
TED日本語
TED Talks(英語 日本語字幕付き動画)
TED日本語 - ブレイス・アグエラ・ヤルカス: コンピューターはこうしてクリエイティブになる
TED Talks
コンピューターはこうしてクリエイティブになる
How computers are learning to be creative
ブレイス・アグエラ・ヤルカス
Blaise Aguera y Arcas
内容
我々はアートと創造性の新時代の入り口におり、その主人公は人間ではない ― そう語るGoogle社の主席サイエンティストのブレイス・アグエラ・ヤルカスは、深層学習を行うニューラルネットワークで機械知覚と分散学習を実現する取り組みを進めています。画像認識用にトレーニングしたニューラルネットワークを逆に使って非常に斬新な映像を生み出すという、目を奪われるようなデモを彼は披露してくれます。コンピューターが作り出すその映像は、幻想的ですばらしく、既成のジャンル分けには当てはまらないコラージュ作品です。そればかりか、コンピューターは今や詩まで作れるといいます。彼はこう言います。「知覚と創造性の間には非常に密接な結び付きがあり、知覚行為を行う能力を有するものは創造力も兼ね備えている」
字幕
SCRIPT
Script
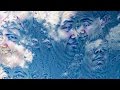
So, I lead a team at Google that works on machine intelligence; in other words, the engineering discipline of making computers and devices able to do some of the things that brains do. And this makes us interested in real brains and neuroscience as well, and especially interested in the things that our brains do that are still far superior to the performance of computers.
Historically,one of those areas has been perception, the process by which things out there in the world -- sounds and images -- can turn into concepts in the mind. This is essential for our own brains, and it's also pretty useful on a computer. The machine perception algorithms, for example, that our team makes, are what enable your pictures on Google Photos to become searchable, based on what's in them. The flip side of perception is creativity: turning a concept into something out there into the world. So over the past year, our work on machine perception has also unexpectedly connected with the world of machine creativity and machine art.
I think Michelangelo had a penetrating insight into to this dual relationship between perception and creativity. This is a famous quote of his: "Every block of stone has a statue inside of it, and the job of the sculptor is to discover it." So I think that what Michelangelo was getting at is that we create by perceiving, and that perception itself is an act of imagination and is the stuff of creativity.
The organ that does all the thinking and perceiving and imagining, of course, is the brain. And I'd like to begin with a brief bit of history about what we know about brains. Because unlike, say, the heart or the intestines, you really can't say very much about a brain by just looking at it, at least with the naked eye. The early anatomists who looked at brains gave the superficial structures of this thing all kinds of fanciful names, like hippocampus, meaning "little shrimp." But of course that sort of thing doesn't tell us very much about what's actually going on inside.
The first person who, I think, really developed some kind of insight into what was going on in the brain was the great Spanish neuroanatomist, Santiago Ramon y Cajal, in the 19th century, who used microscopy and special stains that could selectively fill in or render in very high contrast the individual cells in the brain, in order to start to understand their morphologies. And these are the kinds of drawings that he made of neurons in the 19th century.
This is from a bird brain. And you see this incredible variety of different sorts of cells, even the cellular theory itself was quite new at this point. And these structures, these cells that have these arborizations, these branches that can go very, very long distances -- this was very novel at the time. They're reminiscent, of course, of wires. That might have been obvious to some people in the 19th century; the revolutions of wiring and electricity were just getting underway. But in many ways, these microanatomical drawings of Ramon y Cajal's, like this one, they're still in some ways unsurpassed.
We're still more than a century later, trying to finish the job that Ramon y Cajal started. These are raw data from our collaborators at the Max Planck Institute of Neuroscience. And what our collaborators have done is to image little pieces of brain tissue. The entire sample here is about one cubic millimeter in size, and I'm showing you a very, very small piece of it here. That bar on the left is about one micron. The structures you see are mitochondria that are the size of bacteria. And these are consecutive slices through this very, very tiny block of tissue. Just for comparison's sake, the diameter of an average strand of hair is about 100 microns. So we're looking at something much, much smaller than a single strand of hair.
And from these kinds of serial electron microscopy slices, one can start to make reconstructions in 3D of neurons that look like these. So these are sort of in the same style as Ramon y Cajal. Only a few neurons lit up, because otherwise we wouldn't be able to see anything here. It would be so crowded, so full of structure, of wiring all connecting one neuron to another.
So Ramon y Cajal was a little bit ahead of his time, and progress on understanding the brain proceeded slowly over the next few decades. But we knew that neurons used electricity, and by World War II, our technology was advanced enough to start doing real electrical experiments on live neurons to better understand how they worked. This was the very same time when computers were being invented, very much based on the idea of modeling the brain -- of "intelligent machinery," as Alan Turing called it,one of the fathers of computer science.
Warren McCulloch and Walter Pitts looked at Ramon y Cajal's drawing of visual cortex, which I'm showing here. This is the cortex that processes imagery that comes from the eye. And for them, this looked like a circuit diagram. So there are a lot of details in McCulloch and Pitts's circuit diagram that are not quite right. But this basic idea that visual cortex works like a series of computational elements that pass information one to the next in a cascade, is essentially correct.
Let's talk for a moment about what a model for processing visual information would need to do. The basic task of perception is to take an image like this one and say, "That's a bird," which is a very simple thing for us to do with our brains. But you should all understand that for a computer, this was pretty much impossible just a few years ago. The classical computing paradigm is not one in which this task is easy to do.
So what's going on between the pixels, between the image of the bird and the word "bird," is essentially a set of neurons connected to each other in a neural network, as I'm diagramming here. This neural network could be biological, inside our visual cortices, or, nowadays, we start to have the capability to model such neural networks on the computer. And I'll show you what that actually looks like.
So the pixels you can think about as a first layer of neurons, and that's, in fact, how it works in the eye -- that's the neurons in the retina. And those feed forward into one layer after another layer, after another layer of neurons, all connected by synapses of different weights. The behavior of this network is characterized by the strengths of all of those synapses. Those characterize the computational properties of this network. And at the end of the day, you have a neuron or a small group of neurons that light up, saying, "bird."
Now I'm going to represent those three things -- the input pixels and the synapses in the neural network, and bird, the output -- by three variables: x, w and y. There are maybe a million or so x's -- a million pixels in that image. There are billions or trillions of w's, which represent the weights of all these synapses in the neural network. And there's a very small number of y's, of outputs that that network has. "Bird" is only four letters, right? So let's pretend that this is just a simple formula, x "x" w = y. I'm putting the times in scare quotes because what's really going on there, of course, is a very complicated series of mathematical operations.
That's one equation. There are three variables. And we all know that if you have one equation, you can solve one variable by knowing the other two things. So the problem of inference, that is, figuring out that the picture of a bird is a bird, is this one: it's where y is the unknown and w and x are known. You know the neural network, you know the pixels. As you can see, that's actually a relatively straightforward problem. You multiply two times three and you're done. I'll show you an artificial neural network that we've built recently, doing exactly that.
This is running in real time on a mobile phone, and that's, of course, amazing in its own right, that mobile phones can do so many billions and trillions of operations per second. What you're looking at is a phone looking at one after another picture of a bird, and actually not only saying, "Yes, it's a bird," but identifying the species of bird with a network of this sort. So in that picture, the x and the w are known, and the y is the unknown. I'm glossing over the very difficult part, of course, which is how on earth do we figure out the w, the brain that can do such a thing? How would we ever learn such a model?
So this process of learning, of solving for w, if we were doing this with the simple equation in which we think about these as numbers, we know exactly how to do that: 6 = 2 x w, well, we divide by two and we're done. The problem is with this operator. So, division -- we've used division because it's the inverse to multiplication, but as I've just said, the multiplication is a bit of a lie here. This is a very, very complicated, very non-linear operation; it has no inverse. So we have to figure out a way to solve the equation without a division operator. And the way to do that is fairly straightforward. You just say, let's play a little algebra trick, and move the six over to the right-hand side of the equation. Now, we're still using multiplication. And that zero -- let's think about it as an error. In other words, if we've solved for w the right way, then the error will be zero. And if we haven't gotten it quite right, the error will be greater than zero.
So now we can just take guesses to minimize the error, and that's the sort of thing computers are very good at. So you've taken an initial guess: what if w = 0? Well, then the error is 6. What if w = 1? The error is 4. And then the computer can sort of play Marco Polo, and drive down the error close to zero. As it does that, it's getting successive approximations to w. Typically, it never quite gets there, but after about a dozen steps, we're up to w = 2.999, which is close enough. And this is the learning process.
So remember that what's been going on here is that we've been taking a lot of known x's and known y's and solving for the w in the middle through an iterative process. It's exactly the same way that we do our own learning. We have many, many images as babies and we get told, "This is a bird; this is not a bird." And over time, through iteration, we solve for w, we solve for those neural connections.
So now, we've held x and w fixed to solve for y; that's everyday, fast perception. We figure out how we can solve for w, that's learning, which is a lot harder, because we need to do error minimization, using a lot of training examples.
And about a year ago, Alex Mordvintsev, on our team, decided to experiment with what happens if we try solving for x, given a known w and a known y. In other words, you know that it's a bird, and you already have your neural network that you've trained on birds, but what is the picture of a bird? It turns out that by using exactly the same error-minimization procedure, one can do that with the network trained to recognize birds, and the result turns out to be ... a picture of birds. So this is a picture of birds generated entirely by a neural network that was trained to recognize birds, just by solving for x rather than solving for y, and doing that iteratively.
Here's another fun example. This was a work made by Mike Tyka in our group, which he calls "Animal Parade." It reminds me a little bit of William Kentridge's artworks, in which he makes sketches, rubs them out, makes sketches, rubs them out, and creates a movie this way. In this case, what Mike is doing is varying y over the space of different animals, in a network designed to recognize and distinguish different animals from each other. And you get this strange, Escher-like morph from one animal to another.
Here he and Alex together have tried reducing the y's to a space of only two dimensions, thereby making a map out of the space of all things recognized by this network. Doing this kind of synthesis or generation of imagery over that entire surface, varying y over the surface, you make a kind of map -- a visual map of all the things the network knows how to recognize. The animals are all here; "armadillo" is right in that spot.
You can do this with other kinds of networks as well. This is a network designed to recognize faces, to distinguish one face from another. And here, we're putting in a y that says, "me," my own face parameters. And when this thing solves for x, it generates this rather crazy, kind of cubist, surreal, psychedelic picture of me from multiple points of view at once. The reason it looks like multiple points of view at once is because that network is designed to get rid of the ambiguity of a face being in one pose or another pose, being looked at with one kind of lighting, another kind of lighting. So when you do this sort of reconstruction, if you don't use some sort of guide image or guide statistics, then you'll get a sort of confusion of different points of view, because it's ambiguous. This is what happens if Alex uses his own face as a guide image during that optimization process to reconstruct my own face. So you can see it's not perfect. There's still quite a lot of work to do on how we optimize that optimization process. But you start to get something more like a coherent face, rendered using my own face as a guide.
You don't have to start with a blank canvas or with white noise. When you're solving for x, you can begin with an x, that is itself already some other image. That's what this little demonstration is. This is a network that is designed to categorize all sorts of different objects -- man-made structures, animals ... Here we're starting with just a picture of clouds, and as we optimize, basically, this network is figuring out what it sees in the clouds. And the more time you spend looking at this, the more things you also will see in the clouds. You could also use the face network to hallucinate into this, and you get some pretty crazy stuff.
(Laughter)
Or, Mike has done some other experiments in which he takes that cloud image, hallucinates, zooms, hallucinates, zooms hallucinates, zooms. And in this way, you can get a sort of fugue state of the network, I suppose, or a sort of free association, in which the network is eating its own tail. So every image is now the basis for, "What do I think I see next? What do I think I see next? What do I think I see next?"
I showed this for the first time in public to a group at a lecture in Seattle called "Higher Education" -- this was right after marijuana was legalized.
(Laughter)
So I'd like to finish up quickly by just noting that this technology is not constrained. I've shown you purely visual examples because they're really fun to look at. It's not a purely visual technology. Our artist collaborator, Ross Goodwin, has done experiments involving a camera that takes a picture, and then a computer in his backpack writes a poem using neural networks, based on the contents of the image. And that poetry neural network has been trained on a large corpus of 20th-century poetry. And the poetry is, you know, I think, kind of not bad, actually.
(Laughter)
In closing, I think that per Michelangelo, I think he was right; perception and creativity are very intimately connected. What we've just seen are neural networks that are entirely trained to discriminate, or to recognize different things in the world, able to be run in reverse, to generate. One of the things that suggests to me is not only that Michelangelo really did see the sculpture in the blocks of stone, but that any creature, any being, any alien that is able to do perceptual acts of that sort is also able to create because it's exactly the same machinery that's used in both cases.
Also, I think that perception and creativity are by no means uniquely human. We start to have computer models that can do exactly these sorts of things. And that ought to be unsurprising; the brain is computational.
And finally, computing began as an exercise in designing intelligent machinery. It was very much modeled after the idea of how could we make machines intelligent. And we finally are starting to fulfill now some of the promises of those early pioneers, of Turing and von Neumann and McCulloch and Pitts. And I think that computing is not just about accounting or playing Candy Crush or something. From the beginning, we modeled them after our minds. And they give us both the ability to understand our own minds better and to extend them.
Thank you very much.
(Applause)
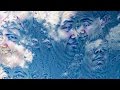
私はGoogleで 機械知能に取り組む 開発チームを率いています 機械知能とは コンピューターや いろいろな種類の端末に 人間の脳のような機能を 持たせるための技術です 仕事上 私たちは 人間の脳の働きや 神経科学に関心があり 脳が未だコンピューターより はるかに優れている領域に 特に興味を持っています
そのような領域として 古くから認識されていたのは 知覚です 知覚とは 外界に存在するもの ― つまり 音や映像のようなものを 心の中の概念に 変えるプロセスです これは 人間の脳に 本質的に備わっている能力ですが コンピューターにも 有用なものです 例えば 私の部署で作っている 機械知覚アルゴリズムは Googleフォトの画像を 写っているものに基づいて 検索できるようにする技術です 一方 知覚と対照的なものに 創造性があります 創造性とは 概念を 何かの形で世に生み出すことです この1年の我々の 機械知覚への取り組みの中で コンピューターによる創造 「機械芸術」の世界との 意外な接点を見ました
ミケランジェロには 先見の明があり この「知覚と創造の二重の関係」を 見ていたのだと思います 彼は 有名な言葉を残しています 「どんな石の塊にも 彫像が隠れており 彫刻家の仕事は その像を見出すことである」 ミケランジェロが気づいていたのは 我々は 知覚によって 創造しているということで 知覚自体が 想像する行為であり 創造的なものだということです
人体の中で 思考 知覚 想像を行う器官は 言うまでもなく 脳です そこで 脳科学の歩みについて 簡単に振り返りましょう 心臓や腸などとは違い 脳については 外観からは 分からないことが多いからです 少なくとも 肉眼で見た場合には 脳に注目した 昔の解剖学者たちは 脳の外部構造を見て しゃれた名前を付けました 例えば「海馬」 これは タツノオトシゴのことです しかし そのように付けられた名前は その働きについて ほとんど何も示していません
脳内で起きていることについて 本当の知見を初めて得たのは 19世紀の 偉大な スペイン人神経解剖学者 サンティアゴ・ラモン・イ・カハールだと 私は思います 彼は顕微鏡と 選択的に染める特殊な染料を使って 脳内の個々の細胞を 非常にはっきりした形で 見られるようにし そこから 形態学的理解が 進むようになりました 19世紀に 彼が描いた 神経のイメージは このようなものでした
これは 鳥の脳です このように 驚くほど 多様な細胞があります 当時は 細胞説自体が ごく新しいものでした この構造 この細胞には 樹状突起があります この突起は 非常に長く伸びうるのですが これも 当時は目新しいことでした 樹状突起は 配線のようにも見えます このことは 19世紀の一部の人には 一目瞭然だったかもしれません 電気による革命が進み 配線が普及し始めた時代だったからです しかし いろいろな面で ラモン・イ・カハールが提示した 微細解剖学的な図は ある意味 今なお越えられていません
1世紀を経た今も 我々は ラモン・イ・カハールが始めた仕事を 完成させようと試み続けています これは 我々が提携している マックス・プランク神経科学研究所による 生のデータです 彼らが行ったのは 脳の組織の小さな断片を 可視化するということです この試料全体の大きさは 1立方ミリメートルで 今 お見せしているのは そのごく一部です 左の棒の長さが 1ミクロンです ご覧の構造は ミトコンドリアで 大きさとしては バクテリアと同程度です ごく小さな組織片の 連続的断面を映しています 比較のために言うと 髪の毛の直径は 平均約100ミクロンです ご覧のものは 髪の毛の直径よりも はるかに小さいんです
このような 電子顕微鏡による 連続断面像から ニューロンの3次元像を 再構成できます ここでは ラモン・イ・カハールが したのと同じように ごく一部のニューロンだけを示しています そうしなければ あまりに密集していて わけが分からなくなってしまいます ニューロンは 互いに結合し合った ― 非常に複雑な構造をしているためです
ラモン・イ・カハールは 時代の先を行っていて 脳に対する理解は その後の数十年で ゆっくりと進んでいきました やがて ニューロンは 電気を使っていることが発見され 第二次世界大戦の頃には 仕組みの解明のため 生きたニューロンを使って 電気的な実験ができるくらいに 技術が進歩しました ほぼ同時期に コンピューターも発明されましたが これは 人間の脳をモデル化するという アイデアに基づいていました コンピュータ科学の父の1人である アラン・チューリングは これを「知的機械」と呼びました
そしてウォーレン・マカロックとウォルター・ピッツが ラモン・イ・カハールの 視覚野の図に 目を向けました 今 ここでお見せしているものです これは 目から受け取ったイメージを 処理する皮質です 2人には これが 回路図のように見えました マカロックとピッツの回路図の 細かい部分には 間違いが たくさんありますが その基本的な概念 つまり 視覚野は一連の 計算要素のように働き 段階的に情報を 受け渡していくという概念は 本質的に正しいものでした
ここで 少し時間を取って 視覚情報処理が どんなことをするのか 説明しようと思います 知覚の基本的な仕事は このような画像を見て 識別をすることです 「あれは鳥だ」と 人間の脳は この処理を簡単にやってのけますが コンピューターにとっては難問で 数年前までは ほとんど不可能でした 従来の コンピューターの構造は こういうタスクには不向きなんです
鳥のピクセル画像と 「鳥」という言葉の間にあるのは ニューラルネットワークの中の 結合しあった 一連のニューロンです 図示すると こうなります このニューラルネットワークは 視覚野内に生物学的なものとして存在し また最近では コンピューター上に モデル化できるようになりました どのように動作するか お見せしましょう
画像は ニューロンの 第1層を示しています これは目で言うと 網膜内のニューロンに相当します 情報は ニューロンの1つの層から別の層へと 次々と受け渡され ニューロン同士は 重みの異なる シナプスでつながれています このネットワークの動作は シナプス結合の 強さによって変わり それが ネットワークの 計算的特徴を決めます そうして最終的には 少数のニューロン群が反応し 「鳥」だと認識されます
ここで3つの対象物 ― 入力されたピクセル ニューラルネットワーク内のシナプス 出力である「鳥」 この3つを 「x」「w」「y」と置きましょう xは 画像中のピクセルなので 100万個くらいあり wは数十億から数兆個 ニューラルネット内の全シナプスの 結合強度を表します このネットワークからの 出力である yの個数はごくわずかです 「bird」は たった4文字ですよね? ここで 次の簡単な式が 成立すると仮定します x “×” w =y 「かける」に引用符を付けたのは この場面で実行される演算は 実際には 非常に複雑な 数学的な計算だからです
1つの方程式があって 3個の変数があります ご存じのように 3つの変数のうち 2つの値が分かれば 残りの変数の値も求められます ここでの問題は 鳥の画像から それが鳥だと推論するということでした つまり y が未知で xとwが分かっています 画像 x と ネットワーク w は 与えられています ご覧のように 比較的単純な問題です 2と3を掛け合わせれば 答えは出ます 我々が最近構築した ニューラルネットワークでは まさに これを実行しています
携帯電話上で リアルタイムで処理をしています こんなに すごいことができるのも 現在の携帯電話では 1秒当たり 数十億~数兆の命令を 実行できるからです ご覧いただいているのは 携帯電話で次々に出す 鳥の画像に対し ニューラルネットが 「これは鳥だ」と言うだけでなく 鳥の種類まで 特定しているところです この式で言うと xとwが既知で yが未知の場合です ここで 難しい部分を はしょっていました wは そもそも どうやって求めたらいいのか 脳がやっているようなことですが 人間は どうやって学ぶのでしょう?
この学習プロセス wを解くという問題は 変数が数値の 簡単な式であれば どうすればよいか分かります 6=2×w を解くには 両辺を2で割れば済みます ここで問題になるのは この演算子です 今 割り算をしましたが それは割り算が 掛け算の逆演算だからです しかし 先ほど言ったとおり 掛け算と見るのには ウソがあり 実際には とても複雑な非線形演算で 逆演算が存在しません だから 除算演算子を使わずに これを解かなければなりません でも そのやり方はそう難しくありません 代数学的な ちょっとしたワザを使うんです まず「6」を式の右辺に移します 依然として乗算を使っています そして 左辺の「0」を誤差と考えます つまり wを正しく求められれば 誤差の値は0になります wの値が 正しくない場合 誤差は0より大きくなります
誤差の値が最小になるよう 推量をします こういう処理なら コンピューターは大得意です 最初の推測値として w=0では どうでしょう? 誤差は6です w=1の場合 誤差は4 マルコ・ポーロ式鬼ごっこのような調子で 誤差を0に近づけていき そうやってwの近似値を求めます 通常 正解そのものにたどり着くことは ありませんが 数十回繰り返すと w=2.999のような 十分近い値が求まります これが 学習プロセスです
ここで改めて 思い出してください 私たちがやっていたのは 既知の xとyに対し 反復的プロセスによって 真ん中の wの値を求めるということです これは人間がものごとを学習するのと 同じやり方です 赤ちゃんのとき たくさんの絵を見せられ 「これは鳥 これは鳥じゃない」と 教わります この学習を反復することで wを解いて 神経結合を作り出すんです
xと w に対して y を求めるというのは 高速な日常的「知覚」です w を求める方法を 先ほど考えましたが これは「学習」であり ずっと難しいことです なぜなら 多くの訓練例を使って 誤差を最小化する必要が あるからです
1年ほど前 私のチームの アレックス・モードヴィンツェフは 既知の w と y に対して x の値を求めるとどうなるか 実験してみることにしました 言い換えると 鳥に対してトレーニングされた ニューラルネットが 鳥だと答えを出す 「鳥の絵」はどんなものか ということです 鳥を認識するネットワークを訓練するのと 同じ誤差最小化の手順が この場合も使えることが 分かりました 結果として出てきたのは ― 一種の鳥の絵です これは「鳥」を認識するように訓練した ニューラルネットワークによって 生成された鳥のイメージです yの値を求めるかわりに 反復によって xを求めたんです
別の面白い例を 紹介しましょう 私のグループのマイク・タイカが 作ってくれたものです マイクは「動物のパレード」と呼んでました ウィリアム・ケントリッジの作品を 思わせます ケントリッジは スケッチを描いては消し また 描いては消しして アニメーションを作り出します この場合 マイクは 動物種の集合の中で yの値を変えていて 動物の種類を識別するよう 設計された ネットワークを使っています エッシャーのだまし絵のように 動物が別の動物へと変形していきます
次は マイクとアレックスが 協力して作ったもので y を二次元空間に 収めようと試みていて ネットワークが認識するもの 全てを含む空間の 地図を作っています この画像合成・画像生成を yの値を変化させながら 画面全体にわたって行うことで このような地図が できあがります ネットワークが認識するものすべての 視覚地図です いろんな動物が現れます あそこにアルマジロがいますね
他のネットワークでも 同様の処理が実行できます これは 人物の顔を見分ける ― 顔認識のために設計された ネットワークです ここで yは「私」です 私の顔をパラメータにして このネットワークを使い xの値を求めると かなり ぶっ飛んだ 画像が作られます キュービズムというか シュールというか サイケな感じの 私の絵です 複数の視点を 1つにまとめています このように 複数の視点が 1つになっている理由は このネットワークが あいまいさを除去する 設計になっているためです 人の顔の見え方は 見る角度によって変わり 光の当たり方によっても 変わります だから このような再構成を行う場合 ガイドとなる画像や 統計値がないと 視点に関して 混乱が生じるんです あいまいさがあるためです ここでは 私の顔を再構成する 最適化プロセスで アレックスの顔の映像を ガイドとして使っています 完璧とは言えず この最適化プロセスを 改善するために やることは まだまだありますが とりあえず 顔をガイドとして使うことで 統一感のあるイメージが できるようになります
別に 真っ白なキャンバスや ホワイトノイズから 始める必要はありません xを求めるという場合 xとして 何か別の画像から 出発してもいいんです それを説明するデモを お見せしましょう これは 人工物であれ 動物であれ あらゆるものを分類するよう 設計されたネットワークです この雲の写真からスタートします これを最適化すると このネットワークは 「雲の中に何が見えるか」を探します 画面をじっと見ているうちに 雲の中に いろんなものが 見えてきます これに顔認識ネットワークを使うと 幻覚っぽい映像になります ちょっと頭がおかしくなりそうな
(笑)
マイクはまた この雲の画像で 別の実験もしています 幻視とズームを繰り返していくと こんな感じに フーガのような 自由連想のような映像を このネットワークから 作り出せます ネットワークが 自分の尻尾を食べています それそれのイメージが 次のイメージのベースになっています 「次に 何が見える? 次に 何が見える? 次に 何が見える?」という風に
ちなみに このデモを 最初に披露したのは シアトルで開かれた 「高等教育」と題した講演会で マリファナが合法化された 直後のことでした
(笑)
最後にまとめとして この技術は お見せしたようなものに 限定されないことを指摘しておきます 今回は視覚的にちょっと面白い例を 紹介しましたが 別に 視覚のテクノロジー というわけではないんです 我々の協力者でアーティストの ロス・グッドウィンによる実験ですが 彼がカメラで写真を撮ると その写真の中身を元に 背負っているコンピューターが ニューラルネットワークで 詩を書きます 詩のニューラルネットワークは 20世紀の詩の 膨大なコーパスで トレーニングされています そうやって書かれた詩は どうして そう悪くないと思います
(笑)
まとめになりますが ミケランジェロは正しかったと つくづく思います 知覚と創造性とは 密接に結びついているんです 先ほどお見せした ニューラルネットワークは 世の中の さまざまなものを区別するよう 学習させたものですが 処理を逆転させて 新しいものを作り出すこともできます そこで気づかされるのは 石の塊の中に 彫刻を見ることができるのは ミケランジェロだけではないということ どんな生物であれ どんな存在 地球外生命体だろうと 知覚を行う能力のあるものは 創造もできるということです どちらも同じメカニズムで できることだからです
そして 知覚と創造は 決して 人間に限られたものではありません 私たちは そのようなことができる コンピューターモデルを作り始めています 驚くことではありません 脳もまた計算機械だからです
最後に コンピューティングは 知的機械の設計から始まり 機械はいかに知的になれるか という発想から モデル化されましたが 今や我々は 昔の先駆者が 夢見た世界を ようやく実現させつつあります チューリングやフォン・ノイマンの夢 マカロックやピッツの夢を コンピューティングは 会計処理だとか キャンディークラッシュで 遊ぶだけのものではありません コンピューターはそもそも 人間の脳をモデルとしたものでしたが それは 人間の知性を よりよく理解するためにも 人間の知性を拡張するためにも 使えるのです
ありがとうございました
(拍手)
品詞分類
- 主語
- 動詞
- 助動詞
- 準動詞
- 関係詞等
TED 日本語
TED Talks
関連動画
10分で分かる量子コンピュータショヒーニ・ゴーシュ
2019.02.01あなたの人生にサウンドトラックを付けてくれるAIピエール・バロー
2018.10.01実在の人物の偽映像の作り方と、その見分け方スパソーン・スワジャナコーン
おすすめ 12018.07.25AIはいかに人の記憶、仕事、社会生活を改善するかトム・グルーバー
2017.08.21コンピューターはいかに物体を即座に認識できるようになったのかジョセフ・レドモン
2017.08.18知性を持つ機械を恐れるな、協働せよガルリ・カスパロフ
2017.06.20人工知能が人間を超えるのを怖れることはないグラディ・ブーチ
2017.03.13直感を持った人工知能が生み出すすごい発明モーリス・コンティ
2017.02.28表計算ソフト誕生の話ダン・ブリックリン
2017.02.01機械知能は人間の道徳性をより重要なものにするゼイナップ・トゥフェックチー
2016.11.11Linuxの背後にある精神リーナス・トーバルズ
2016.05.03ホログラム時代の未来にあるものアレックス・キップマン
2016.04.18拡張現実ヘッドセットを通して未来を覗くメロン・グリベッツ
2016.04.11形状変化テクノロジーが仕事を革新するショーン・フォルマー
2016.03.01インターネットをスクリーンなしで見るとトム・アグロ―
2015.11.13顔を見るだけで感情がわかるアプリラナ・エル・カリウビ
2015.06.15
洋楽 おすすめ
RECOMMENDS
洋楽歌詞
ステイザ・キッド・ラロイ、ジャスティン・ビーバー
洋楽最新ヒット2021.08.20スピーチレス~心の声ナオミ・スコット
洋楽最新ヒット2019.05.23シェイプ・オブ・ユーエド・シーラン
洋楽人気動画2017.01.30フェイデッドアラン・ウォーカー
洋楽人気動画2015.12.03ウェイティング・フォー・ラヴアヴィーチー
洋楽人気動画2015.06.26シー・ユー・アゲインウィズ・カリファ
洋楽人気動画2015.04.06シュガーマルーン5
洋楽人気動画2015.01.14シェイク・イット・オフテイラー・スウィフト
ポップス2014.08.18オール・アバウト・ザット・ベースメーガン・トレイナー
ポップス2014.06.11ストーリー・オブ・マイ・ライフワン・ダイレクション
洋楽人気動画2013.11.03コール・ミー・メイビーカーリー・レイ・ジェプセン
洋楽人気動画2012.03.01美しき生命コールドプレイ
洋楽人気動画2008.08.04バッド・デイ~ついてない日の応援歌ダニエル・パウター
洋楽人気動画2008.05.14サウザンド・マイルズヴァネッサ・カールトン
洋楽人気動画2008.02.19イッツ・マイ・ライフボン・ジョヴィ
ロック2007.10.11アイ・ウォント・イット・ザット・ウェイバックストリート・ボーイズ
洋楽人気動画2007.09.14マイ・ハート・ウィル・ゴー・オンセリーヌ・ディオン
洋楽人気動画2007.07.12ヒーローマライア・キャリー
洋楽人気動画2007.03.21オールウェイズ・ラヴ・ユーホイットニー・ヒューストン
洋楽人気動画2007.02.19オネスティビリー・ジョエル
洋楽人気動画2005.09.16