に30億のコメントを 入手しています すると 不思議な結果が出ました イベント構造 つまり 言葉が関与する共通基盤を テレビ放送から取り出して そのトピックに関連のある 会話を抽出しました さらに 意味解析を通じて― こちらは実際に処理した データです 黄色の線がつなぐのは 生のコメントと テレビ信号で送られるイベント構造です 先ほどのアイデアを 発展させて こんな― ワードスケープを作りました 言葉の出所はリビングではありません 番組コンテンツが 環境つまり共通基盤となって 会話を引き出しているのです この高層ビル群を作るのは 番組コンテンツに関する コメントです コンセプトは同じですが 先ほどとは全く別領域の コミュニケーション・ダイナミクスが見えます
基本的には 例えば 番組コンテンツの評価に視聴者数を使いません 番組コンテンツへの関心度 に焦点をあてた基本データを使います 家庭で起こるフィードバック・サイクルや ダイナミクスと同じようなものです 今や 同じコンセプトを発展させて もっと大きな集団を対象にできるのです これは データベースの一部― 数百万のうち5万人分のデータを 公の情報源に基づいて 結びつけるソーシャル・グラフです 第一の平面にそれを置き 第二の平面に番組コンテンツをのせます 番組 例えば スポーツ大会 コマーシャル すべてを結ぶリンク構造が コンテンツ・グラフです 重要なのは第三の次元です 浮かび上がる各リンクは だれかの発言と 番組コンテンツとを 結んでいます 数千万のリンクが ソーシャル・グラフの結合組織となって 番組コンテンツに結びついています 面白いやり方で この構造を探検できます
コメントを引き出す番組コンテンツからのびる― 経路を たどりたいときは コメントの流れを追って ソーシャル・グラフ全体の活動を見渡して ソーシャル・グラフと番組コンテンツとの関係性を 再確認します すると 興味深い構造― 共視聴グループが現れます 疑似リビングといえるかもしれません そこに興味深いダイナミクスが現れます 一方向ではなく 番組コンテンツつまりイベントから会話が生まれ 別の人に広がって また マスメディアにチャンネルを合わせます 全体の行動を誘発する― サイクルがあるのです
ほかにも例があります データベースには数千とまではいきませんが ある種の人が 数百人います その名も プロ・アマチュア(プロアマ)・メディア批評家です 幅広く影響力が及ぶので 批評を聞くと たくさんの人が 番組の話をします マスメディアとソーシャルメディアとの 架け橋になる人たちです
次は 最後の例となります 番組コンテンツには特殊なものもあります 例えば ほんの数週間前の オバマ大統領の 一般教書演説です データ群から何が分かるか 同じ視点で見ると コンテンツへの関心度は 飛び抜けているのが分かります 放送に反応して リアルタイムに 会話が広がっています このすべての線を通じて 雑多な話が飛び交っています 世界の脈動を リアルタイムに見通せて ソーシャル・グラフ内で 番組コンテンツに誘発された様々な集団の 社会的反応をリアルタイムに見通せます
まとめると この世界に手段が増えていき 人々の発言と その発言の背景との 結びつきを 把握できるようになって 見えてきたのは 今までにない新たな社会構造や ダイナミクスです 顕微鏡や望遠鏡を作り コミュニケーションに絡む行動に 新たな構造を見い出すようなものです ここには 深い意味があると思っています 科学的にも 商業的にも 政治的にも そして特に 個人にとっても深い意味があります
息子の話に戻りますが 講演の準備中に 息子が肩越しにのぞくので 一連の動画を見せて 息子の許可を伺いました それからよく考えて 「すごくない? これだけのデータベースと記録を 君や妹に残すんだよ」 2歳下の妹のことです 「過去に戻って 追体験できるんだ 生物学的には絶対記憶できないことを 覚えておけるんだよ」 息子は口を開きません 「まだ5歳だった 分かるわけないな」 そう思っていると 息子は口を開きました 「ぼくが大きくなったら 子どもに見せられるね」 これはすごいことだと思いました
では 最後に 私たち家族にとって 思い出に残る場面をご覧いただきます 息子が初めて 2歩以上歩いた瞬間を とらえた映像です ぜひ注目してください これは 雑然とした― 日常生活の様子です 母がキッチンで料理中 私は廊下にいて 歩きそうだと気づきました 息子に声援を送り これから起こる事を予感し 感動の瞬間を迎えます 注意して聞いてください 三つの段階があります 素晴らしい瞬間を目にして 最高のフィードバック・ループが始まり 一呼吸置いて 「やった」とつぶやきます 無意識に何度も繰り返します では 思い出のあの瞬間へ 飛び立ちましょう
「よ~し」 「おいで」 「できるかな?」 「いい子だ」 「できるかな?」 (赤ちゃん)「ヤァ~」 「お~い 歩いてるよ~」
(笑い)
(拍手)
ありがとうございました
(拍手)
品詞分類
- 主語
- 動詞
- 助動詞
- 準動詞
- 関係詞等
TED 日本語
TED Talks
関連動画
うつやPTSDを予防できる新種の薬 | TED Talkレベッカ・ブラックマン
2019.04.17騒音が健康に有害な理由 ― そして私たちにできることマティアス・バスナー
2019.02.26さまよう心を鎮めるには | TED Talkアミシ・ジャー
2018.04.18鬱の友達と心を通わせるにはビル・バーナット
2018.03.23脳に良い変化をもたらす運動の効果ウェンディー・スズキ
おすすめ 12018.03.21物事の「良し悪し」は思い込みに過ぎないヘザー・ラニエ
おすすめ 12018.01.19脳が深い睡眠から更なる恩恵を得る方法ダン・ガーテンバーグ
2018.01.04うつを一人で抱え込まないでニッキー・ウェバー・アレン
2017.10.26長寿の秘訣は周囲の人との交流かもスーザン・ピンカー
2017.09.04脳はどのように美しさを判定するか?アンジャン・チャタジー
2017.08.22脳が「意識された現実」という幻覚を作り出す仕組みアニル・セス
2017.07.18注意を向けた時、脳では何が起きているのかメディ・オディカニ=セイドラー
2017.07.12ティーンエイジャーの登校時間を遅らせるべき理由とはウェンディ・トロクセル
2017.06.09死に直面したとき、人生に生きる価値を与えてくれるのはルーシー・カラニシ
おすすめ 12017.06.07最善の自己と最悪の自己の生物学ロバート・サポルスキー
2017.05.31メンタルヘルスを気遣うのは恥ずかしくなんかないサング・デリ
2017.05.26
洋楽 おすすめ
RECOMMENDS
洋楽歌詞
ステイザ・キッド・ラロイ、ジャスティン・ビーバー
洋楽最新ヒット2021.08.20スピーチレス~心の声ナオミ・スコット
洋楽最新ヒット2019.05.23シェイプ・オブ・ユーエド・シーラン
洋楽人気動画2017.01.30フェイデッドアラン・ウォーカー
洋楽人気動画2015.12.03ウェイティング・フォー・ラヴアヴィーチー
洋楽人気動画2015.06.26シー・ユー・アゲインウィズ・カリファ
洋楽人気動画2015.04.06シュガーマルーン5
洋楽人気動画2015.01.14シェイク・イット・オフテイラー・スウィフト
ポップス2014.08.18オール・アバウト・ザット・ベースメーガン・トレイナー
ポップス2014.06.11ストーリー・オブ・マイ・ライフワン・ダイレクション
洋楽人気動画2013.11.03コール・ミー・メイビーカーリー・レイ・ジェプセン
洋楽人気動画2012.03.01美しき生命コールドプレイ
洋楽人気動画2008.08.04バッド・デイ~ついてない日の応援歌ダニエル・パウター
洋楽人気動画2008.05.14サウザンド・マイルズヴァネッサ・カールトン
洋楽人気動画2008.02.19イッツ・マイ・ライフボン・ジョヴィ
ロック2007.10.11アイ・ウォント・イット・ザット・ウェイバックストリート・ボーイズ
洋楽人気動画2007.09.14マイ・ハート・ウィル・ゴー・オンセリーヌ・ディオン
洋楽人気動画2007.07.12ヒーローマライア・キャリー
洋楽人気動画2007.03.21オールウェイズ・ラヴ・ユーホイットニー・ヒューストン
洋楽人気動画2007.02.19オネスティビリー・ジョエル
洋楽人気動画2005.09.16